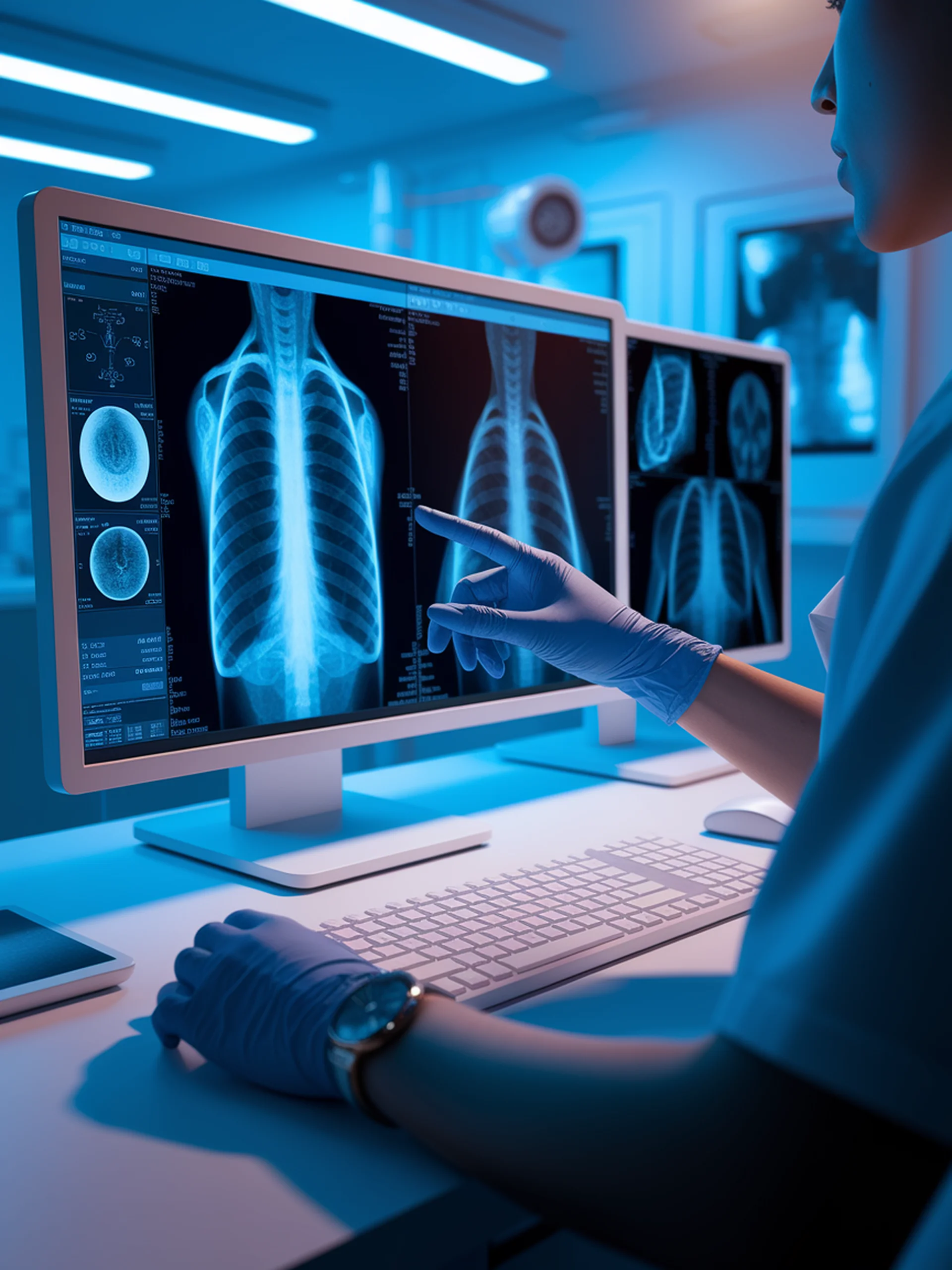
Revolutionizing Medical Imaging with Zero-Shot Learning
How CLIP Integration Improves Chest X-Ray Analysis Without Labeled Data
This research introduces MoCoCLIP, a novel approach that combines contrastive language-image pre-training with advanced techniques to enable effective diagnosis of thoracic diseases without requiring large labeled datasets.
- Addresses the critical challenge of limited labeled data in medical imaging
- Leverages zero-shot learning to detect pulmonary pathologies in chest X-rays
- Demonstrates improved performance when evaluated on NIH ChestXray14 and CheXpert datasets
- Offers a practical solution to assist radiologists with the growing volume of medical imaging data
This innovation matters for healthcare because it reduces dependency on expert annotations, potentially accelerating diagnostic workflows and improving accessibility of AI-assisted medical imaging analysis in resource-constrained settings.