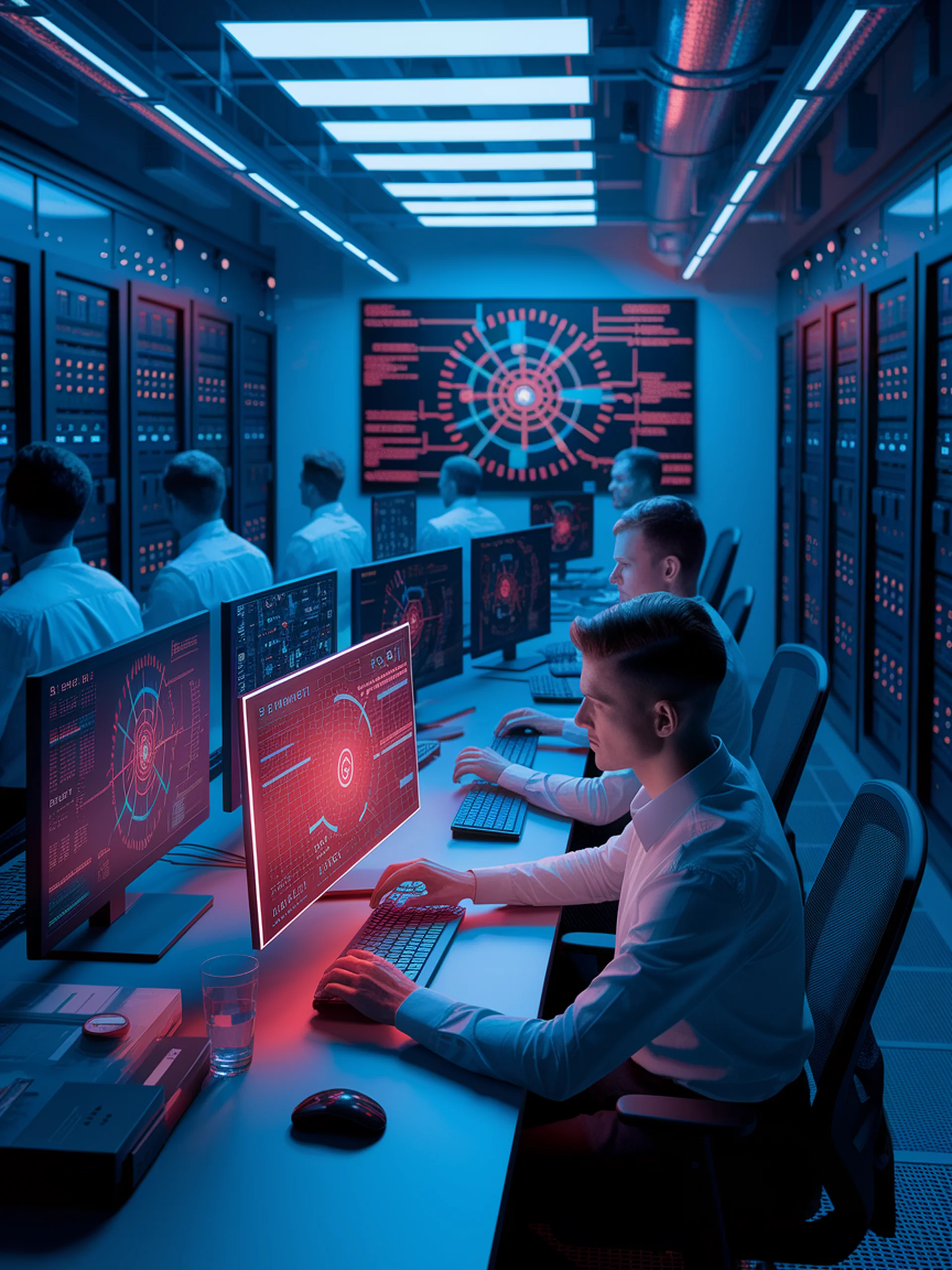
Guarding the Gates: LLM Security Red-Teaming
Detecting and preventing jailbreaking in conversational AI systems
This research introduces a novel framework for analyzing and preventing jailbreak attempts in conversational AI systems through systematic red-teaming of real-world interactions.
- Identified 105 unique jailbreak attempts from over 360,000 conversations with conversational agents
- Categorized attack patterns into alignment, parasocial, sexual, and access-focused strategies
- Developed a comprehensive taxonomy of jailbreaking techniques to improve defensive measures
- Found LLMs can effectively classify jailbreak attempts with 96% precision when properly prompted
This research provides critical insights for security professionals implementing guardrails and safety protocols in deployed LLM systems, helping to protect against unauthorized access while maintaining legitimate user experiences.
RICoTA: Red-teaming of In-the-wild Conversation with Test Attempts