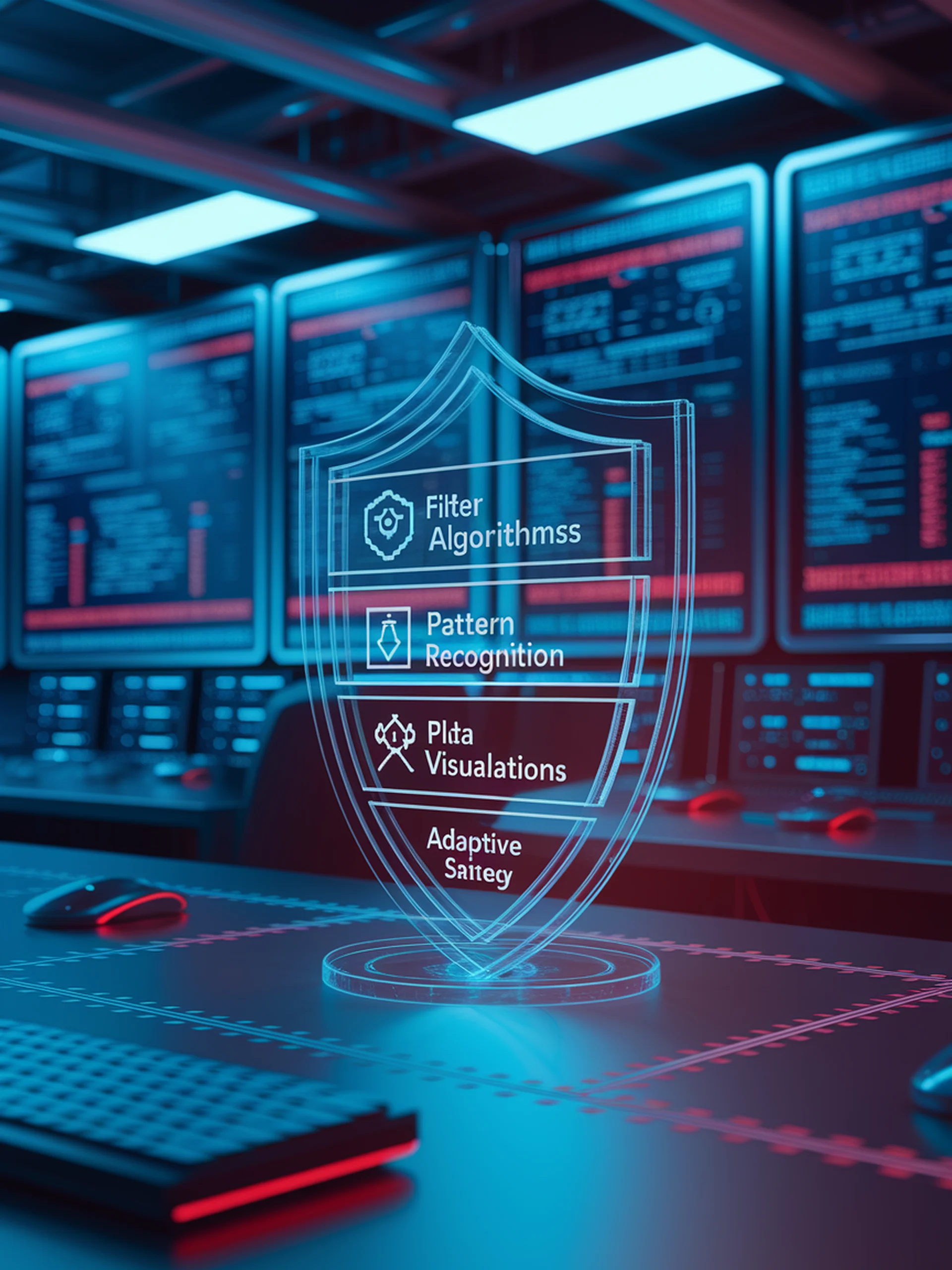
Countering LLM Jailbreak Attacks
A three-pronged defense strategy against many-shot jailbreaking
PANDAS introduces an advanced defense method that significantly reduces LLMs' vulnerability to many-shot jailbreaking attacks, where malicious prompts are hidden within hundreds of fabricated conversation turns.
- Positive Affirmation: Reinforces the model's safety guidelines by including examples of proper refusals
- Negative Demonstration: Explicitly shows the model examples of harmful requests and appropriate rejections
- Adaptive Sampling: Strategically selects defense examples based on the specific attack content
This research matters because it addresses a critical security vulnerability in large language models that could otherwise be exploited to generate harmful content, providing practical defense mechanisms that can be implemented by AI deployment teams to maintain safer AI systems.