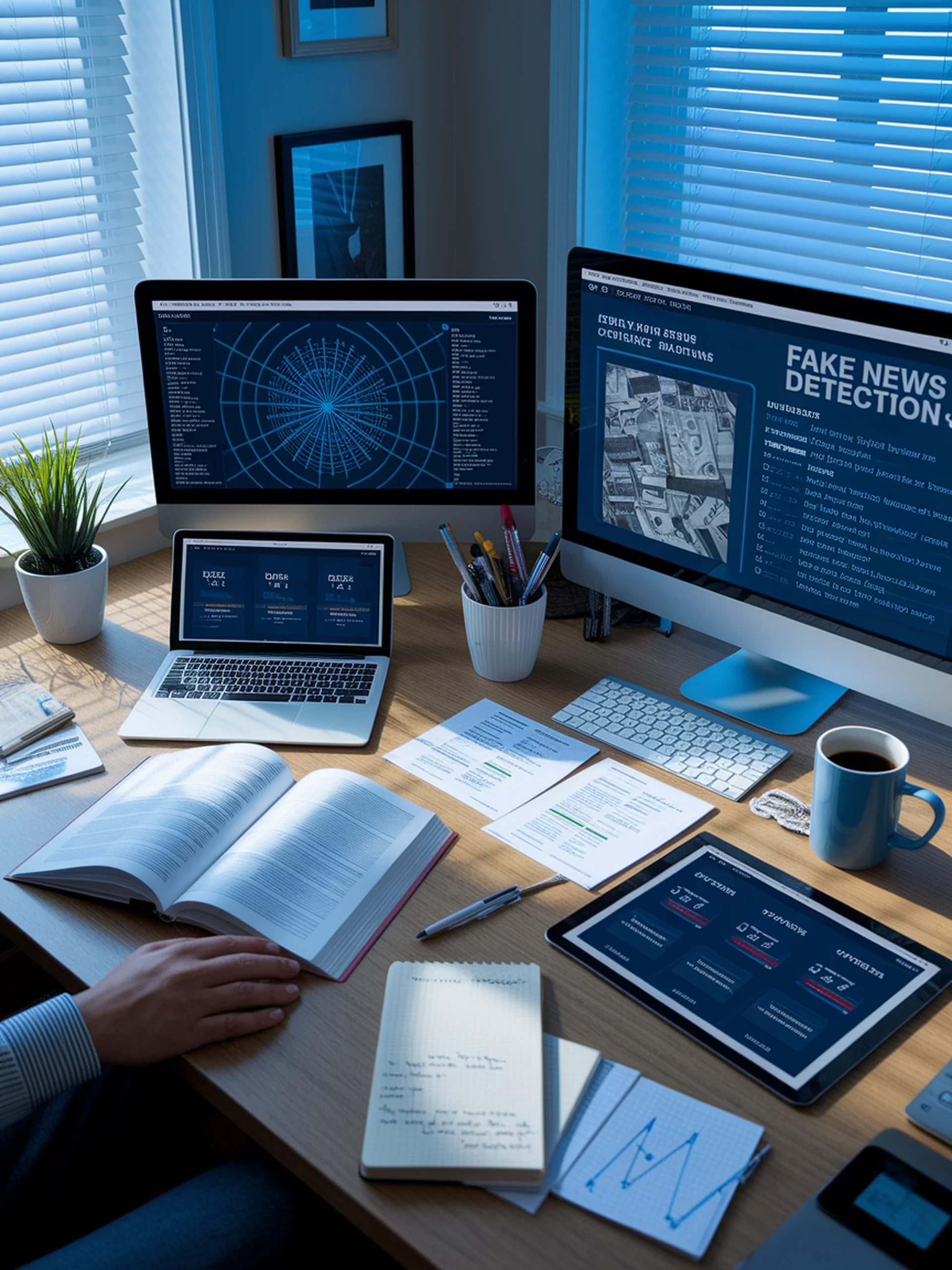
Improving Fake News Detection
Beyond Token-Based Models: Creating More Generalizable Detection Systems
This research addresses a critical challenge in fake news detection: models that perform well in lab settings but fail in real-world applications across different datasets.
- Identifies key limitations in current approaches that rely on publisher-based labels
- Explores how token-based models (TF-IDF, BERT) are sensitive to dataset-specific biases
- Investigates alternative features to improve generalization capability
- Examines Large Language Models as potential solutions to overcome generalization barriers
Security Impact: By improving the generalizability of fake news detection, this research strengthens information security defenses against misinformation campaigns that threaten democratic processes and public safety.
An exploration of features to improve the generalisability of fake news detection models