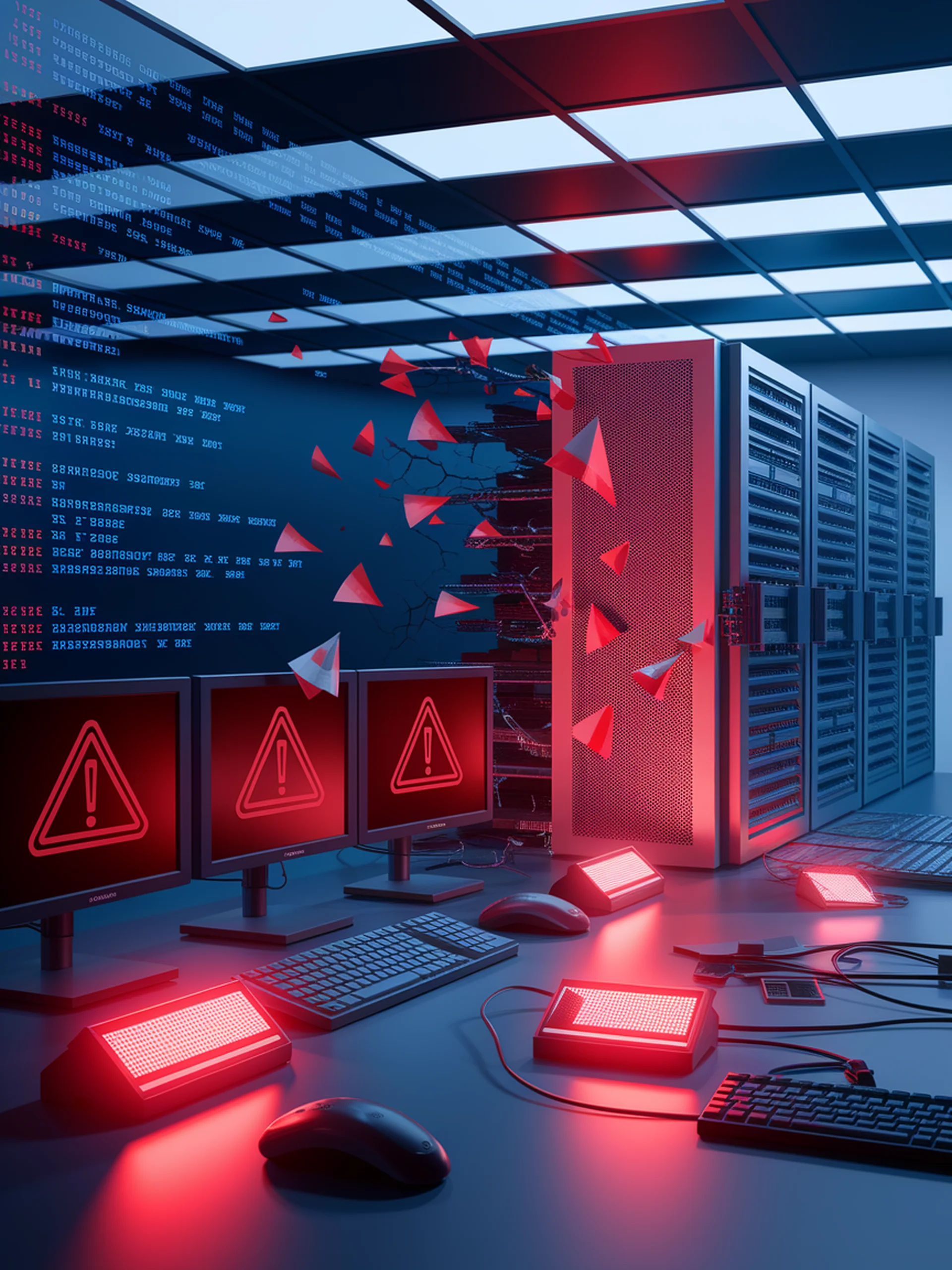
The Hidden Danger in LLM Safety Mechanisms
How attackers can weaponize false positives in AI safeguards
This research reveals a novel denial-of-service attack vector against LLMs by exploiting false positives in safety mechanisms, turning protective guardrails into vulnerabilities.
- Attackers can craft prompts that trigger safety rejections, preventing legitimate users from accessing LLM services
- The study demonstrates successful attacks against major commercial LLMs including GPT-4, Claude, and PaLM-2
- Proposed defenses include enhanced filtering techniques and runtime monitoring to detect suspicious patterns
- These attacks require minimal technical expertise yet can cause significant service disruption
For security teams, this research highlights the delicate balance between protection and availability in AI systems, requiring more sophisticated safeguards that resist manipulation while maintaining service reliability.
LLM Safeguard is a Double-Edged Sword: Exploiting False Positives for Denial-of-Service Attacks