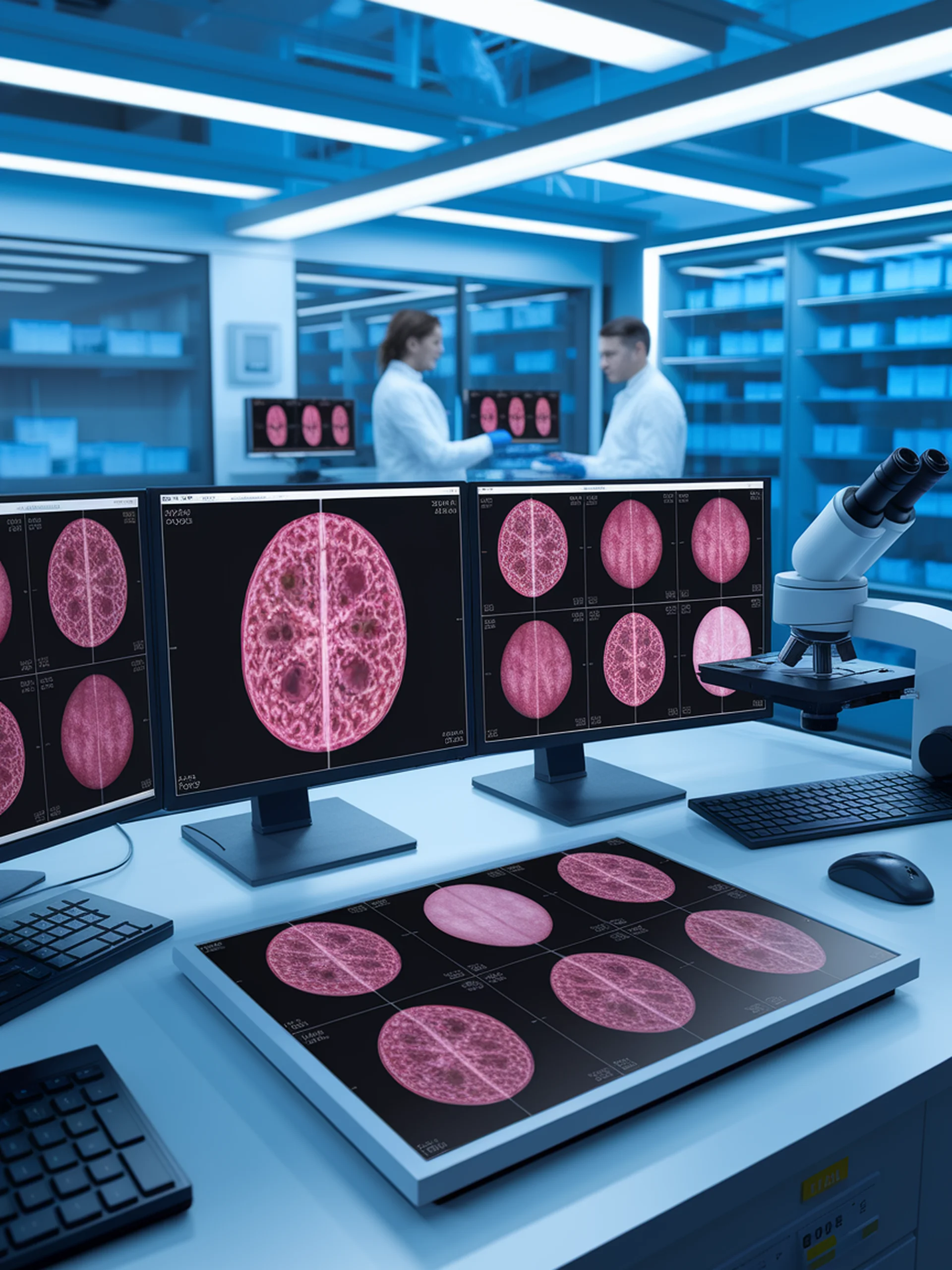
Multi-Granular Prompting for Medical Imaging
Advancing Few-Shot Pathology Classification with Vision-Language Models
This research enhances gigapixel pathology image analysis through innovative multi-granular prompt learning, enabling accurate classification with minimal labeled examples.
- Extends Prov-GigaPath foundation model (pre-trained on 1.3B pathology images) into a vision-language model
- Implements MGPATH: a novel approach using multi-scale contextual cues for improved diagnostic accuracy
- Achieves superior performance in few-shot learning scenarios across lung, kidney, and breast pathology
- Bridges the gap between massive unlabeled medical datasets and limited annotated cases
This advancement matters for healthcare by enabling more accurate pathology diagnostics with fewer labeled samples, potentially accelerating specialized disease identification and treatment planning in clinical settings.
MGPATH: Vision-Language Model with Multi-Granular Prompt Learning for Few-Shot WSI Classification