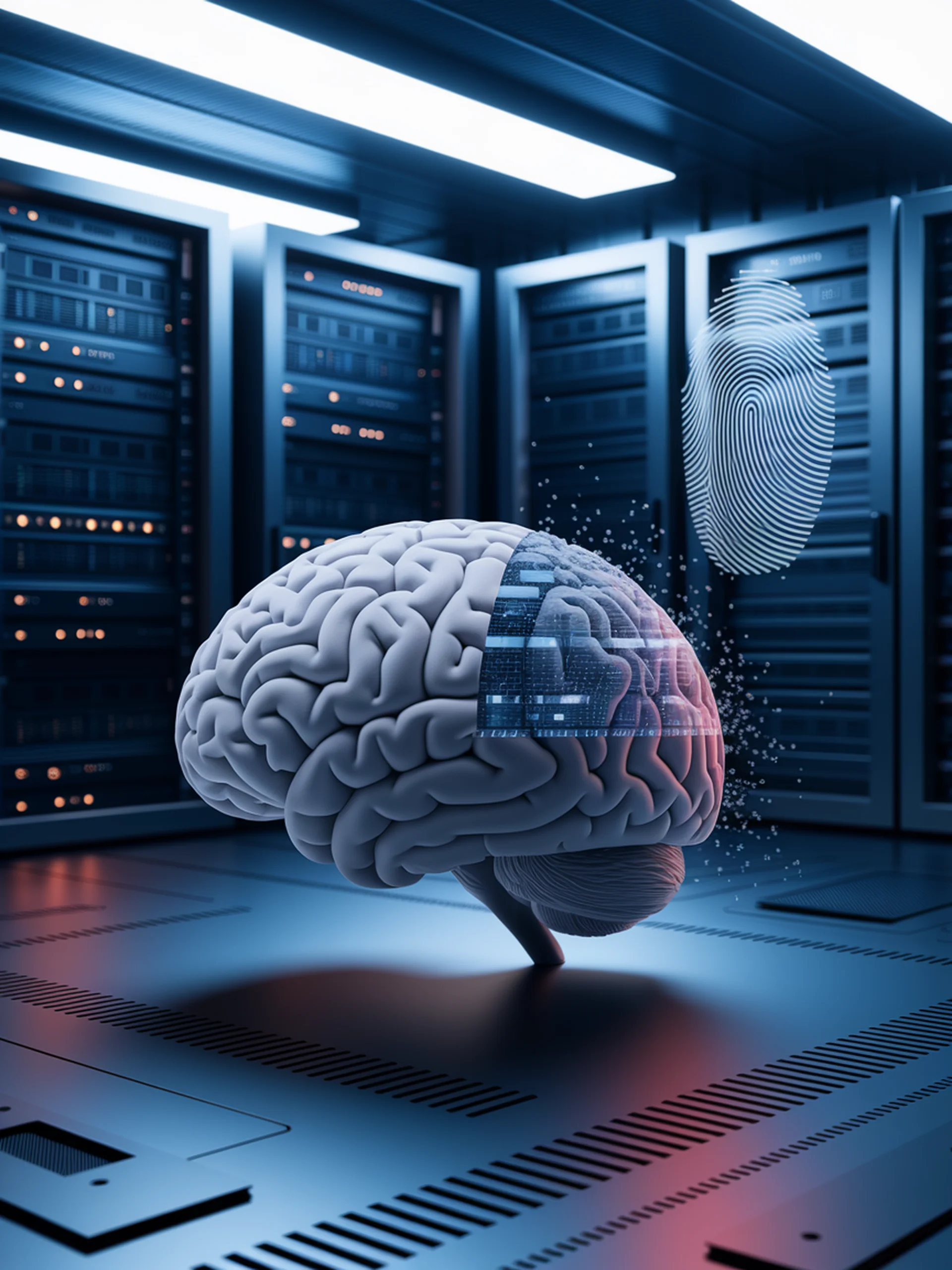
The Illusion of Forgetting in LLMs
Why soft token attacks fail as reliable auditing tools for machine unlearning
This research challenges the effectiveness of soft token attacks as reliable methods to verify if LLMs have truly 'forgotten' data through machine unlearning techniques.
Key findings:
- Soft token attacks perform no better than random guessing when auditing unlearning in LLMs
- Current unlearning verification methods are fundamentally flawed in determining if sensitive data has been removed
- The study reveals significant security implications for organizations relying on unlearning to protect sensitive information
- Researchers demonstrated results across multiple models and datasets, highlighting a systematic vulnerability
This research matters for security professionals because it exposes critical gaps in our ability to verify if sensitive data has been properly removed from AI models, raising important questions about compliance with privacy regulations and data protection standards.
Soft Token Attacks Cannot Reliably Audit Unlearning in Large Language Models