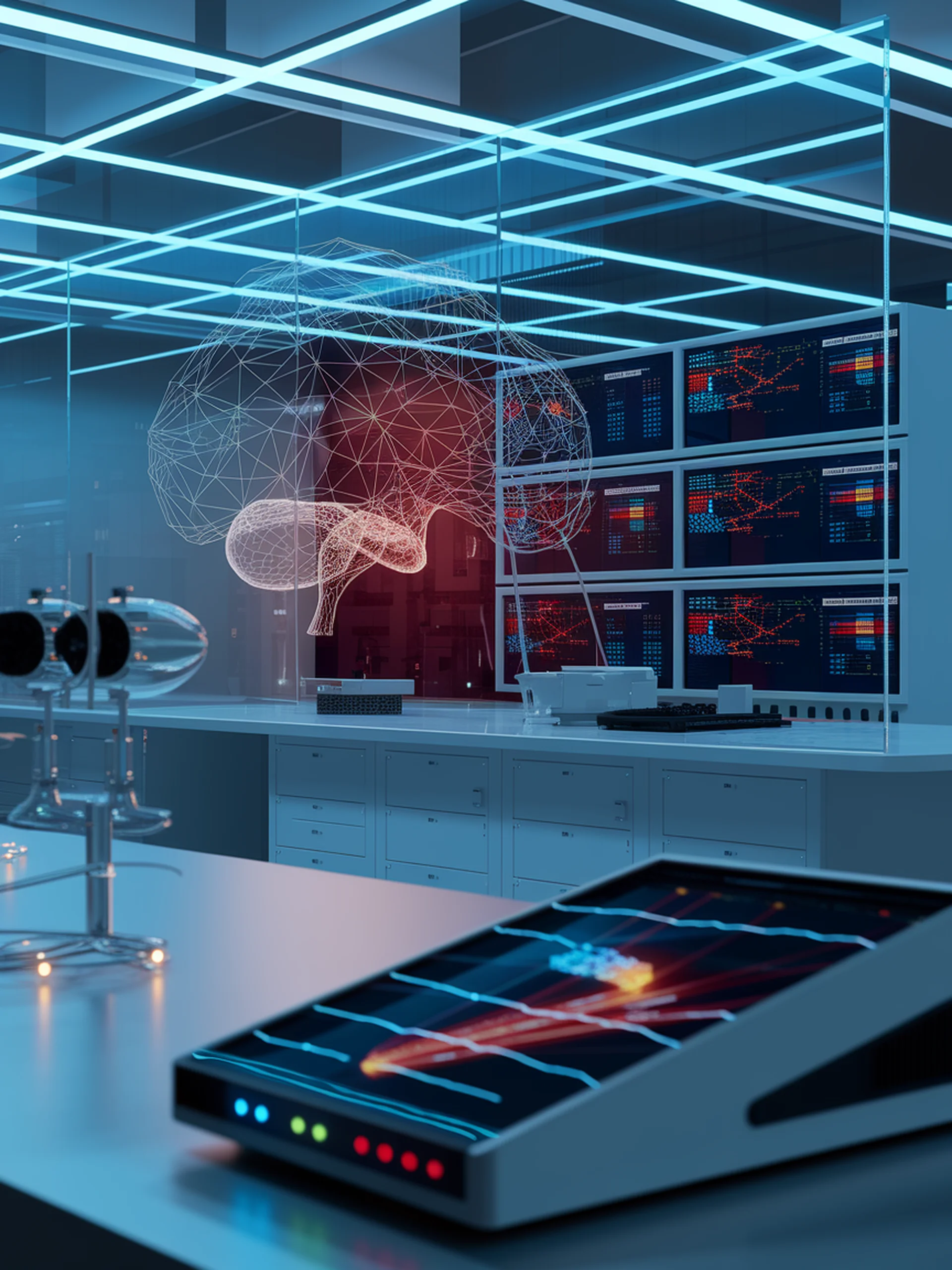
Understanding Unlearning Difficulty in LLMs
A neuro-inspired approach to selective knowledge removal
This research introduces a sample-level unlearning difficulty framework for Large Language Models that enables more precise and interpretable privacy protection.
- Challenges the assumption that all data is equally difficult to unlearn from LLMs
- Proposes a neuro-inspired interpretation to measure unlearning difficulty
- Demonstrates that samples with higher perplexity require more unlearning effort
- Enables more effective privacy protection strategies through selective unlearning
Security Impact: This framework provides organizations with a more nuanced approach to removing sensitive information from deployed AI systems, improving compliance with privacy regulations while maintaining model performance.