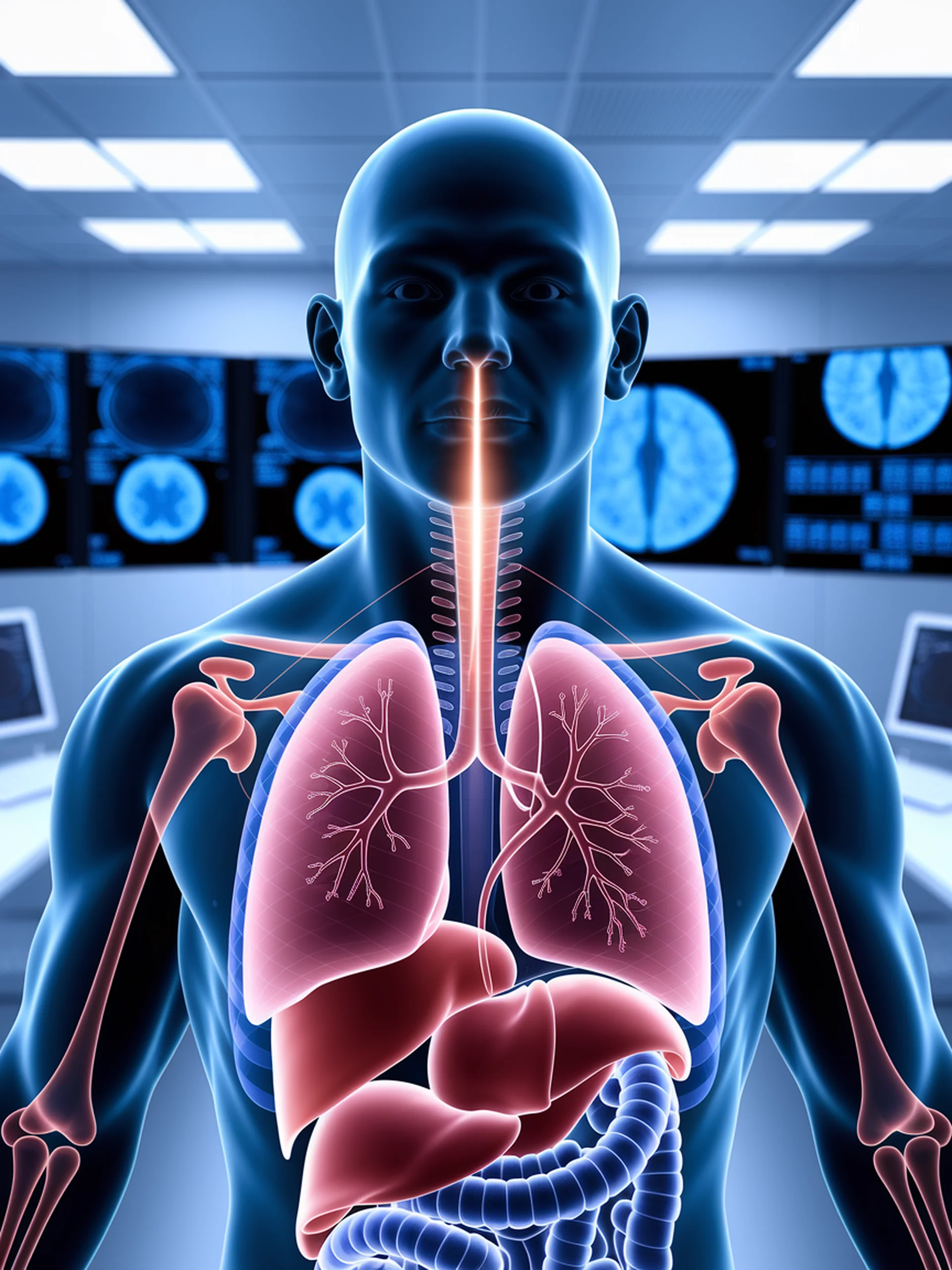
Universal Text-Driven CT Segmentation
Bridging the gap between natural language and medical image analysis
This research introduces a novel text-driven framework that enables segmentation of any anatomical structure in CT images based on natural language descriptions.
- Developed a foundation model that processes both textual descriptions and CT volumes simultaneously
- Achieved superior performance compared to specialized models, especially with limited training data
- Successfully generalized to unseen anatomical structures without requiring additional training
- Demonstrated effectiveness for both organs and tumors across diverse clinical datasets
This innovation matters for medicine by eliminating the need for specialized models for each anatomical structure, reducing annotation requirements, and enabling radiologists to segment structures using simple text descriptions.