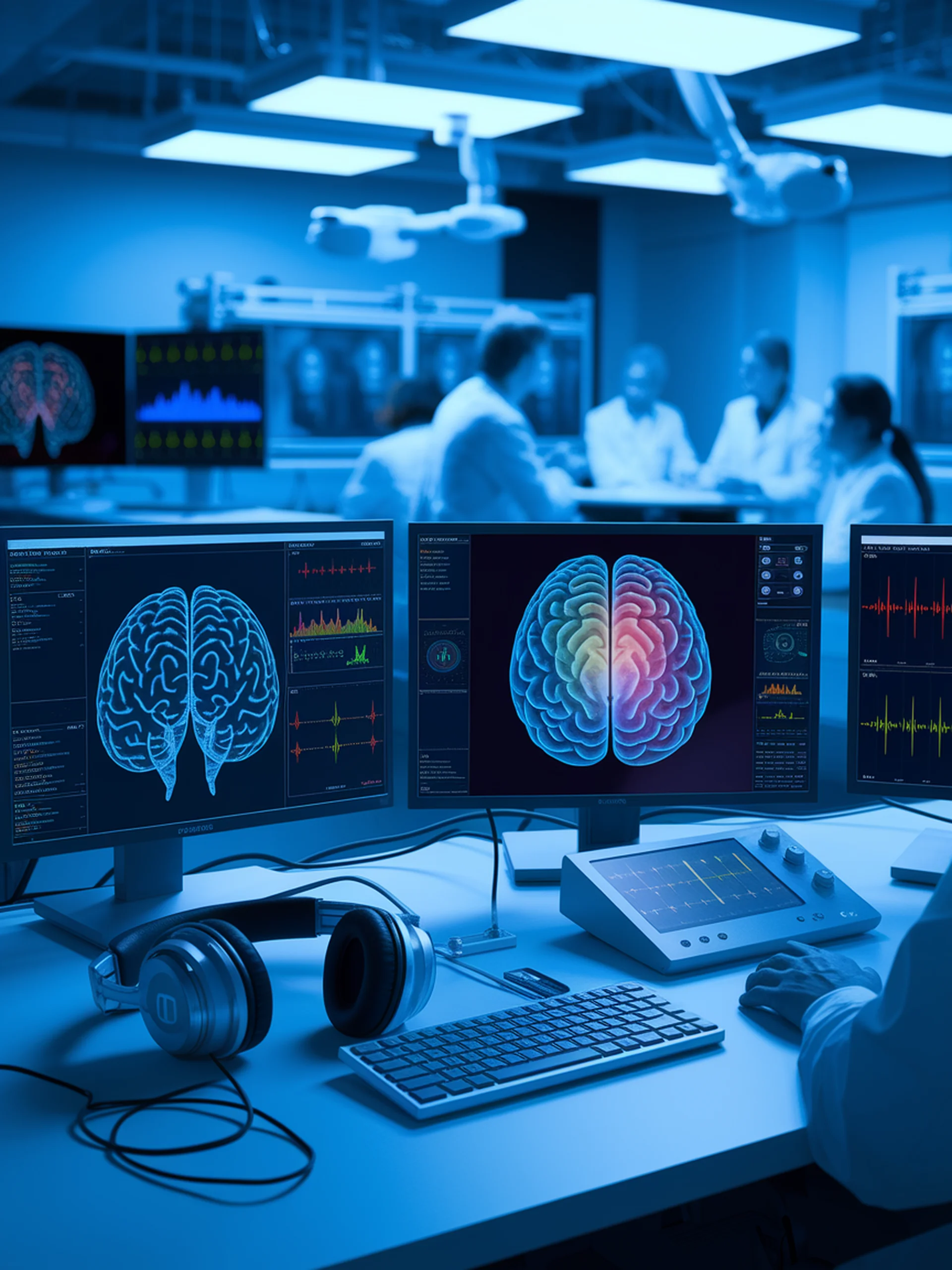
Multimodal Depression Detection
Fusing Text and Audio for More Accurate Mental Health Assessment
A novel teacher-student architecture that combines text and audio data to significantly improve depression classification accuracy.
- Multi-head attention mechanisms enable more effective feature fusion
- Weighted multimodal transfer learning optimizes integration of different data types
- Student fusion model leverages guidance from specialized text and audio teacher models
- DAIC-WOZ dataset validation demonstrates superior performance over traditional approaches
Medical Impact: This multimodal approach offers mental health professionals more reliable diagnostic tools, potentially enabling earlier intervention and improving treatment outcomes for depression patients.
Multimodal Magic: Elevating Depression Detection with a Fusion of Text and Audio Intelligence