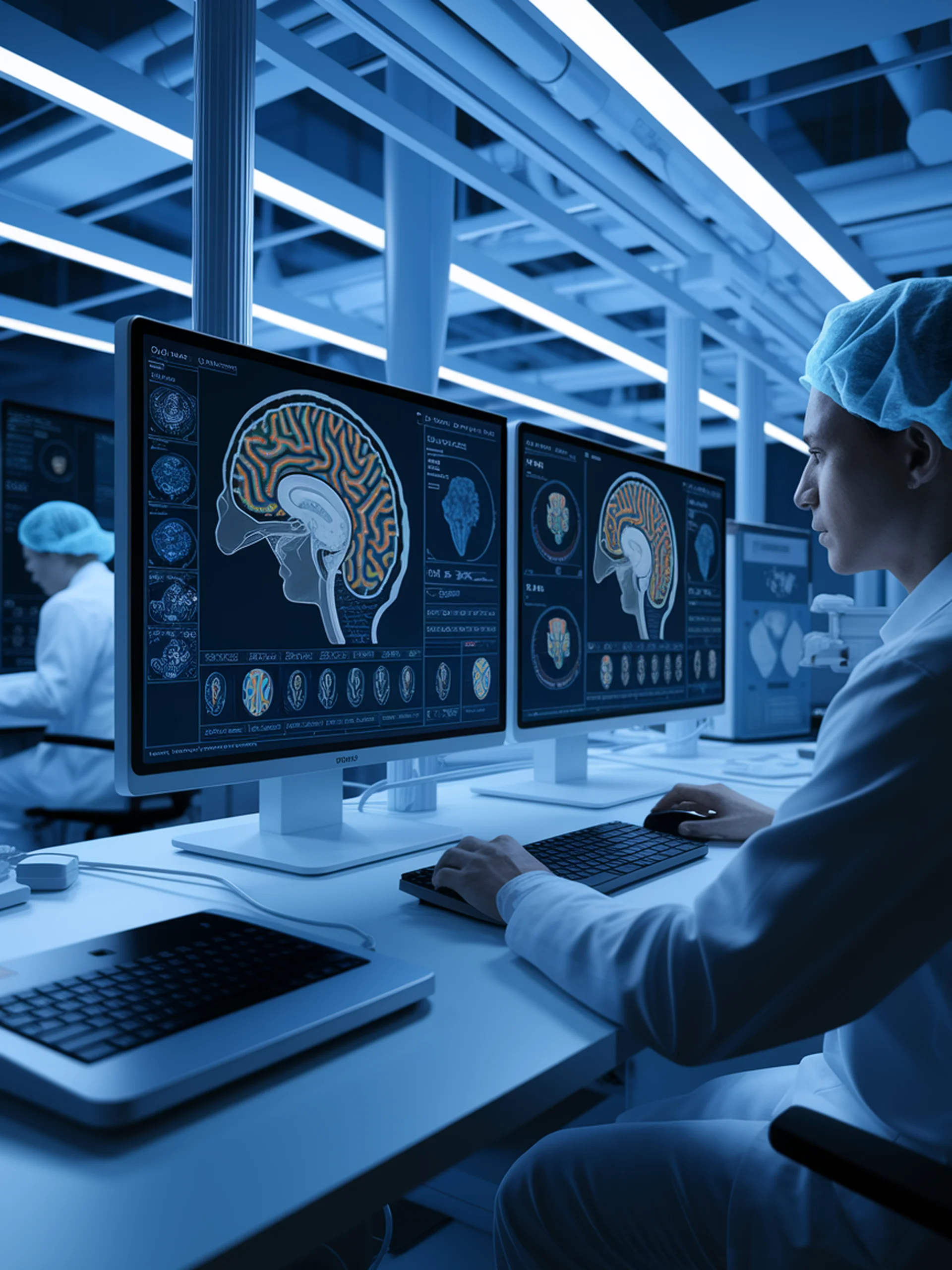
Enhancing Visual Intelligence Through Self-Learning
Improving multimodal AI reasoning and explainability with synthetic data
This research introduces a visual rejection sampling framework that boosts large multimodal models' ability to perform fine-grained visual reasoning and provide justifiable explanations.
- Addresses critical limitations in current vision-language models
- Leverages self-synthesized data to improve cognitive capabilities
- Enhances domain-specific visual understanding and reasoning
- Improves explainability of AI decisions through better justifications
Medical Impact: This approach is particularly valuable for medical applications where precise visual analysis and transparent decision-making are crucial for diagnostics, treatment planning, and clinical decision support. The improved explainability creates more trustworthy AI systems for healthcare professionals.