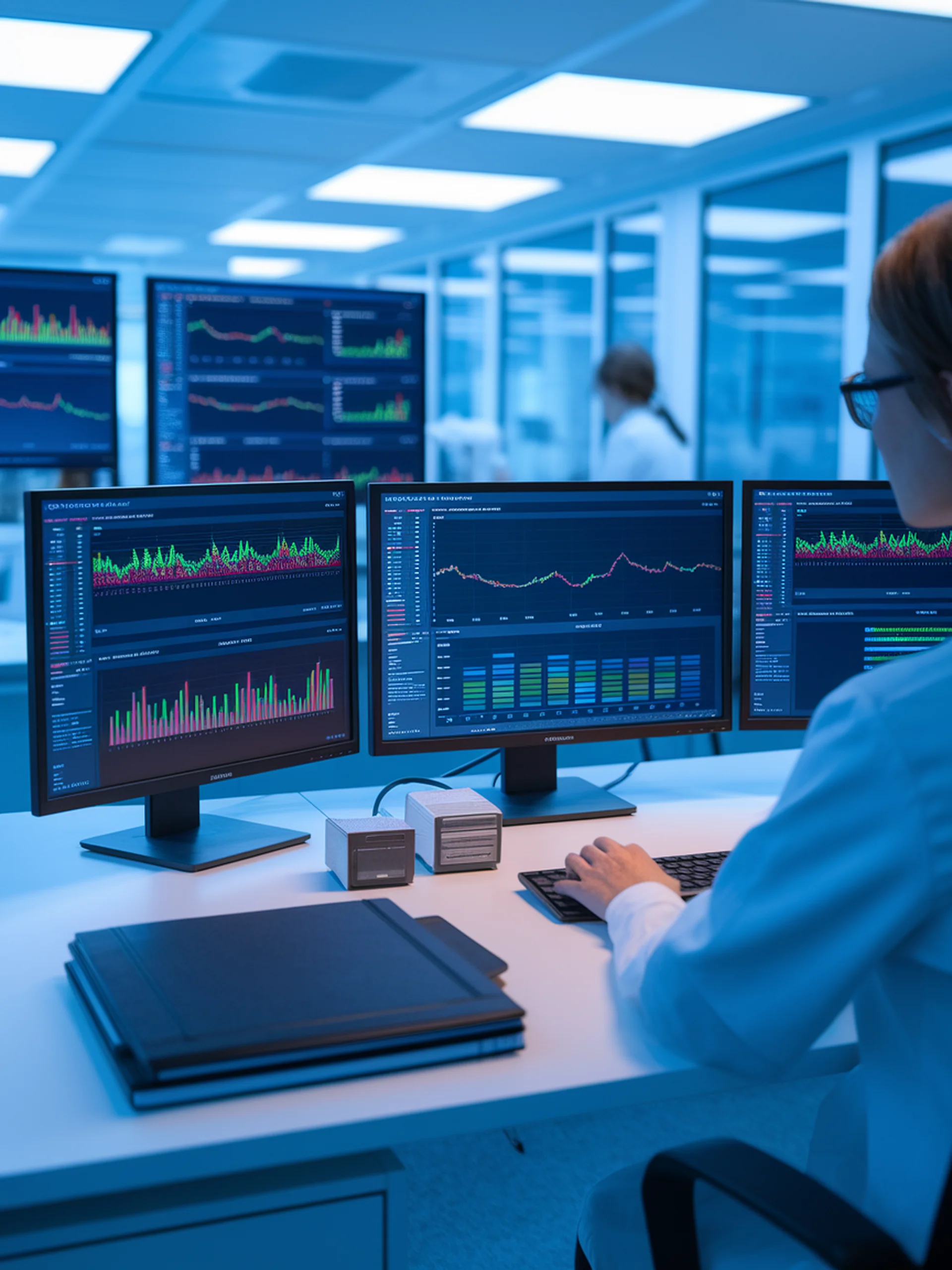
Harnessing LLM Ensembles for Medical Text Analysis
Privacy-Preserving Automated Labeling of Sensitive Free-Text Data
This research introduces an ensemble LLM approach for efficiently labeling and rating sensitive free-text responses in psychological studies, particularly for eating disorders.
- Combines multiple locally-run open-source LLMs to analyze free-text data while maintaining privacy
- Achieves near human-expert level accuracy through meta-prompting and model ensembling
- Reduces the time-consuming process of manual coding by trained specialists
- Demonstrates effectiveness on sensitive medical text data from eating disorder patients
This innovation addresses a critical challenge in medical research by allowing researchers to quickly extract structured insights from unstructured patient text data while maintaining privacy compliance—potentially accelerating discovery in mental health research.
Ensemble of Large Language Models for Curated Labeling and Rating of Free-text Data