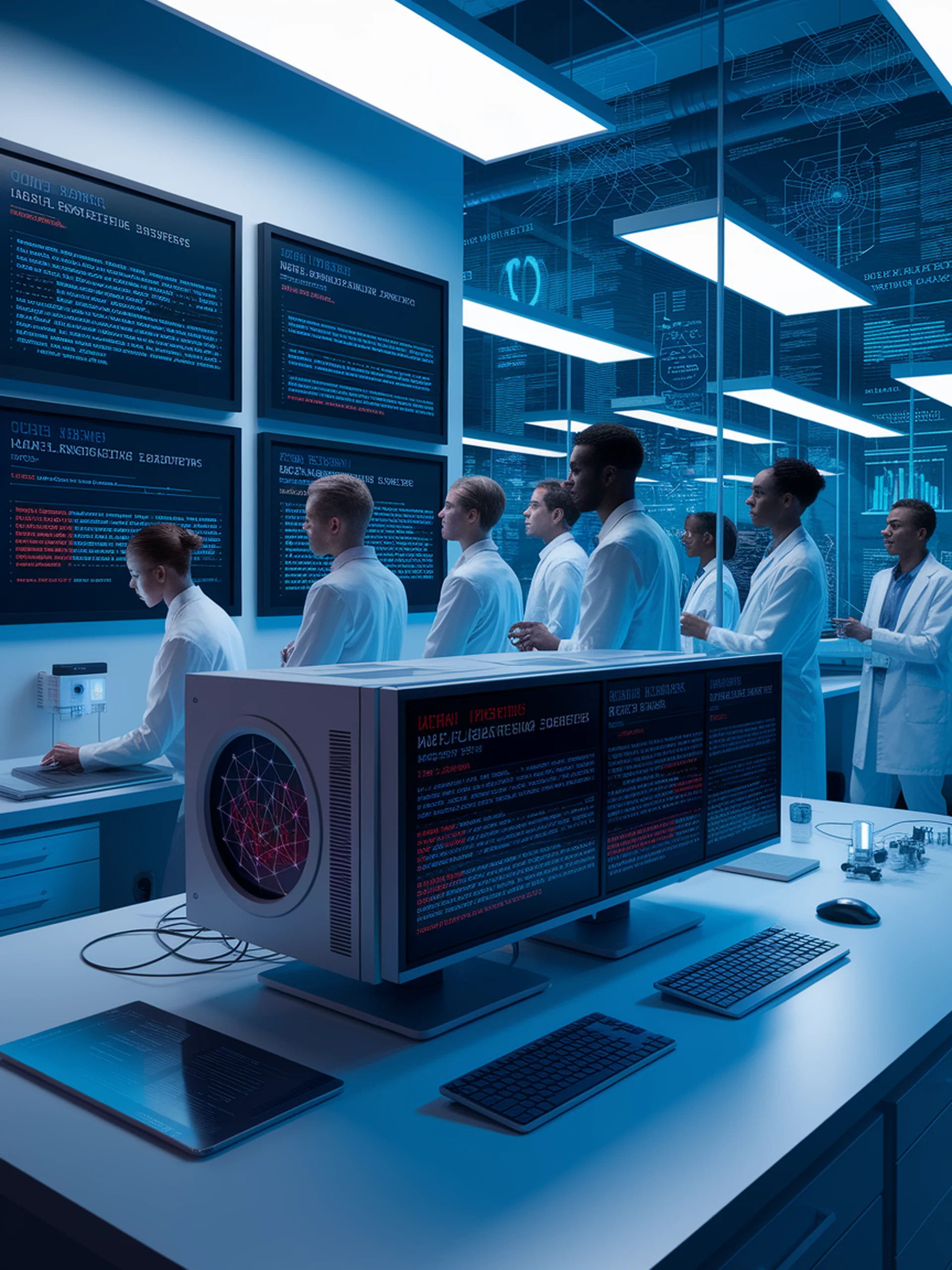
Detecting AI-Generated Academic Content
Evaluating the generalization and adaptation of LLM detection systems
This research evaluates how effectively machine-generated text (MGT) detection systems can identify AI-written academic content across different contexts and models.
Key findings:
- Detection systems show limited cross-domain generalization when applied to different subject areas
- Detectors trained on one LLM often struggle to identify content from newer or different models
- Adaptation techniques can significantly improve detection performance when systems are exposed to small samples from target domains
- Results highlight the need for continuously updated detection systems as LLMs evolve
For security professionals, this research provides critical insights into the limitations of current AI content detection methods and offers practical approaches to improve systems protecting academic integrity.
On the Generalization and Adaptation Ability of Machine-Generated Text Detectors in Academic Writing