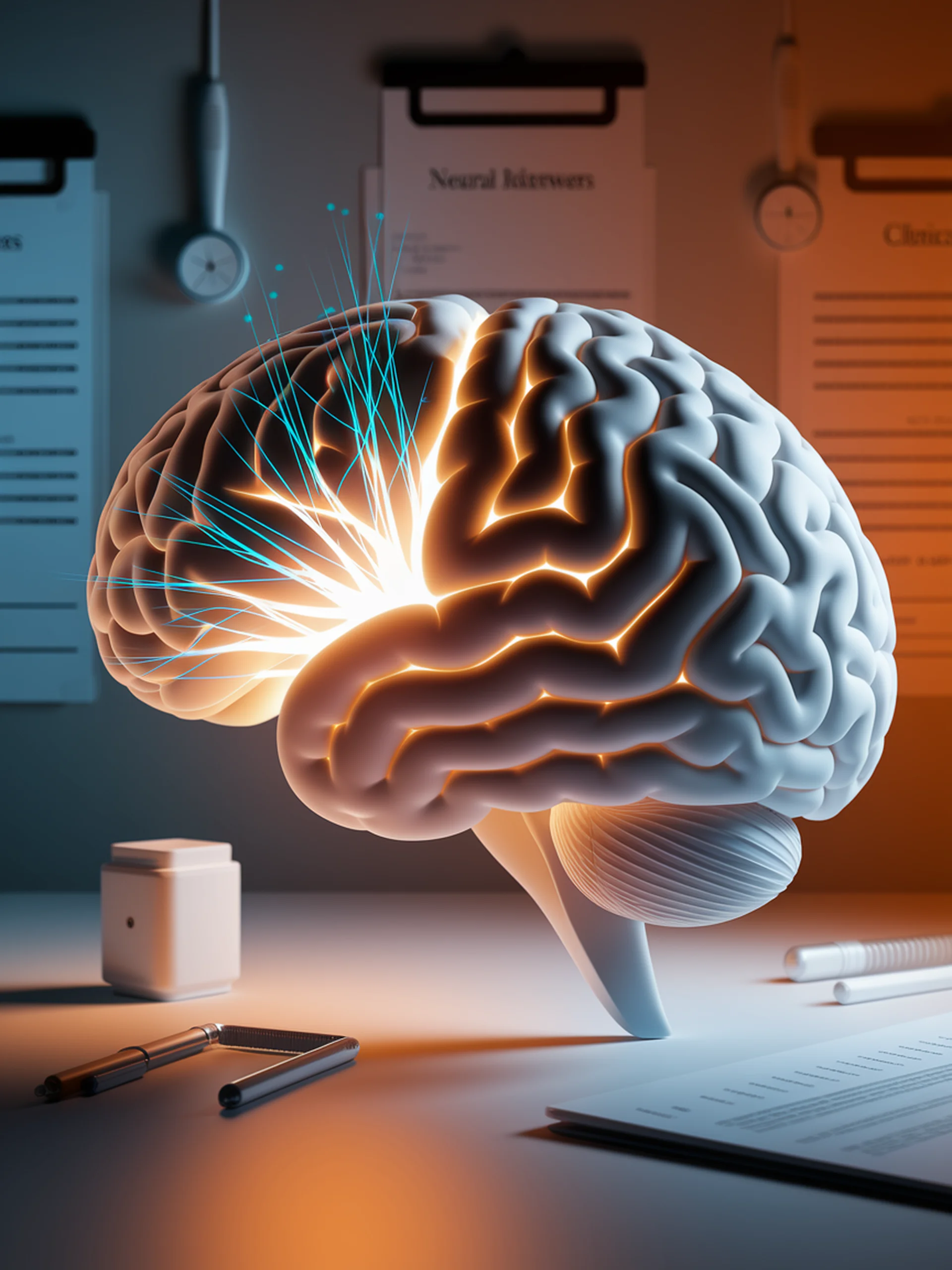
Small Models, Big Impact in Mental Health
Why smaller neural language models outperform LLMs in detecting thought disorders
This research challenges the "bigger is better" paradigm by demonstrating that smaller neural language models can more effectively detect thought disorders in schizophrenia patients than large language models.
- Superior performance: Small neural language models outperform larger models in detecting disorganized thinking patterns
- Clinical practicality: Smaller models address privacy concerns, reduce computational/financial costs, and offer greater transparency
- Practical applications: Potential for more accessible and efficient mental health screening tools in clinical settings
- Paradigm shift: Demonstrates that model size isn't always correlated with effectiveness for specialized tasks
For medical professionals, this research offers a path toward more accessible, efficient, and privacy-preserving tools for mental health assessment that could be deployed in resource-limited settings.