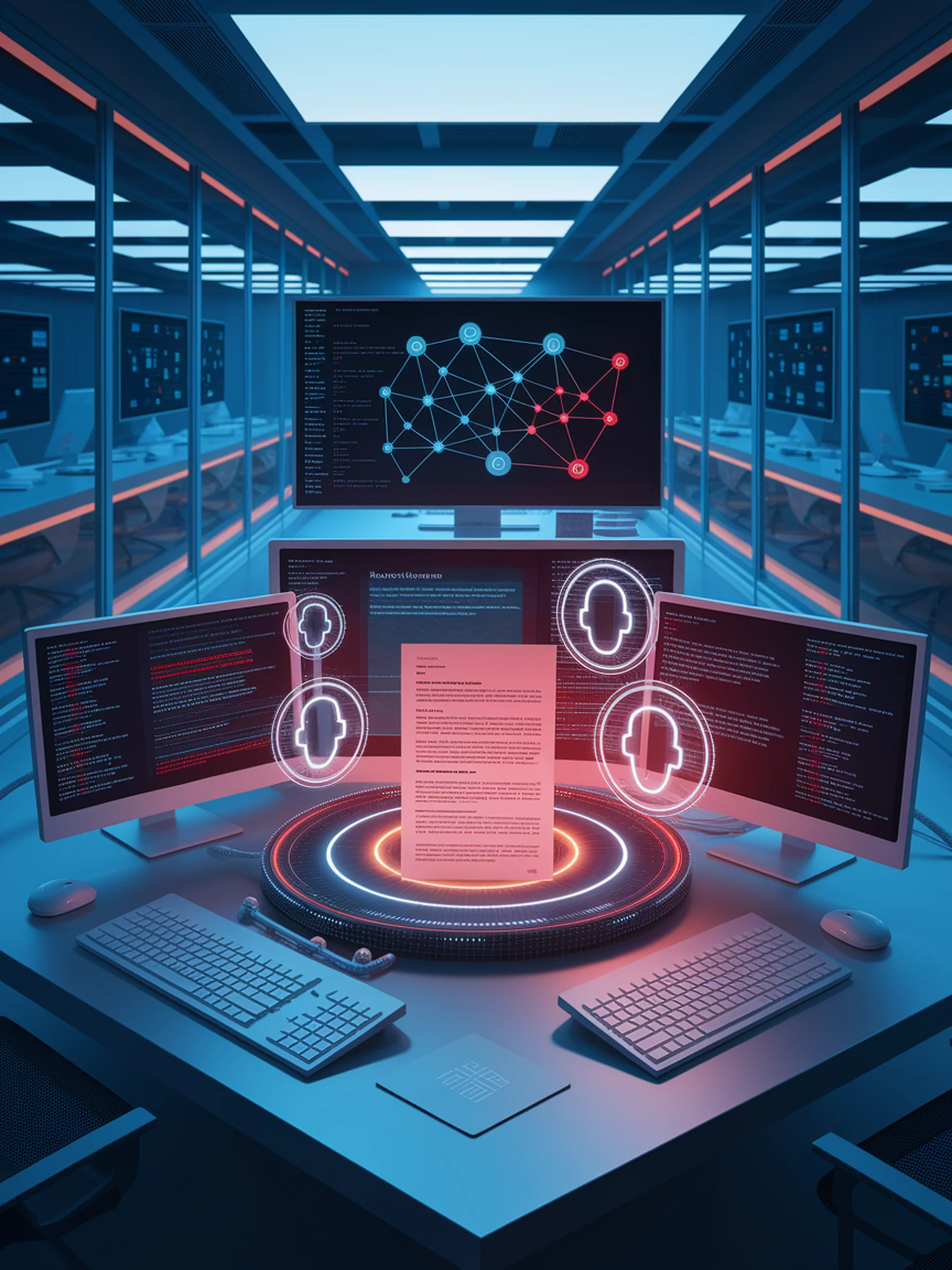
FACT-AUDIT: Dynamic Fact-Checking for LLMs
An adaptive multi-agent framework that uncovers LLMs' fact-checking limitations
FACT-AUDIT moves beyond static evaluation datasets to dynamically assess LLMs' fact-checking capabilities through an innovative agent-driven approach.
Key Innovations:
- Uses importance sampling to adaptively generate challenging evaluation cases
- Employs a multi-agent framework that reveals nuanced limitations in LLM fact-checking
- Evaluates both accuracy and justification production capabilities of LLMs
- Provides a more comprehensive assessment than traditional static methods
This research addresses critical security concerns by improving our ability to identify weaknesses in LLMs' factual reasoning, helping prevent the spread of misinformation and enhancing information security in AI systems.
Original Paper: FACT-AUDIT: An Adaptive Multi-Agent Framework for Dynamic Fact-Checking Evaluation of Large Language Models