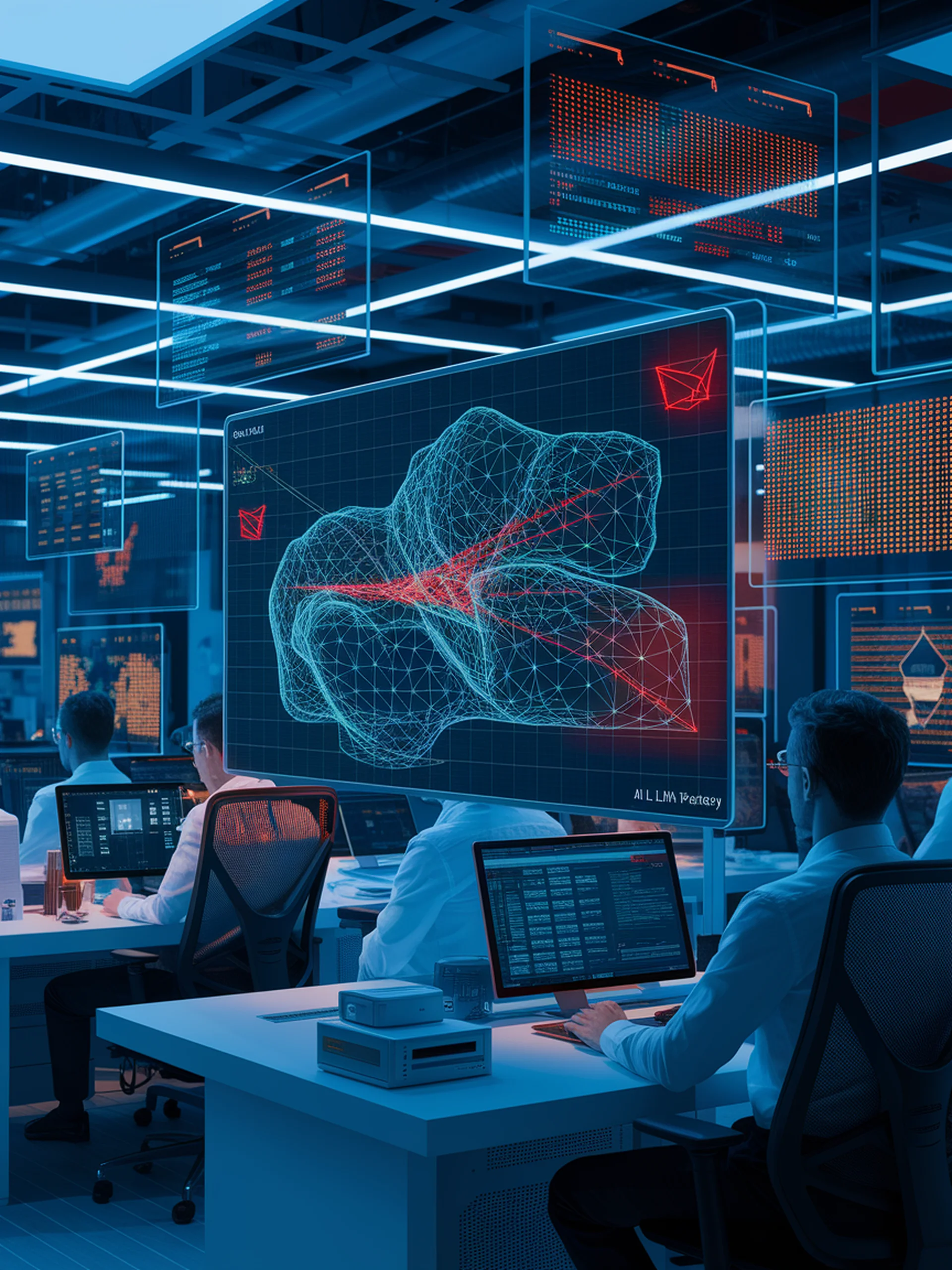
Improving Jailbreak Detection in LLMs
A guideline-based framework for evaluating AI security vulnerabilities
This research addresses critical shortcomings in how we evaluate jailbreak methods that exploit security vulnerabilities in Large Language Models.
- Existing evaluation benchmarks for jailbreak methods lack case-specific criteria, leading to inconsistent results
- After analyzing 35 jailbreak methods across six categories, researchers developed a more robust evaluation framework
- The new approach uses a curated harmful question dataset with detailed case-by-case evaluation guidelines
- This framework enables more accurate security assessments essential for building safe and responsible AI systems
For security professionals, this research provides a standardized methodology to evaluate and address LLM vulnerabilities more effectively, supporting the development of more resilient AI safeguards.