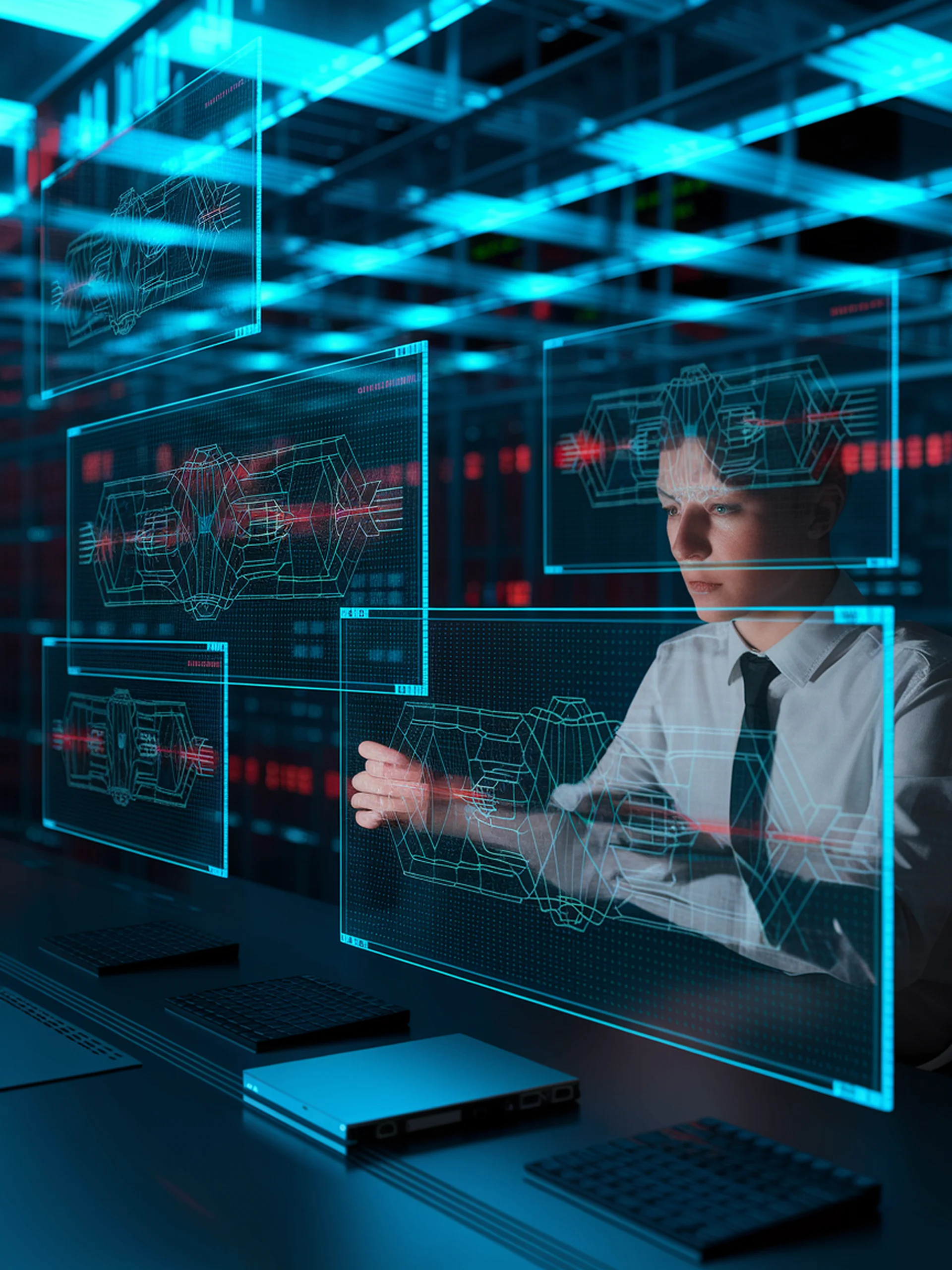
Reasoning-Enhanced Attacks on LLMs
A novel framework for detecting security vulnerabilities in conversational AI
This research introduces a powerful new approach to testing LLM security through multi-turn conversational attacks that more effectively expose safety vulnerabilities.
- Employs a reasoning-augmented framework that reformulates harmful queries into benign-looking conversations
- Achieves higher attack success rates while maintaining semantic coherence across multiple turns
- Demonstrates concerning evasion capabilities against current detection systems
- Highlights the need for more robust safety alignment techniques in production LLMs
This work matters for security professionals as it reveals how sophisticated attackers could potentially exploit LLMs in real-world settings through seemingly normal conversations, demonstrating the urgency for enhanced defensive measures.
Reasoning-Augmented Conversation for Multi-Turn Jailbreak Attacks on Large Language Models