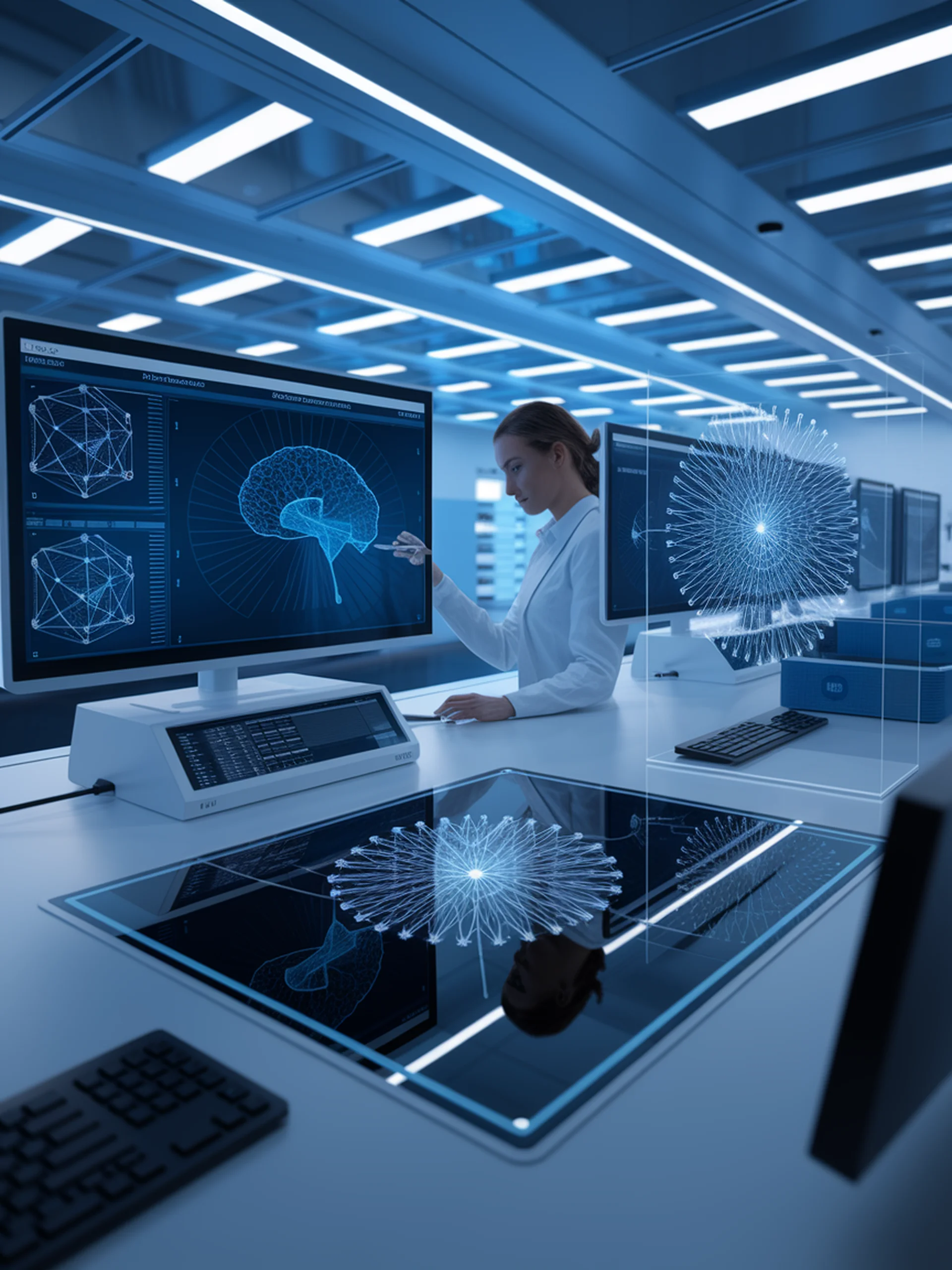
Selective Concept Unlearning for AI Security
Fine-grained knowledge removal in vision-language models using sparse autoencoders
SAUCE introduces a novel approach for selectively removing specific concepts from vision-language models without degrading overall performance, addressing critical security and privacy concerns.
- Leverages sparse autoencoders to enable precise, targeted concept removal
- Performs unlearning at a fine-grained level rather than broad knowledge erasure
- Maintains model utility while effectively removing sensitive information
- Requires minimal annotation compared to existing methods
This research enables organizations to address regulatory requirements for the "right to be forgotten" while preserving model functionality, making AI systems more secure and compliant with privacy regulations.
SAUCE: Selective Concept Unlearning in Vision-Language Models with Sparse Autoencoders