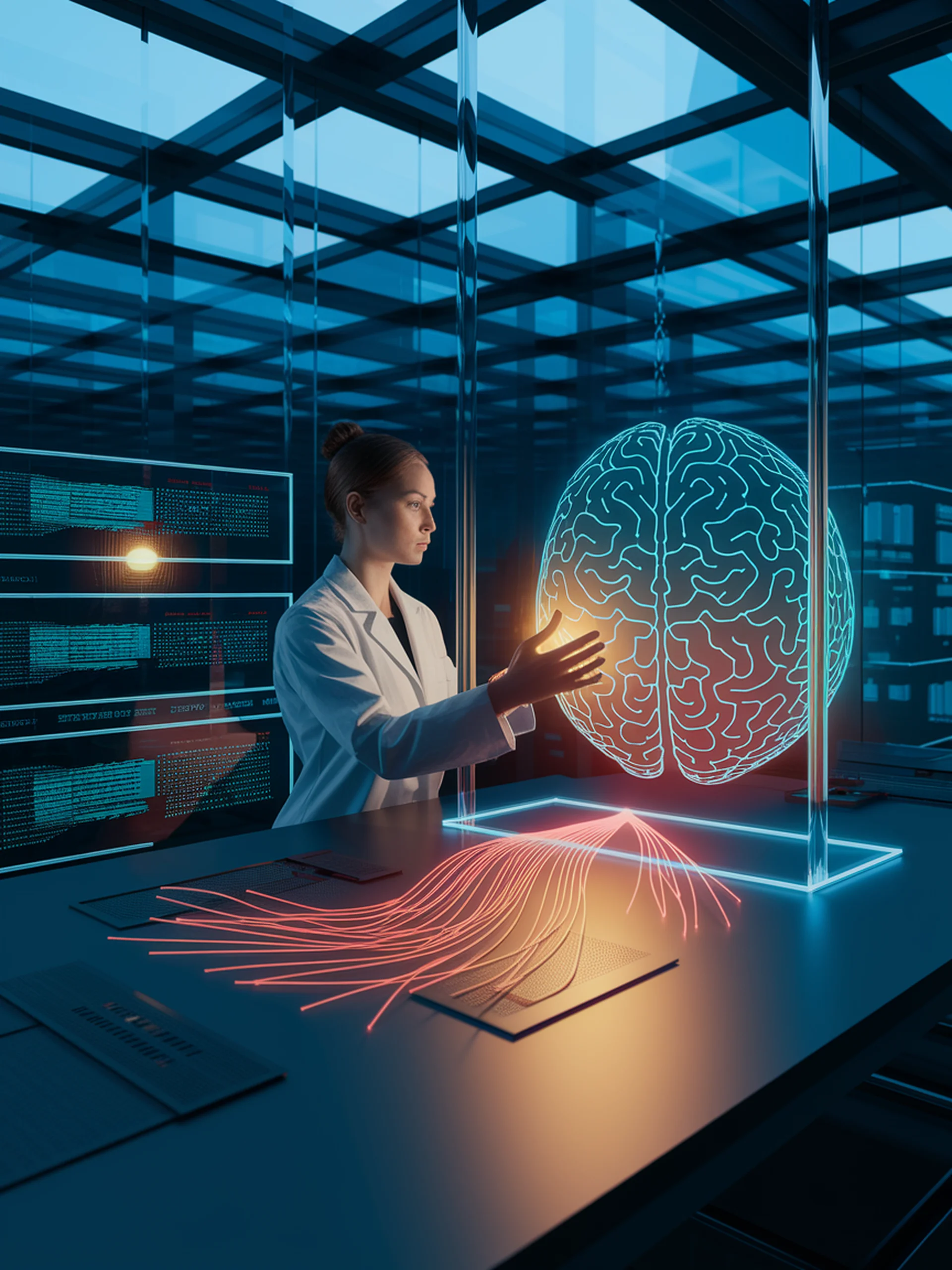
Balancing Unlearning & Retention in LLMs
A Gradient-Based Approach to Selective Knowledge Removal
GRU framework offers a solution to the critical trade-off between removing harmful content from LLMs while preserving general capabilities.
- Analyzes gradients during unlearning to identify and preserve essential knowledge
- Reduces performance degradation that typically occurs during unlearning processes
- Enables more precise removal of privacy and copyright-related responses
- Maintains model functionality while enhancing security and legal compliance
This research is vital for security professionals as it provides a pathway to deploy safer AI systems that can selectively remove harmful content without compromising overall performance—a key requirement for responsible AI deployment.
GRU: Mitigating the Trade-off between Unlearning and Retention for Large Language Models