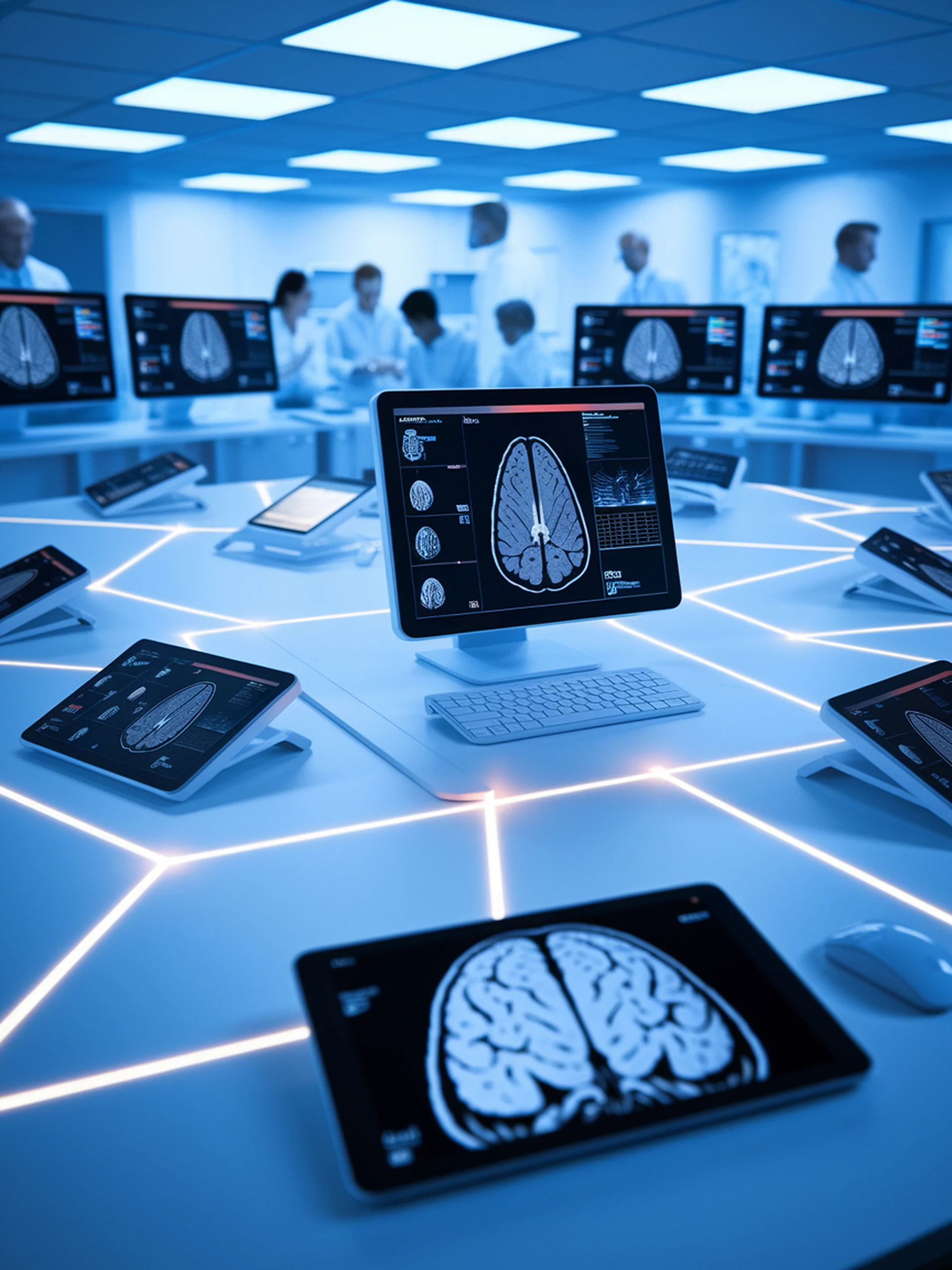
Smart Federated Learning for Vision-Language Models
Optimizing Model Fine-tuning on Resource-constrained Devices
F³OCUS introduces a novel approach for efficient federated fine-tuning of large vision-language models across distributed medical devices with limited resources.
- Implements layer-specific importance scoring to identify the most critical model layers for fine-tuning on each client
- Utilizes inter-client layer diversity to encourage different devices to focus on complementary parts of the model
- Employs multi-objective meta-heuristics to optimize the selection strategy across the federation
- Demonstrates significant performance gains on medical image analysis tasks while reducing computational burden
This research enables more effective deployment of advanced vision-language models in healthcare settings where data privacy is critical and computing resources are limited.