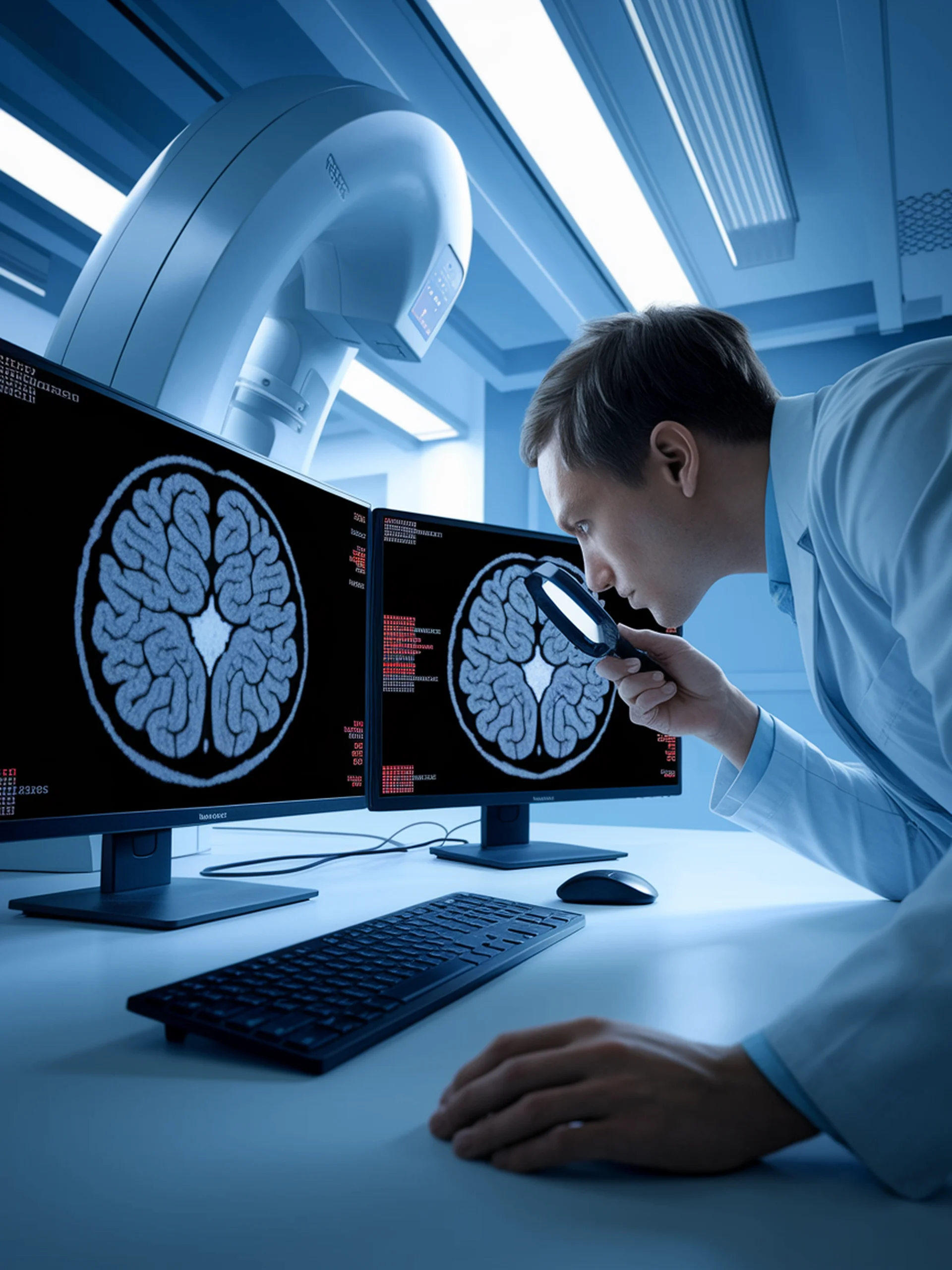
The Challenge of Small Visual Details
Understanding and enhancing MLLMs' perception capabilities
This study reveals how Multimodal Large Language Models (MLLMs) struggle to perceive small visual details, presenting both limitations and opportunities for improvement.
- Performance degradation occurs significantly when visual subjects are small
- Training-free zooming approach effectively improves MLLMs' perception abilities
- Cross-model evaluation shows this limitation exists across various MLLM architectures
- Security implications highlight risks in critical applications where small detail detection matters
For security professionals, these findings underscore the importance of robust testing when deploying MLLMs in sensitive domains like surveillance, medical diagnostics, or document verification where small visual details may be crucial.
MLLMs Know Where to Look: Training-free Perception of Small Visual Details with Multimodal LLMs