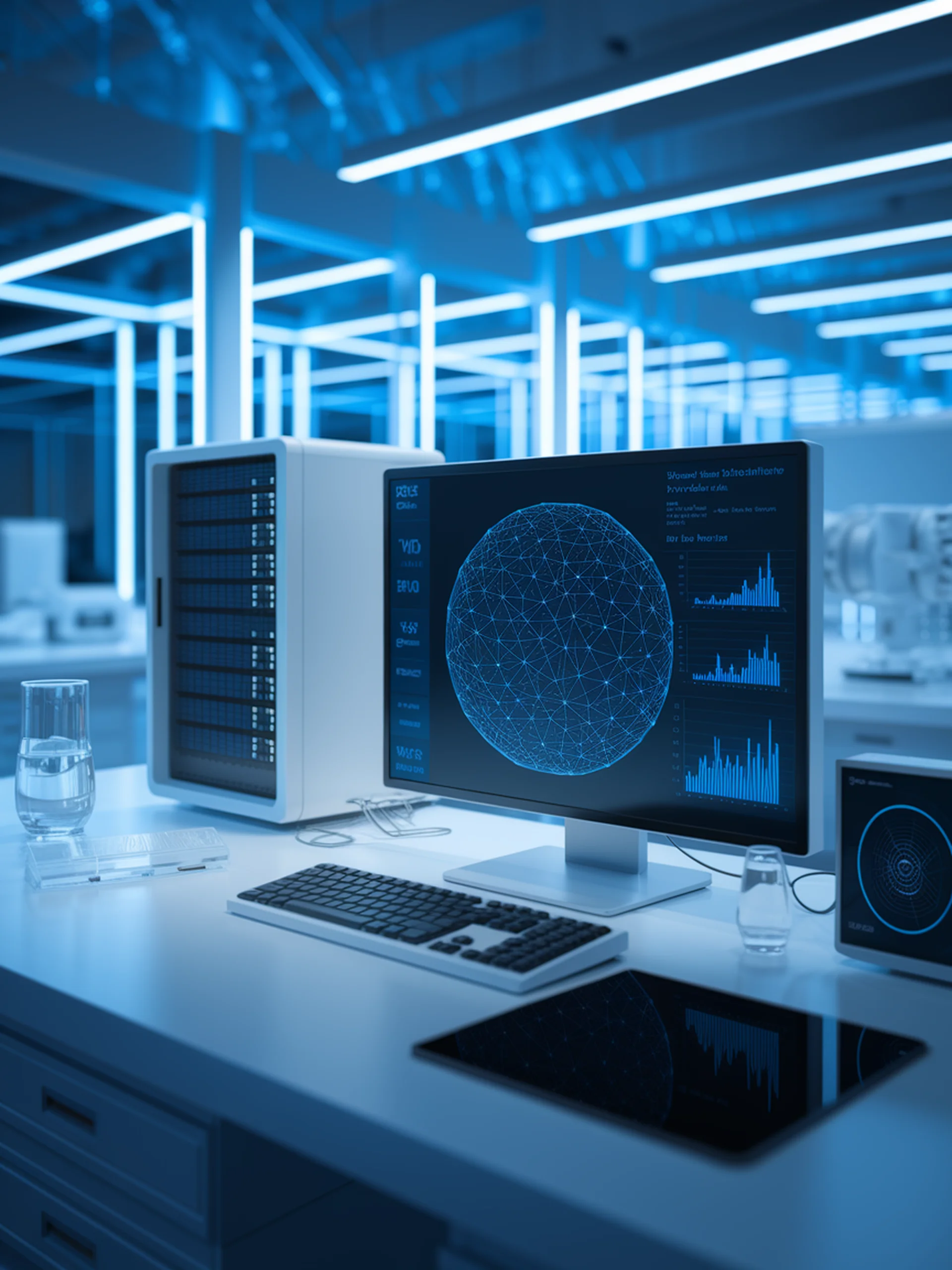
Strategic Unlearning in LLMs
Precision-targeted weight modification for secure AI models
WAGLE introduces a novel approach for selectively removing knowledge from large language models without compromising overall performance.
- Weight-centric approach that identifies and modifies only the most relevant parameters
- Achieves up to 16.4% superior forgetting efficiency compared to existing methods
- Maintains model utility while effectively removing targeted information
- Modular design allows for practical implementation in production environments
This research addresses critical security concerns by providing organizations with efficient mechanisms to comply with data regulations and prevent malicious use of AI systems, while preserving valuable model capabilities.
WAGLE: Strategic Weight Attribution for Effective and Modular Unlearning in Large Language Models