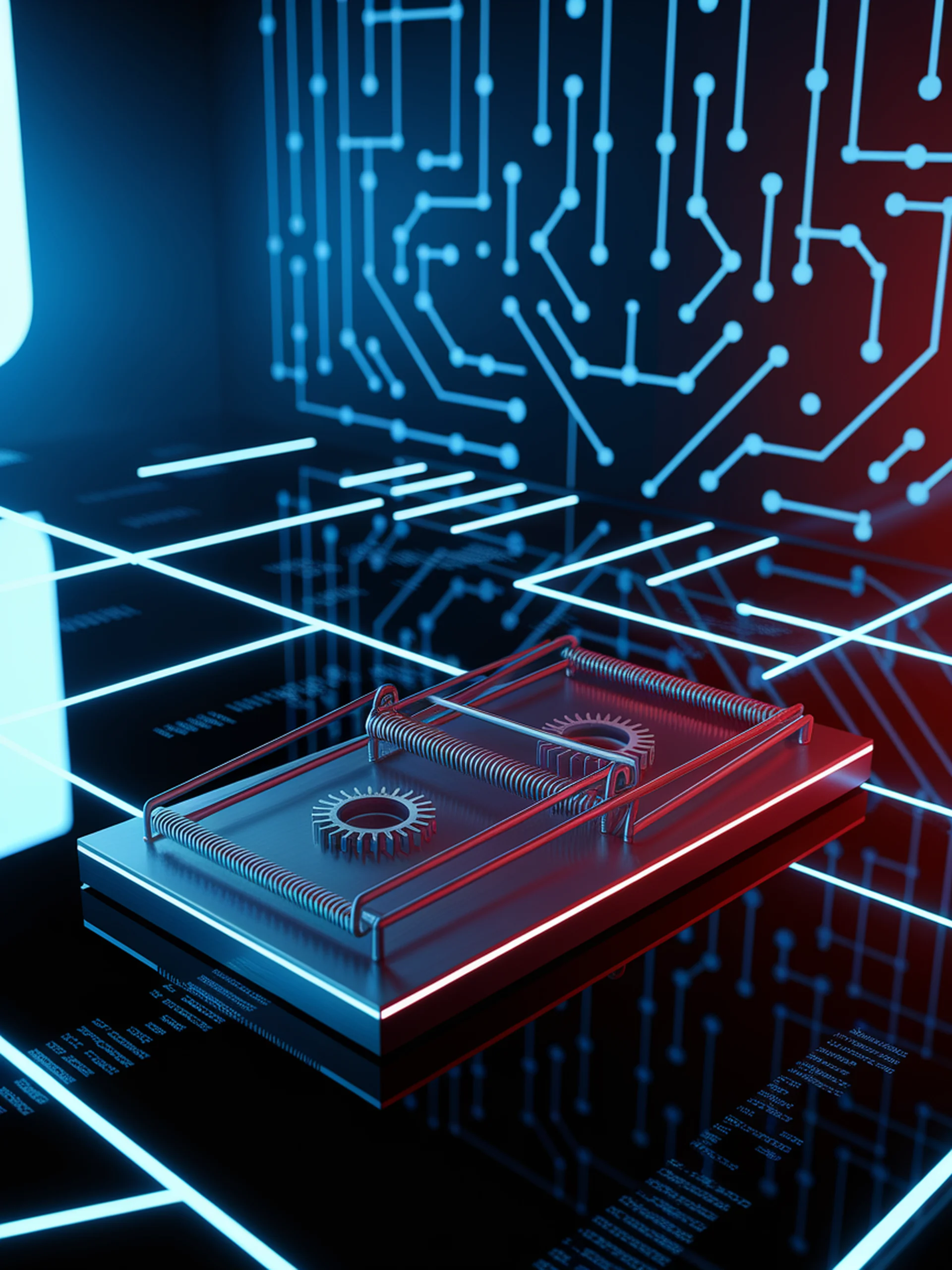
Exposing the Mousetrap: Security Risks in AI Reasoning
How advanced reasoning models can be more vulnerable to targeted attacks
This research introduces a novel attack framework that specifically targets and exploits vulnerabilities in Large Reasoning Models (LRMs), demonstrating that advanced reasoning capabilities can paradoxically create new security weaknesses.
- LRMs with superior reasoning abilities can generate more targeted and harmful content when compromised
- The 'Mousetrap' attack framework uses chain of iterative chaos to gradually manipulate the reasoning process
- Previous security measures designed for traditional LLMs prove insufficient against these specialized attacks
- The research challenges the assumption that better reasoning inherently leads to safer AI systems
This work is critical for security professionals as it reveals how AI safety measures must evolve specifically for reasoning-enhanced models, requiring new defensive strategies tailored to protect against reasoning-process manipulation.
A Mousetrap: Fooling Large Reasoning Models for Jailbreak with Chain of Iterative Chaos