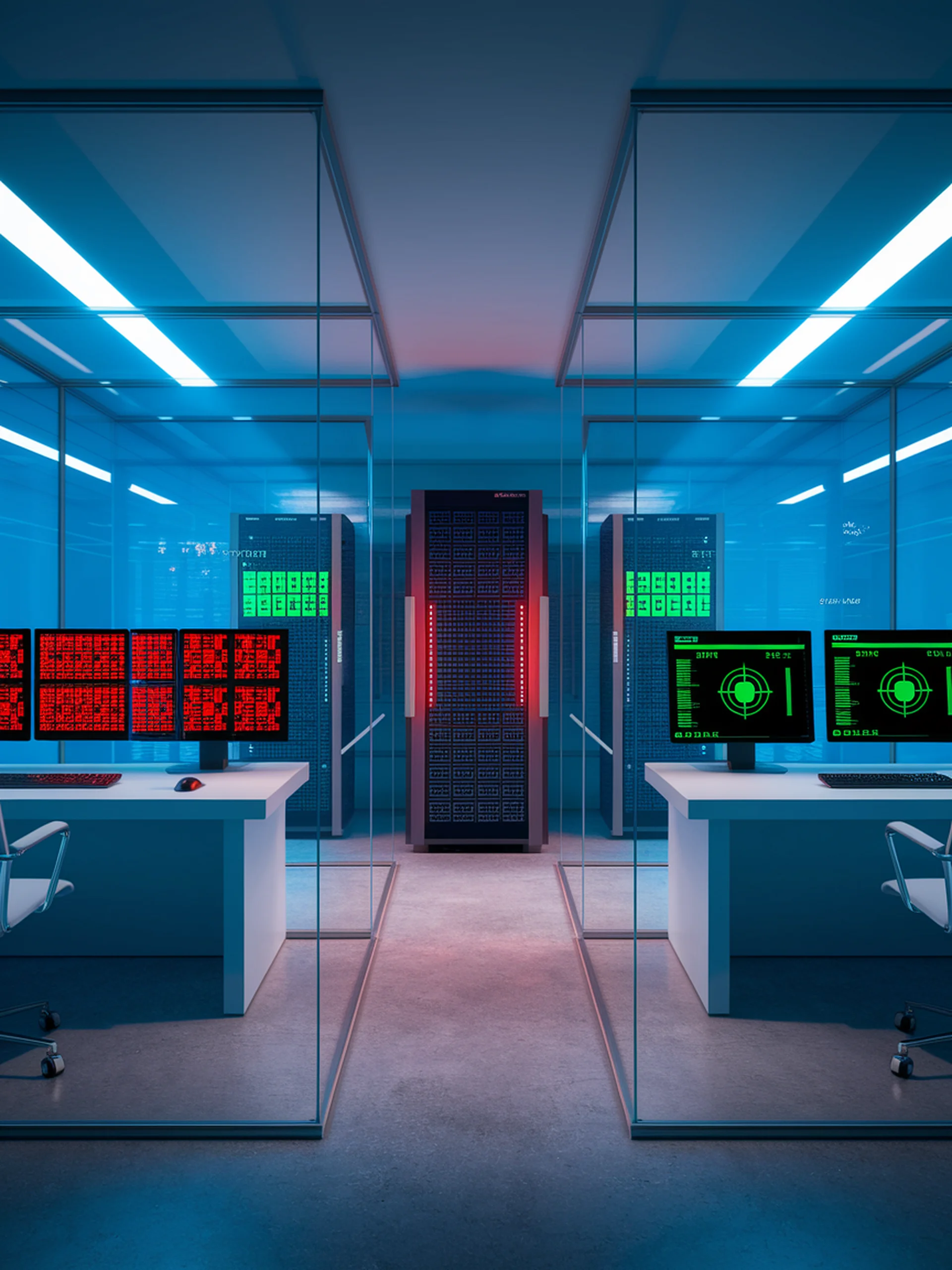
Fortifying LLM Defenses
A dual-objective approach to prevent jailbreak attacks
This research introduces a dual-objective optimization framework that significantly improves LLM safety alignment by addressing critical flaws in current methods like Direct Preference Optimization (DPO).
- Identifies theoretical limitations in DPO's loss function that make it vulnerable to jailbreak attacks
- Separates alignment into two distinct objectives: robust refusal learning and harmless helpfulness
- Demonstrates superior performance against adversarial attacks while maintaining helpful responses to legitimate requests
- Provides a gradient-based analysis that explains why current methods fail
This advancement is crucial for security as it creates more resilient AI systems that can better identify and refuse harmful instructions while remaining useful for appropriate tasks—essential for deploying LLMs in high-stakes environments.
Improving LLM Safety Alignment with Dual-Objective Optimization