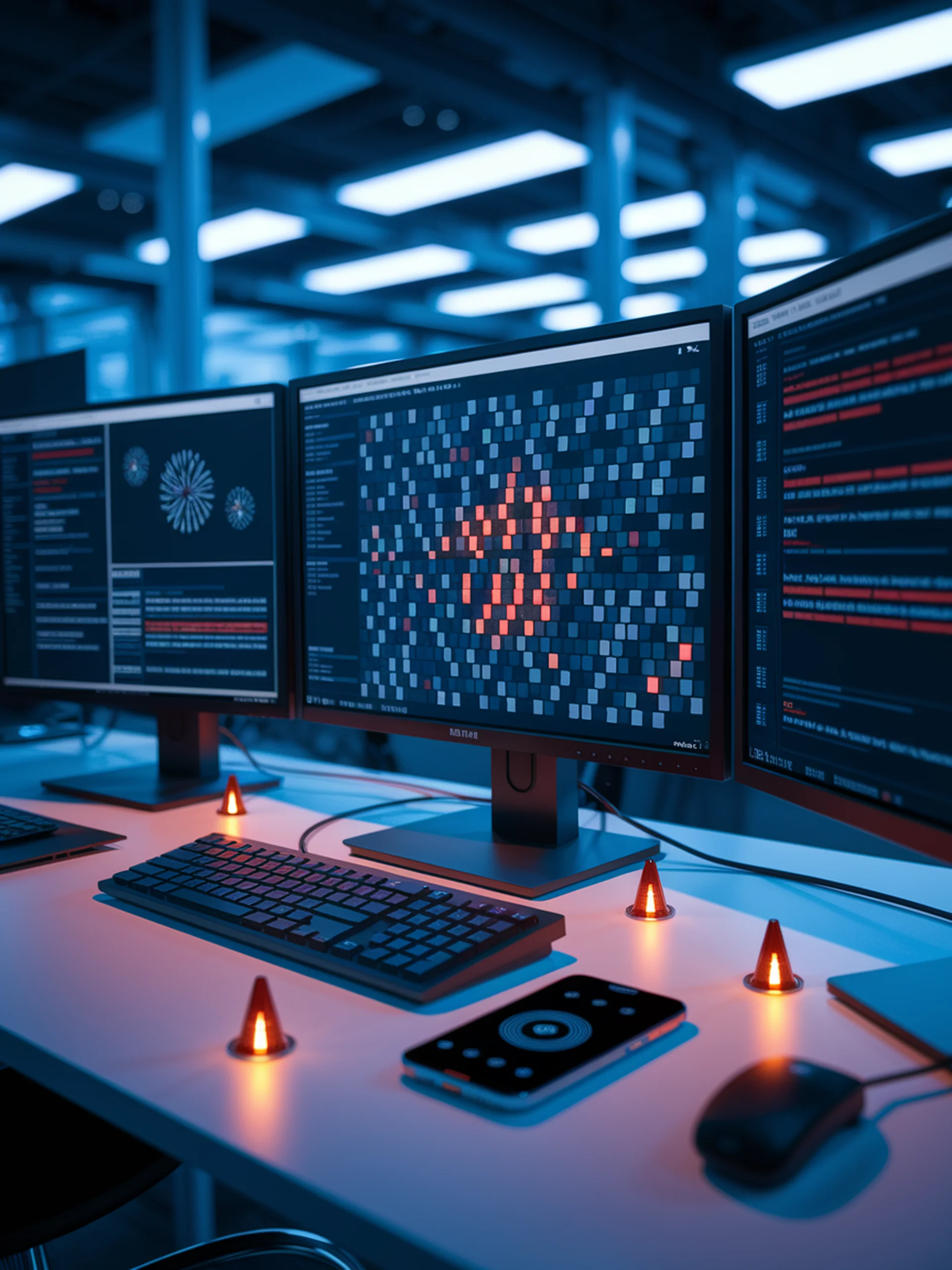
Breaking LLM Safeguards with Universal Magic Words
How embedding-based security can be bypassed with simple text patterns
Researchers discovered a fundamental vulnerability in text embedding models that form the basis of many LLM safety measures.
- Identified biased distribution patterns in text embedding outputs
- Created universal magic words that can bypass safety filters with 80-100% success rates
- Demonstrated attacks work across multiple commercial LLMs including GPT-4 and Claude
- Proposed practical defense mechanisms to mitigate these vulnerabilities
This research highlights critical security concerns as embedding models become more widely used in content filtering systems for commercial AI applications.
Jailbreaking LLMs' Safeguard with Universal Magic Words for Text Embedding Models