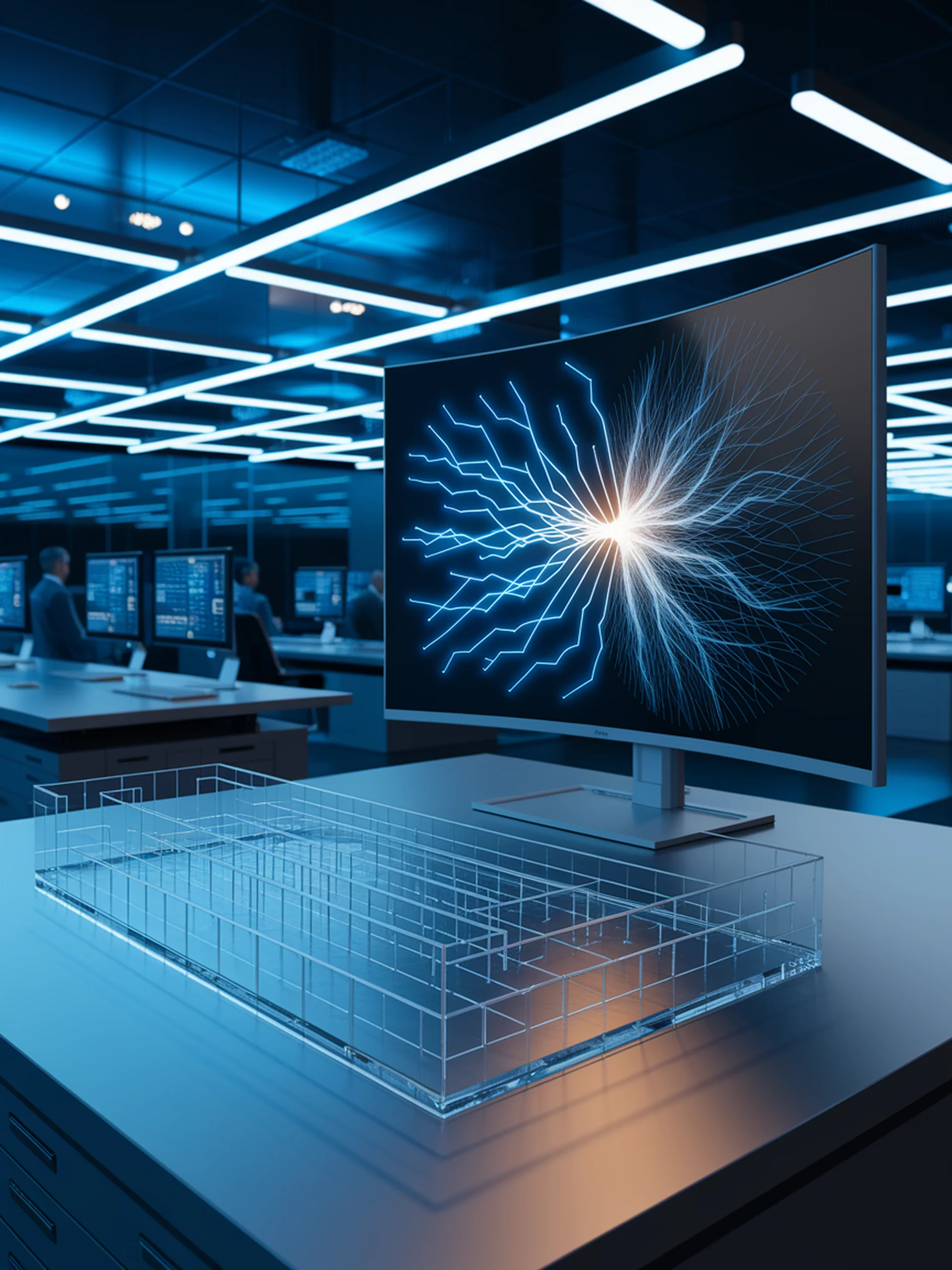
Securing LLMs Against Harmful Outputs
A Novel Representation Bending Approach for Enhanced Safety
RepBend is a new technique that modifies internal representations within large language models to enhance safety without compromising performance.
- Addresses critical safety risks in LLMs including harmful content generation and vulnerability to adversarial attacks
- Operates by bending the representation space to prevent harmful outputs while preserving model functionality
- Demonstrates effectiveness across multiple popular LLM architectures
- Offers a more robust safety solution compared to traditional fine-tuning approaches
This research is crucial for security as it provides a fundamental approach to hardening LLMs against jailbreak attempts and harmful use cases, essential for deploying these models in high-stakes environments.