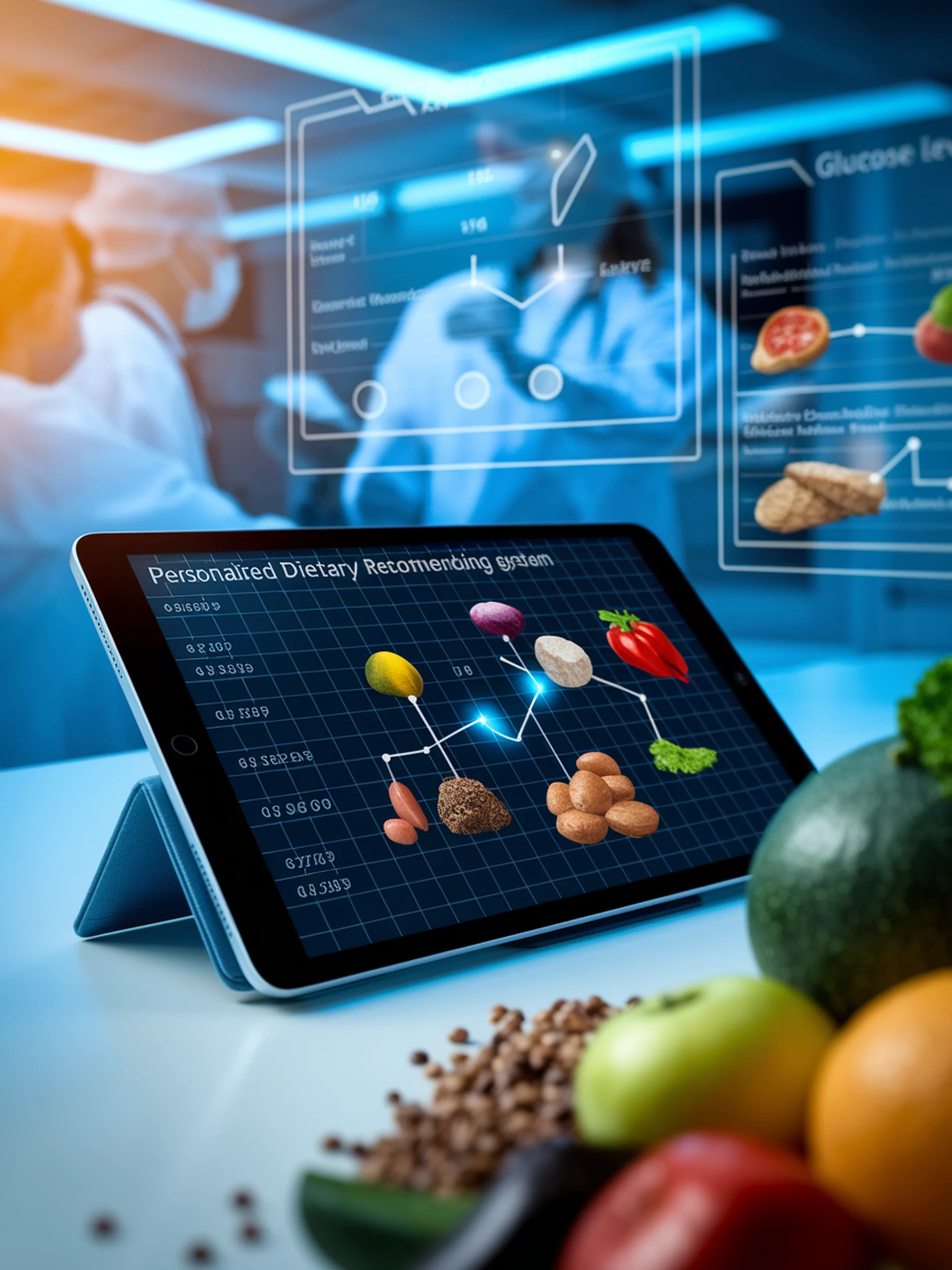
Personalizing LLM Reasoning for Healthcare
Enhancing AI dietary recommendations through causal graphs
This research introduces a framework that equips LLMs with personalized reasoning capabilities for tailored dietary recommendations based on individual health data.
- Combines personal glucose response data with causal reasoning graphs
- Outperforms standard LLMs in providing contextual dietary advice
- Enables precise, individual-specific recommendations instead of generic advice
- Creates a foundation for trustworthy AI-driven personalized healthcare
Why it matters: This approach bridges a critical gap in healthcare AI, moving beyond one-size-fits-all recommendations to truly personalized medical guidance that considers individual biological responses to different foods.
Personalized Causal Graph Reasoning for LLMs: A Case Study on Dietary Recommendations