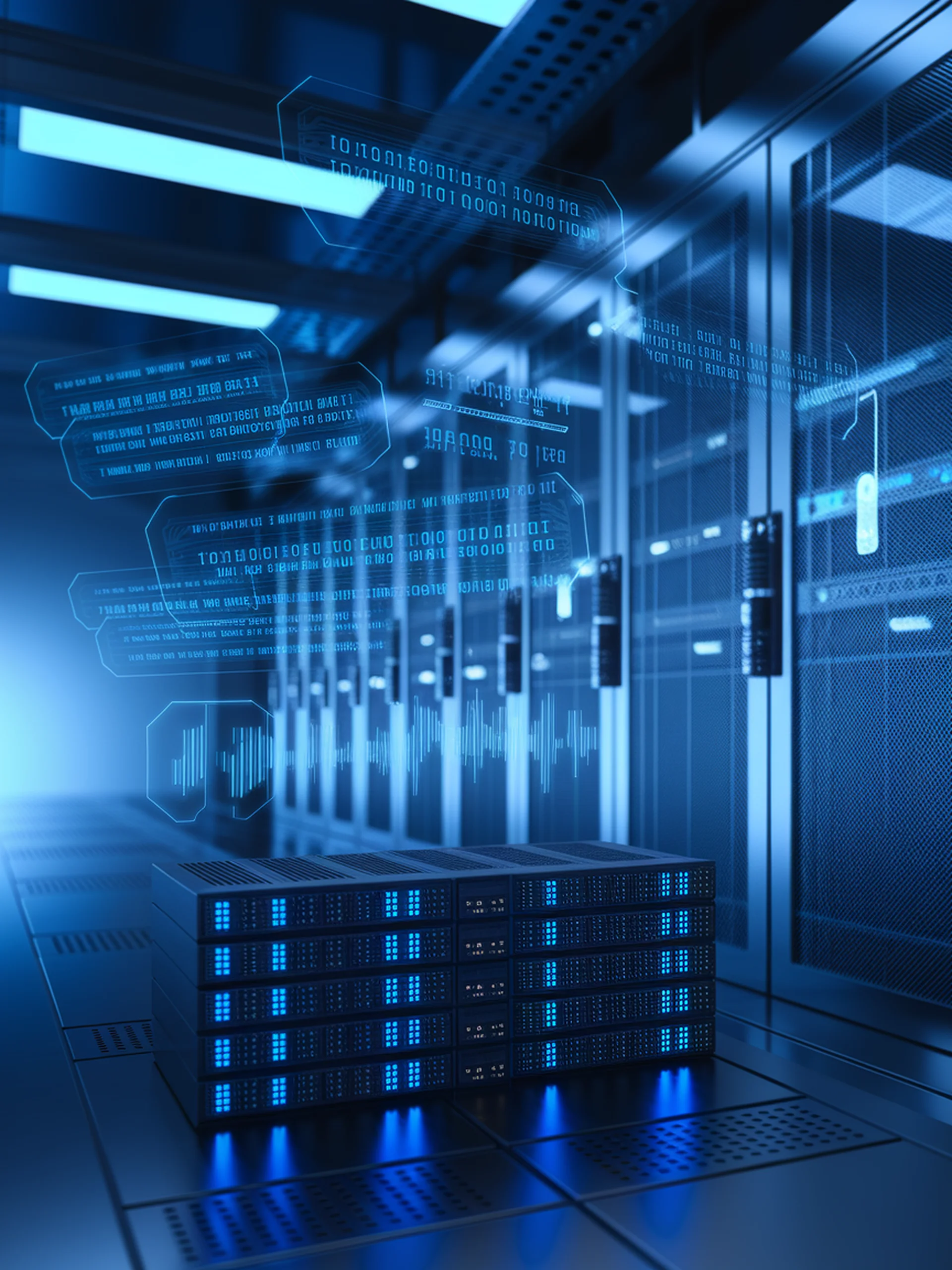
Erasing Sensitive Data from AI Models
A More Effective Approach to AI Unlearning
CE-U (Cross Entropy Unlearning) offers a superior method for removing sensitive information from large language models, addressing fundamental limitations of previous techniques.
- Solves the vanishing/exploding gradient problems that plague standard unlearning approaches
- Creates a unified framework that brings together learning and unlearning processes
- Achieves state-of-the-art performance on the TOFU benchmark for machine unlearning
- Enhances model security by effectively removing memorized sensitive data
This research provides crucial capabilities for organizations needing to protect user privacy and comply with data removal regulations, offering a more reliable way to delete specific information from AI systems without retraining.