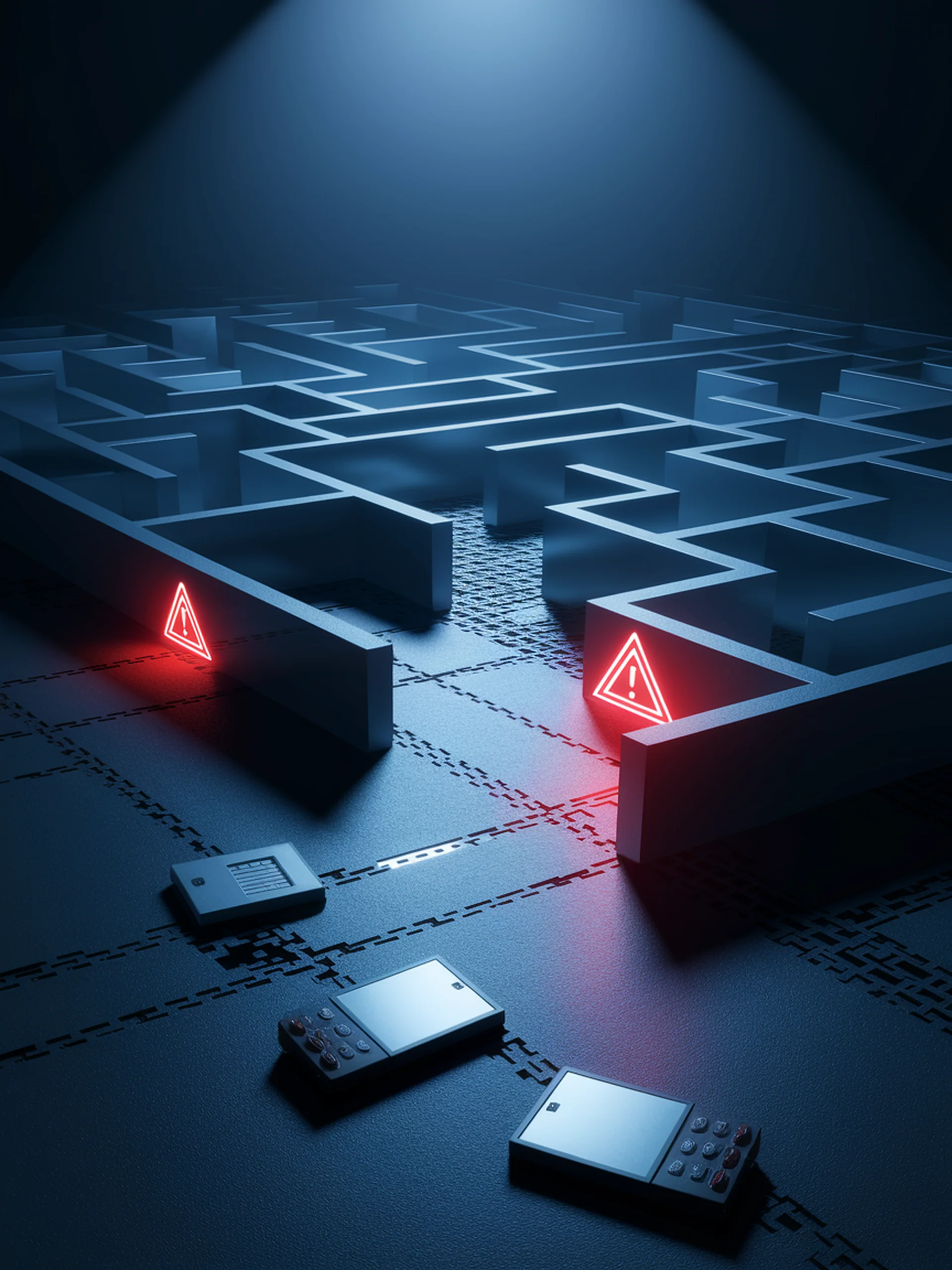
Rethinking LLM Unlearning Evaluations
Current benchmarks overestimate unlearning effectiveness
This research reveals significant flaws in how we measure large language models' ability to forget sensitive or harmful information.
- Existing LLM unlearning benchmarks provide overly optimistic and potentially misleading effectiveness measures
- Simple, benign modifications to evaluation methods reveal these benchmarks are weak indicators of actual unlearning
- Current evaluation practices may create a false sense of security about LLM privacy and safety controls
- Researchers need more robust evaluation frameworks to accurately assess unlearning methods
For security professionals, this work highlights critical gaps in our ability to verify that sensitive information has truly been removed from AI systems, calling for more rigorous testing standards before deploying unlearning methods in production.
Position: LLM Unlearning Benchmarks are Weak Measures of Progress