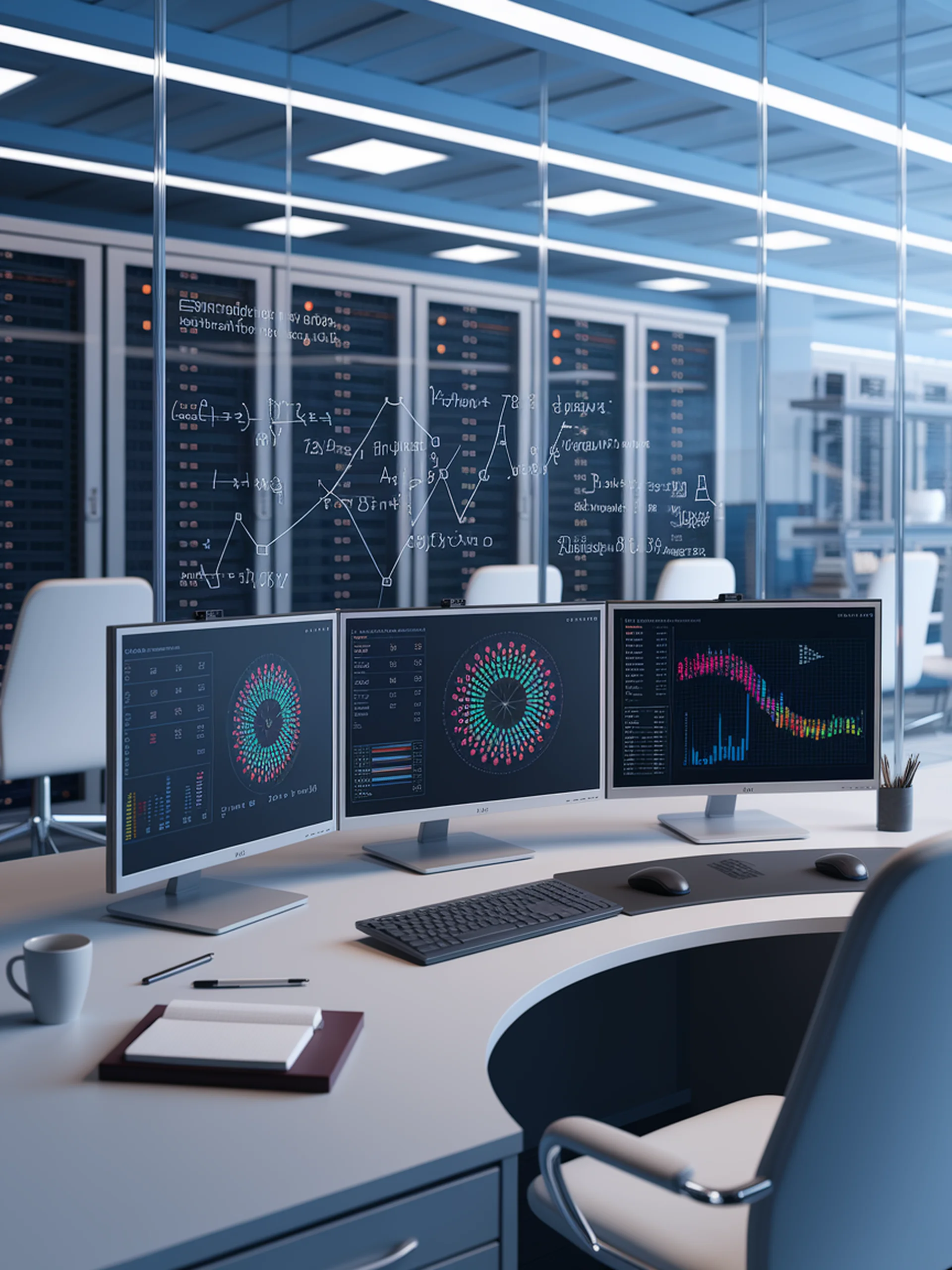
Evolving LLM Benchmarks
From Static to Dynamic Evaluation: Combating Data Contamination
This research examines the shift from static to dynamic benchmarking methods for large language models to address data contamination risks.
- Documents the evolution of benchmarking approaches designed to reduce contamination concerns
- Analyzes methods that enhance traditional static benchmarks
- Explores emerging dynamic evaluation techniques that create novel test scenarios
- Provides a comprehensive framework for evaluating LLM integrity
Why it matters for security: Data contamination threatens the reliability of LLM evaluations and potentially compromises model integrity. This research offers systematic approaches to ensure more accurate, trustworthy assessments of LLM capabilities.