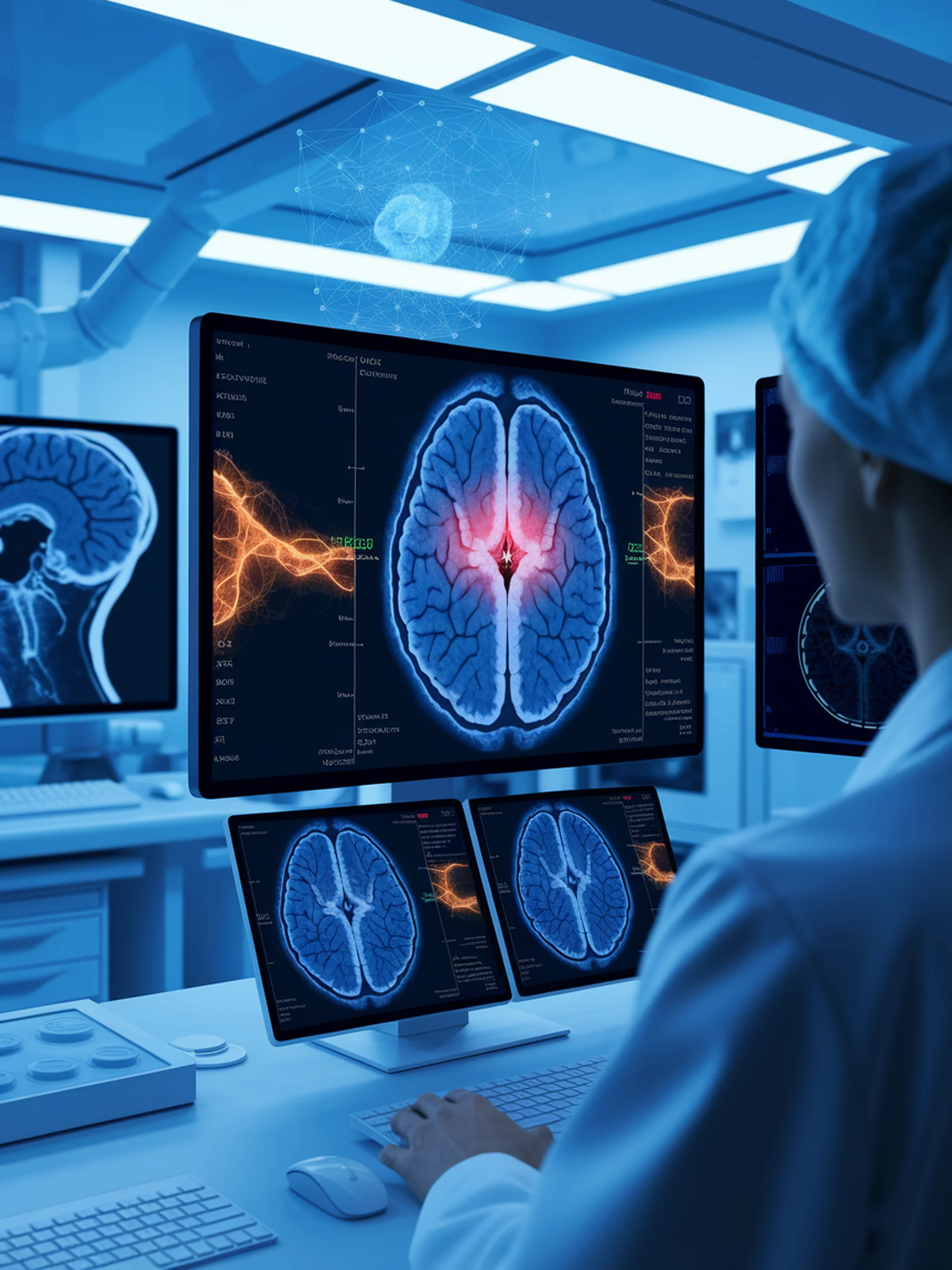
AI-Powered Tumor Detection
Foundation Models for Annotation-Free Brain Tumor Segmentation
This research introduces CLISC, a novel approach combining CLIP and SAM foundation models to accurately segment brain tumors without human annotations.
- Leverages vision-language models to identify tumors in medical images autonomously
- Achieves superior performance by enhancing Class Activation Maps (CAM) techniques
- Eliminates costly and time-consuming manual annotation requirements
- Demonstrates potential for wider application across medical imaging diagnostics
Why It Matters: This breakthrough reduces diagnostic bottlenecks in healthcare by enabling automatic tumor identification without expert annotations, potentially accelerating treatment decisions and improving patient outcomes.
CLISC: Bridging clip and sam by enhanced cam for unsupervised brain tumor segmentation