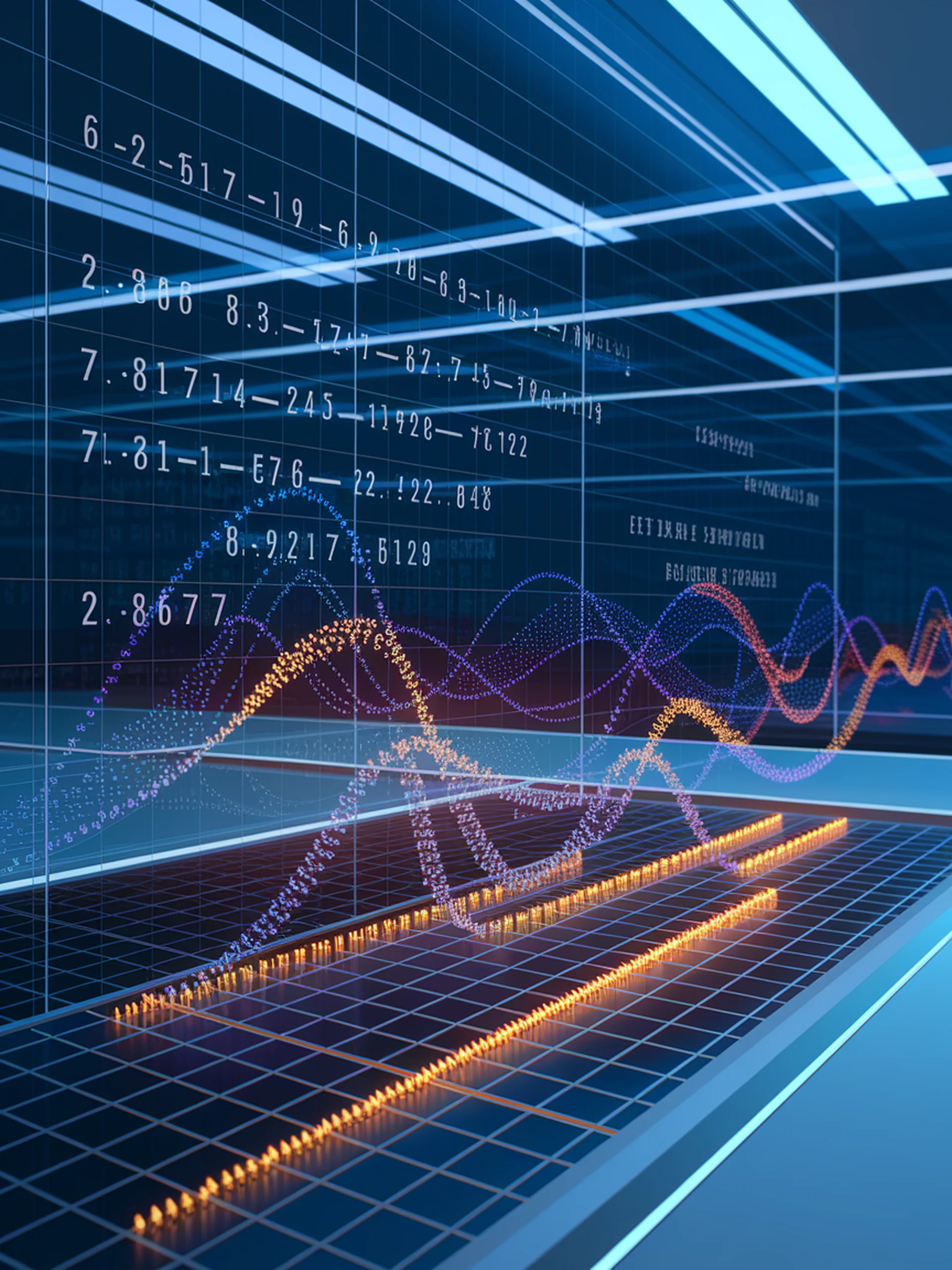
Modeling Self-Destructive Reasoning in LLMs
A mathematical framework for tracking toxicity amplification in language models
This research introduces a stochastic dynamical framework that models how LLMs can unintentionally amplify harmful content through their own reasoning processes.
- Models LLM reasoning as a continuous-time stochastic process with measurable severity variables
- Conceptualizes harmful content generation as a critical threshold phenomenon
- Provides mathematical tools to identify and prevent toxicity self-amplification
- Establishes foundation for more robust security guardrails in LLM systems
For security teams, this framework offers a principled approach to anticipate and mitigate cases where an LLM might generate increasingly harmful outputs through extended reasoning chains - a critical advancement for deploying safer AI systems.