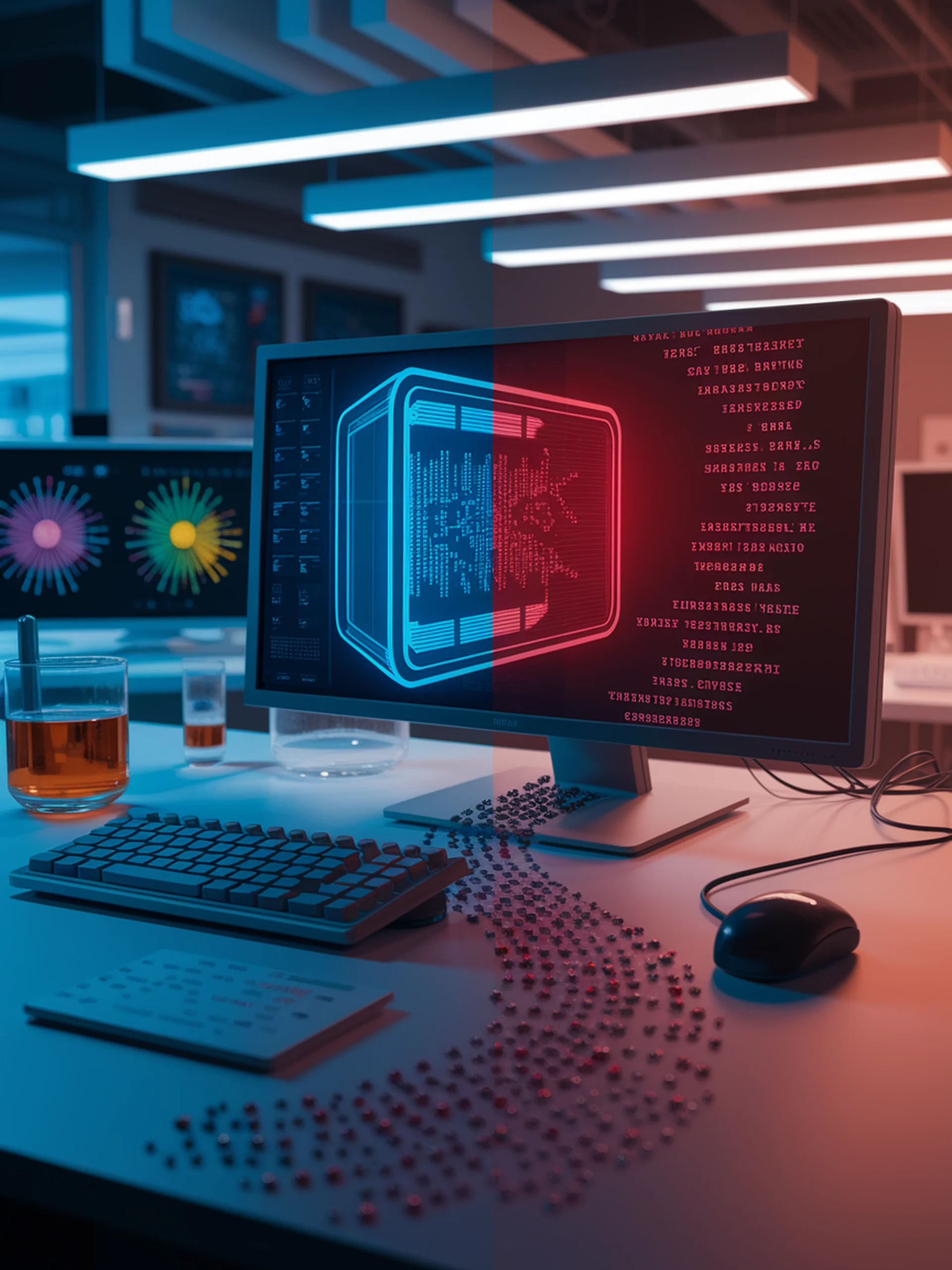
When Safety Training Falls Short
Testing LLM safety against natural, semantically-related harmful prompts
This research evaluates whether safety training in large language models effectively protects against natural harmful prompts that are semantically similar to known toxic content.
Key findings:
- Safety training shows limited generalization to semantically related natural prompts
- Researchers developed a new evaluation framework called SemAttack that generates natural variants of harmful prompts
- Models often fail to detect harm in reworded harmful requests, revealing critical security gaps
- Safety training appears to focus on specific phrases rather than understanding underlying harmful intent
For security professionals, this research highlights the need for more robust safety alignment techniques that address intent recognition rather than just filtering specific language patterns.
Does Safety Training of LLMs Generalize to Semantically Related Natural Prompts?