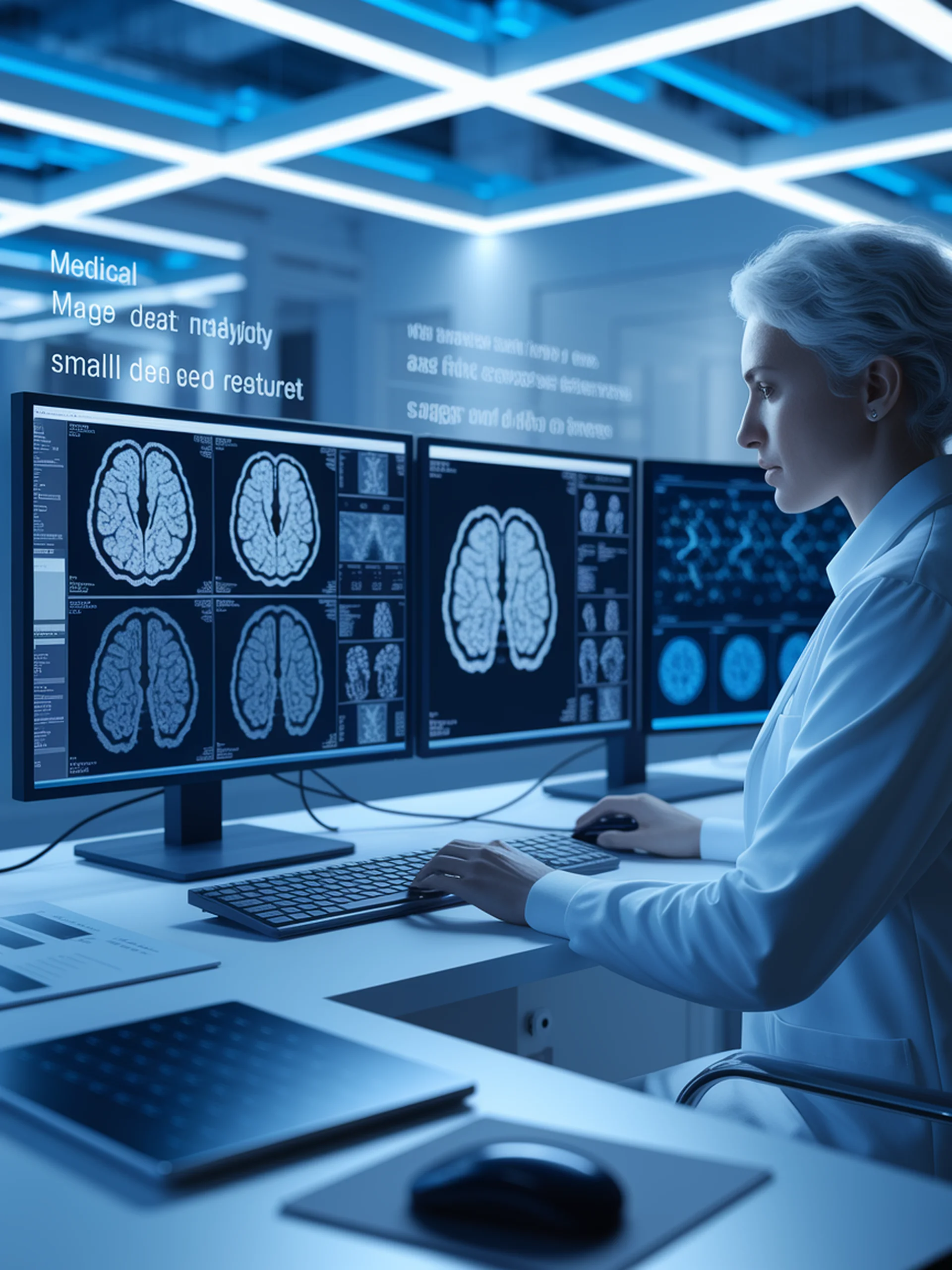
Bridging the Small Data Gap in Vision AI
Addressing the overlooked sweet spot of 100-1000 labeled samples
This research examines the critical yet understudied small-data regime in computer vision, where applications require hundreds to thousands of expert-annotated samples.
- Current AI research focuses heavily on zero/few-shot learning, neglecting this practical middle ground
- Small-data applications are crucial for specialized fields requiring expert annotations
- The study uses Natural World Task to evaluate performance in this regime
- Traditional vision models may have significant performance gaps in these real-world scenarios
For medical diagnostics, this research highlights how current evaluation practices fail to address the reality of clinical image analysis, where collecting thousands of expert-annotated medical images is costly but essential for reliable AI systems.
Mind the Gap: Evaluating Vision Systems in Small Data Applications