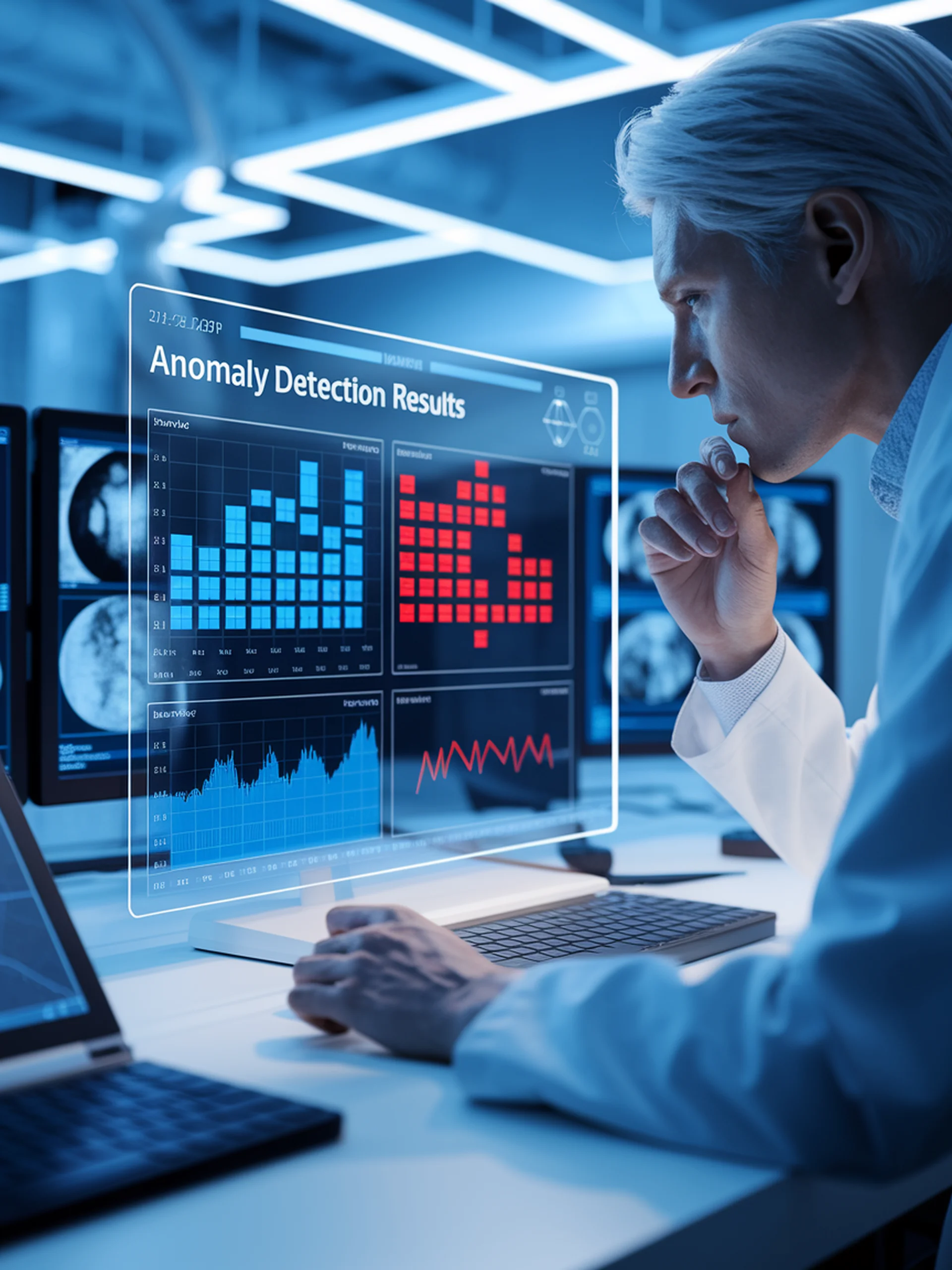
Personalizing Anomaly Detection
Improving few-shot anomaly detection through feature personalization
This research introduces One-to-Normal (O2N), a novel approach that significantly enhances few-shot anomaly detection by personalizing anomaly features to normal samples.
- Transforms anomaly features to better align with normal sample distributions
- Outperforms existing methods by focusing on feature personalization rather than direct comparison
- Demonstrates effectiveness across security, factory, medical, and engineering applications
- Requires only a few normal samples for effective anomaly detection
For security applications, this approach enables more accurate threat detection with minimal examples, improving system reliability while reducing false positives in security monitoring scenarios.
One-to-Normal: Anomaly Personalization for Few-shot Anomaly Detection