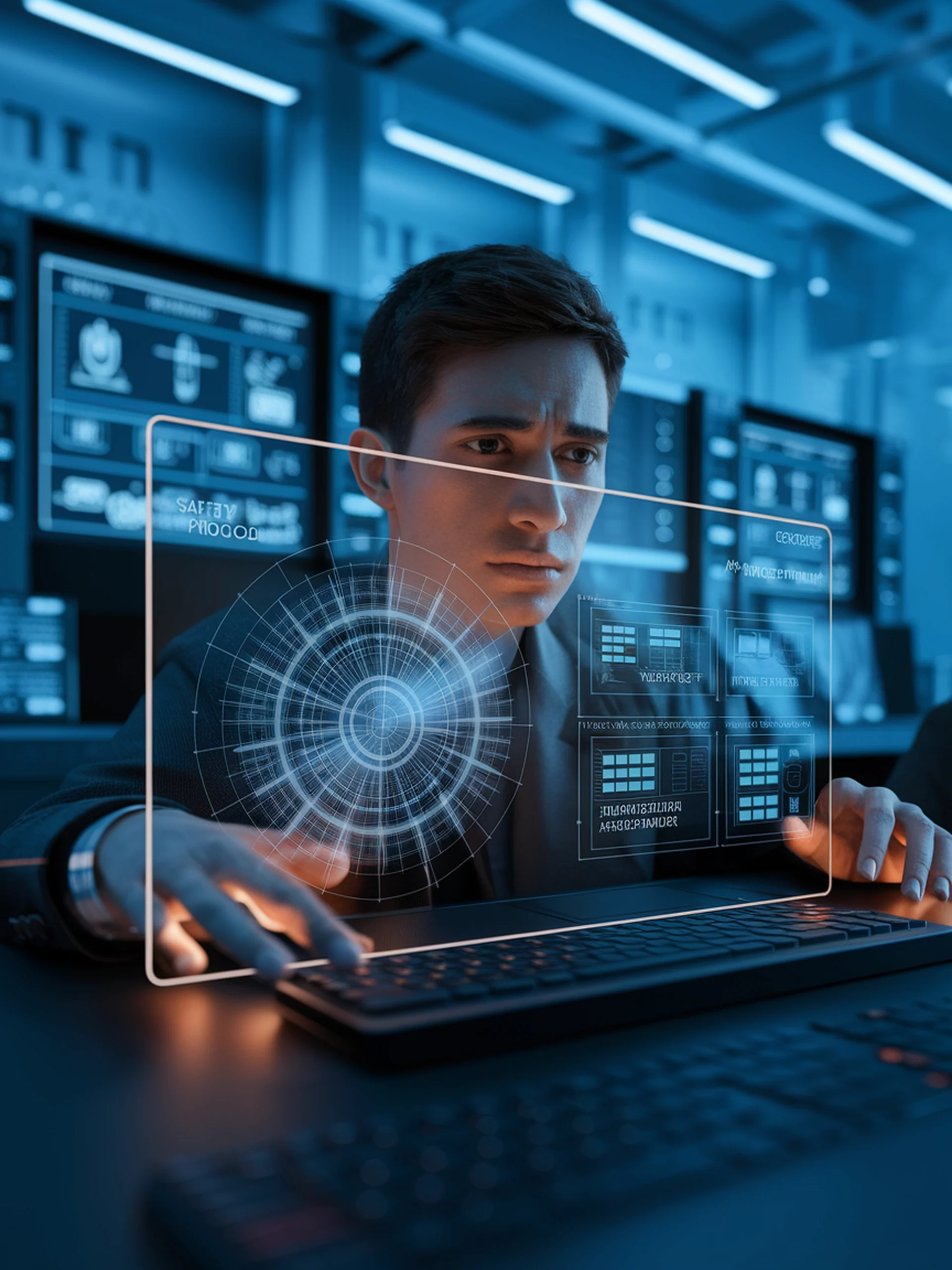
Enhancing Safety in Vision-Language Models
Addressing the Safety Reasoning Gap in VLMs
This research identifies and addresses critical safety reasoning bottlenecks in Vision-Language Models, improving their deployment in high-risk environments.
- Current safety fine-tuning methods fail at complex visual reasoning for unsafe content
- Researchers identified a significant safety reasoning gap in existing approaches
- The paper introduces a specialized Multi-Image Safety dataset to enhance visual safety reasoning
- Implementation reduces attack success rates while maintaining model helpfulness
For security professionals, this work provides crucial advances in protecting AI systems from exploitation through visual inputs, addressing a key vulnerability in modern multimodal AI applications.
Original Paper: Rethinking Bottlenecks in Safety Fine-Tuning of Vision Language Models