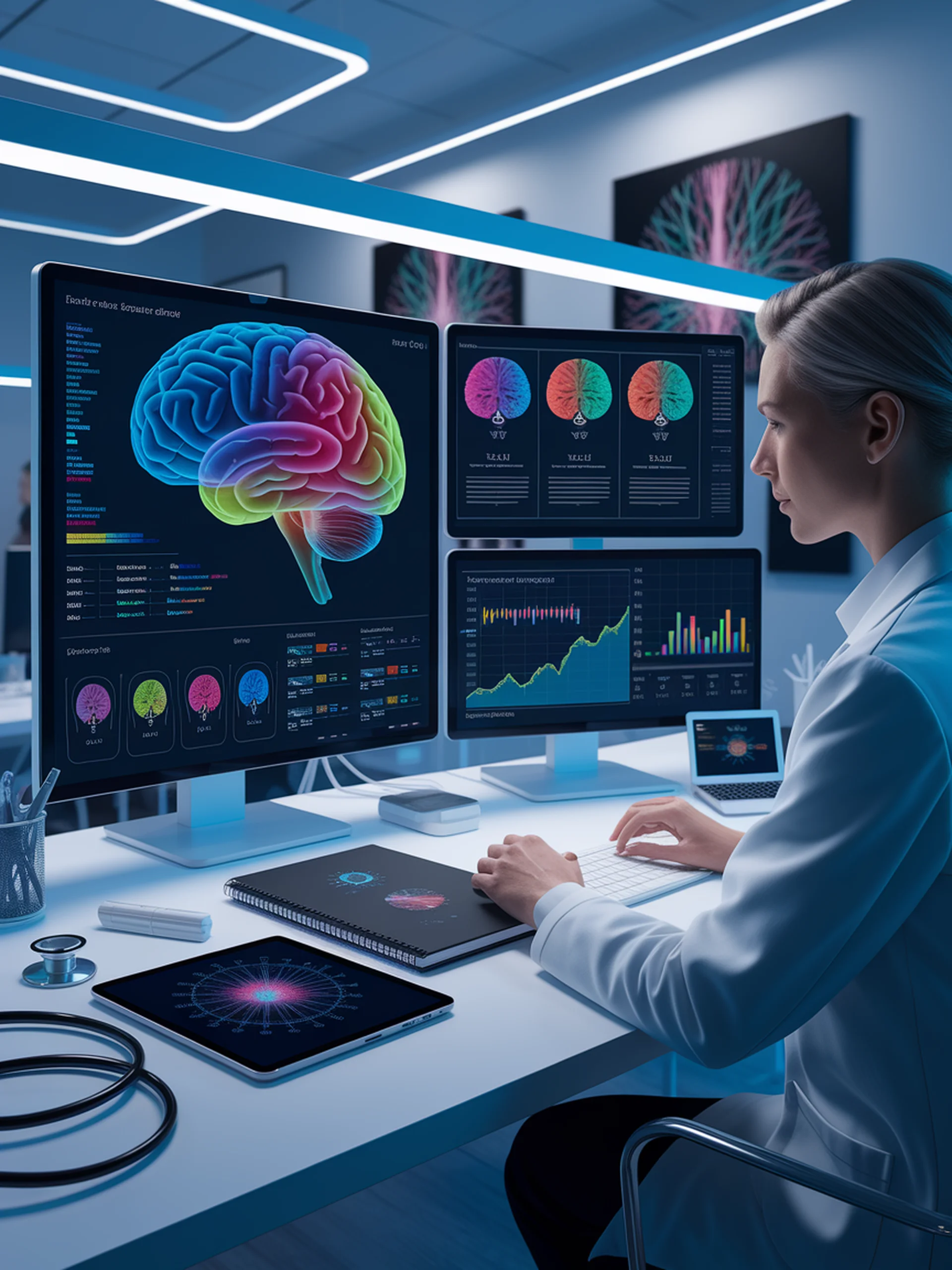
Optimizing LLMs for Mental Health Analysis
Fine-tuning outperforms prompt engineering and RAG for mental health text analysis
This research systematically compares three approaches for mental health text analysis using LLaMA 3, evaluating their effectiveness across emotion classification and mental health condition detection tasks.
- Fine-tuning achieved the highest accuracy (91% for emotion classification, 80% for mental health conditions)
- Prompt engineering offers a balance between performance and resource requirements
- RAG demonstrated potential but didn't match fine-tuning performance
- Results highlight the trade-off between computational resources and accuracy
This research provides crucial guidance for developing more effective mental health support systems and clinical tools, enabling improved detection of mental health conditions through text analysis.