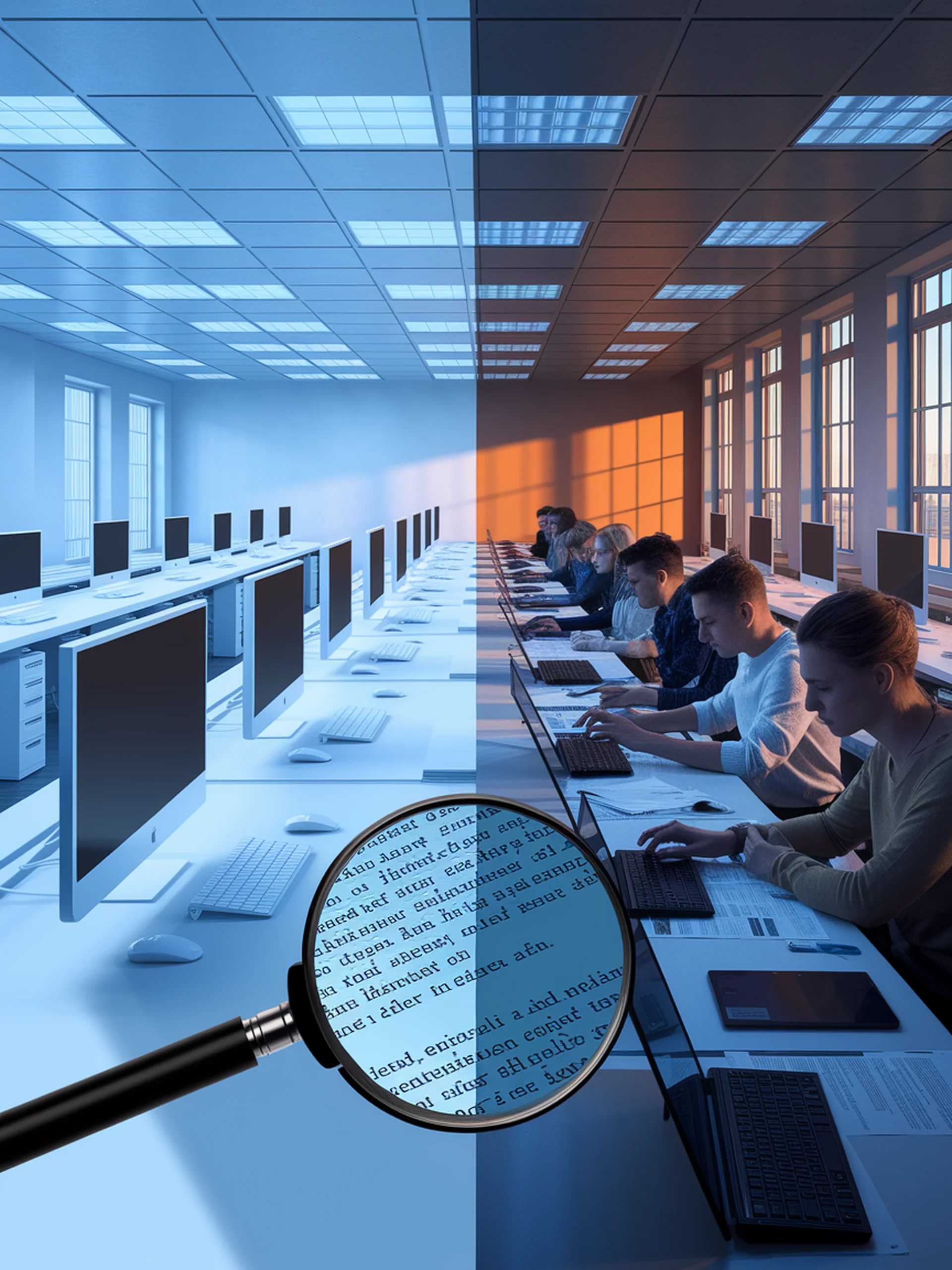
The Reality Gap in LLM Text Detection
Why current detectors fail in real-world scenarios
This research introduces DetectRL, a new benchmark revealing that even state-of-the-art LLM text detectors underperform in practical applications.
- Current detection methods show impressive results in lab settings but struggle with real-world text samples
- The benchmark covers domains highly susceptible to AI misuse (education, news, finance)
- Tests reveal significant performance gaps between controlled and real-world scenarios
- Provides insights for developing more robust detection systems for security applications
For security professionals, this work highlights critical vulnerabilities in our ability to detect AI-generated content that could be used for misinformation, academic dishonesty, or other harmful purposes.
DetectRL: Benchmarking LLM-Generated Text Detection in Real-World Scenarios