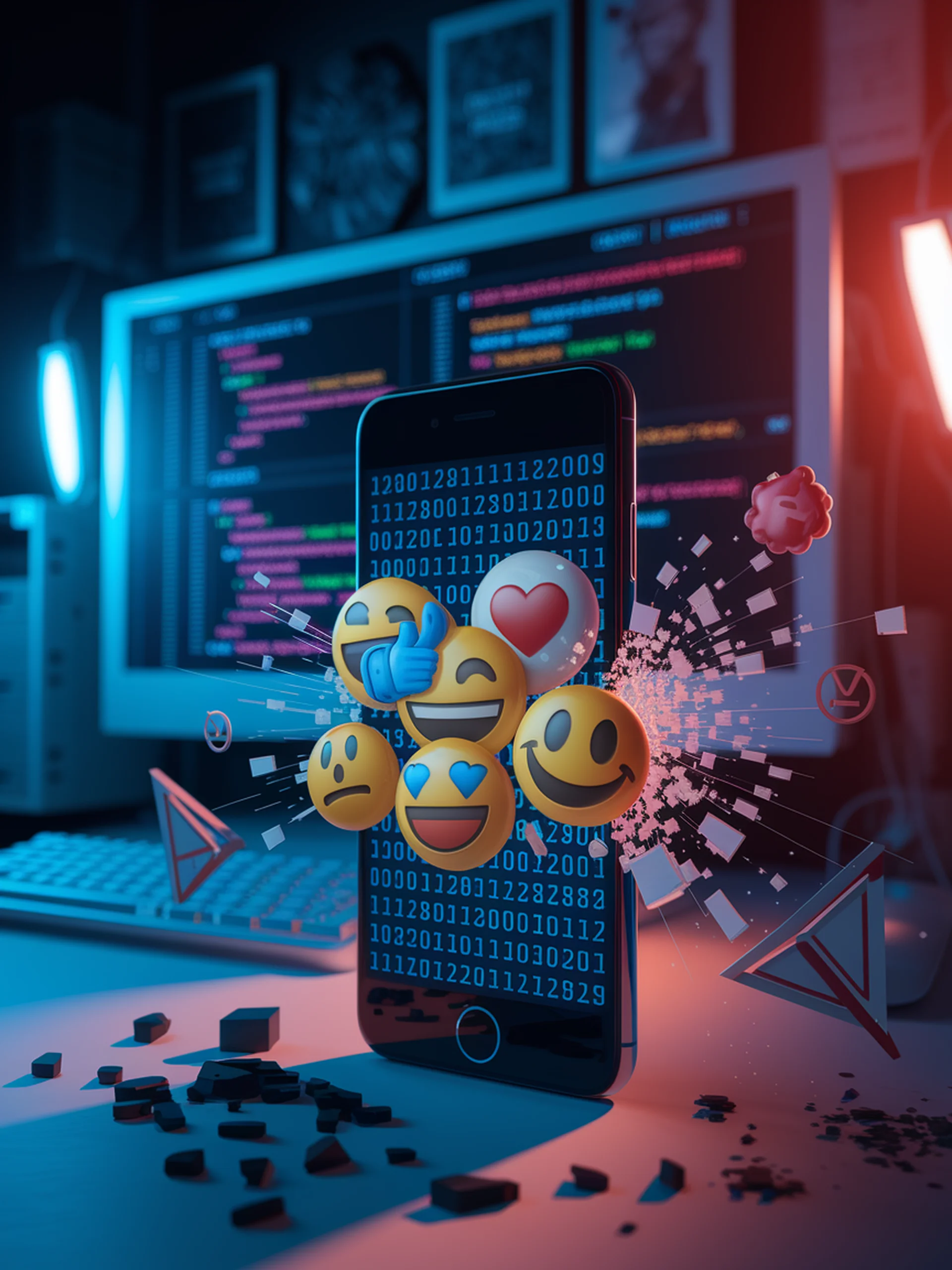
Emoji Attack: Undermining AI Safety Guards
How emojis can bypass safety detection systems in Large Language Models
This research reveals a critical vulnerability in Judge LLMs used to detect harmful AI outputs. By exploiting token segmentation bias with emojis, attackers can bypass safety measures.
- Introduces token segmentation bias as a fundamental vulnerability in Judge LLMs
- Demonstrates how innocuous emojis can drastically reduce detection accuracy
- Shows that current safety systems have a blind spot when tokens are segmented
- Highlights the urgent need for more robust defenses against these subtle attacks
This work is crucial for security professionals as it exposes how seemingly harmless characters can compromise AI safety guardrails, potentially allowing harmful content to reach users unchecked.
Original Paper: Emoji Attack: Enhancing Jailbreak Attacks Against Judge LLM Detection