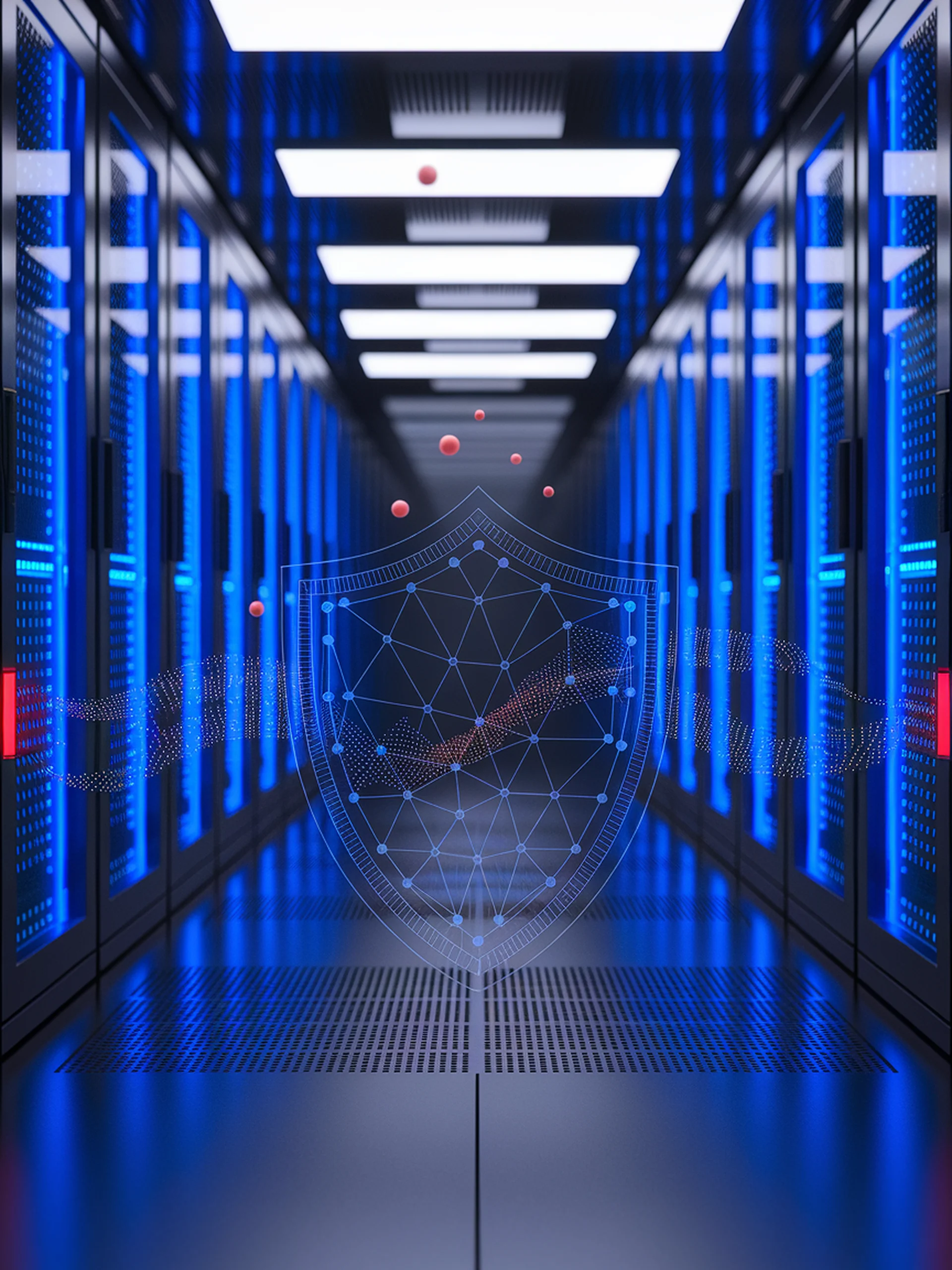
Securing Multimodal AI Systems
A Probabilistic Approach to Detecting and Preventing Jailbreak Attacks
This research introduces a groundbreaking probabilistic framework for assessing and defending against jailbreak attacks on Multimodal Large Language Models (MLLMs).
- Moves beyond binary (success/fail) classification to measure jailbreak probability across multiple queries
- Develops a novel attack method leveraging this probabilistic approach to breach MLLM defenses
- Creates an effective defensive mechanism that adapts to potential threats
- Demonstrates significant improvements in both attack detection and prevention
As MLLMs become increasingly integrated into business applications, this research provides crucial insight for security teams needing to protect AI systems from harmful exploitation while maintaining functionality.
Utilizing Jailbreak Probability to Attack and Safeguard Multimodal LLMs