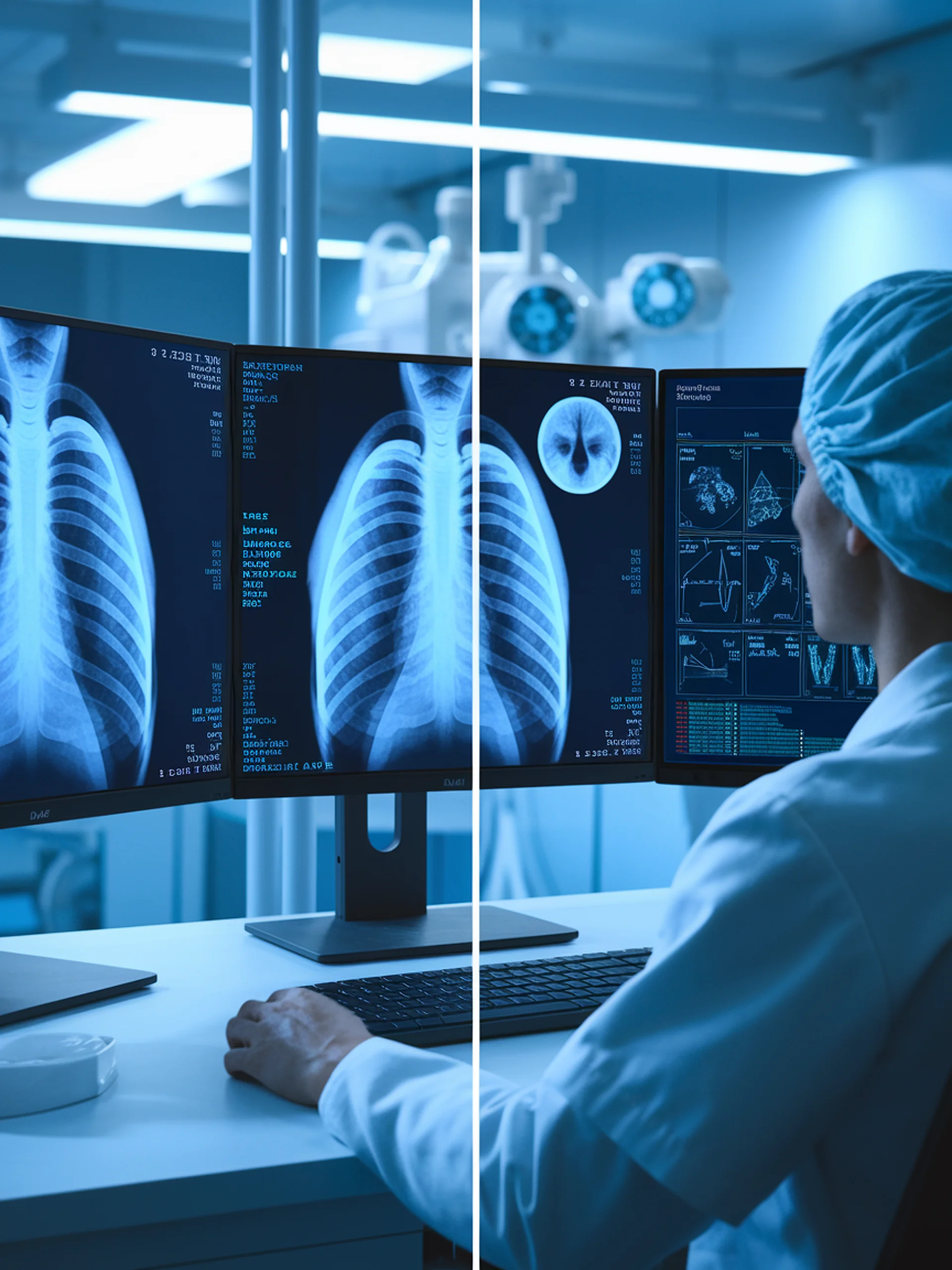
CLIP Adaptation for Radiology Reports
Leveraging pre-trained vision-language models for medical imaging
UniCrossAdapter effectively transfers knowledge from general-purpose vision-language models to specialized medical applications, addressing data scarcity in radiology.
- Introduces a novel cross-attention adapter architecture that enables efficient fine-tuning of CLIP for medical imaging
- Achieves state-of-the-art performance in generating accurate radiology reports from medical images
- Demonstrates how transfer learning can bridge the gap between general visual understanding and specialized medical domains
- Significantly reduces the amount of labeled medical data needed for effective model training
This research matters because it helps automate and improve the efficiency of radiology reporting workflows, potentially reducing physician burnout while maintaining diagnostic accuracy in clinical settings.
UniCrossAdapter: Multimodal Adaptation of CLIP for Radiology Report Generation