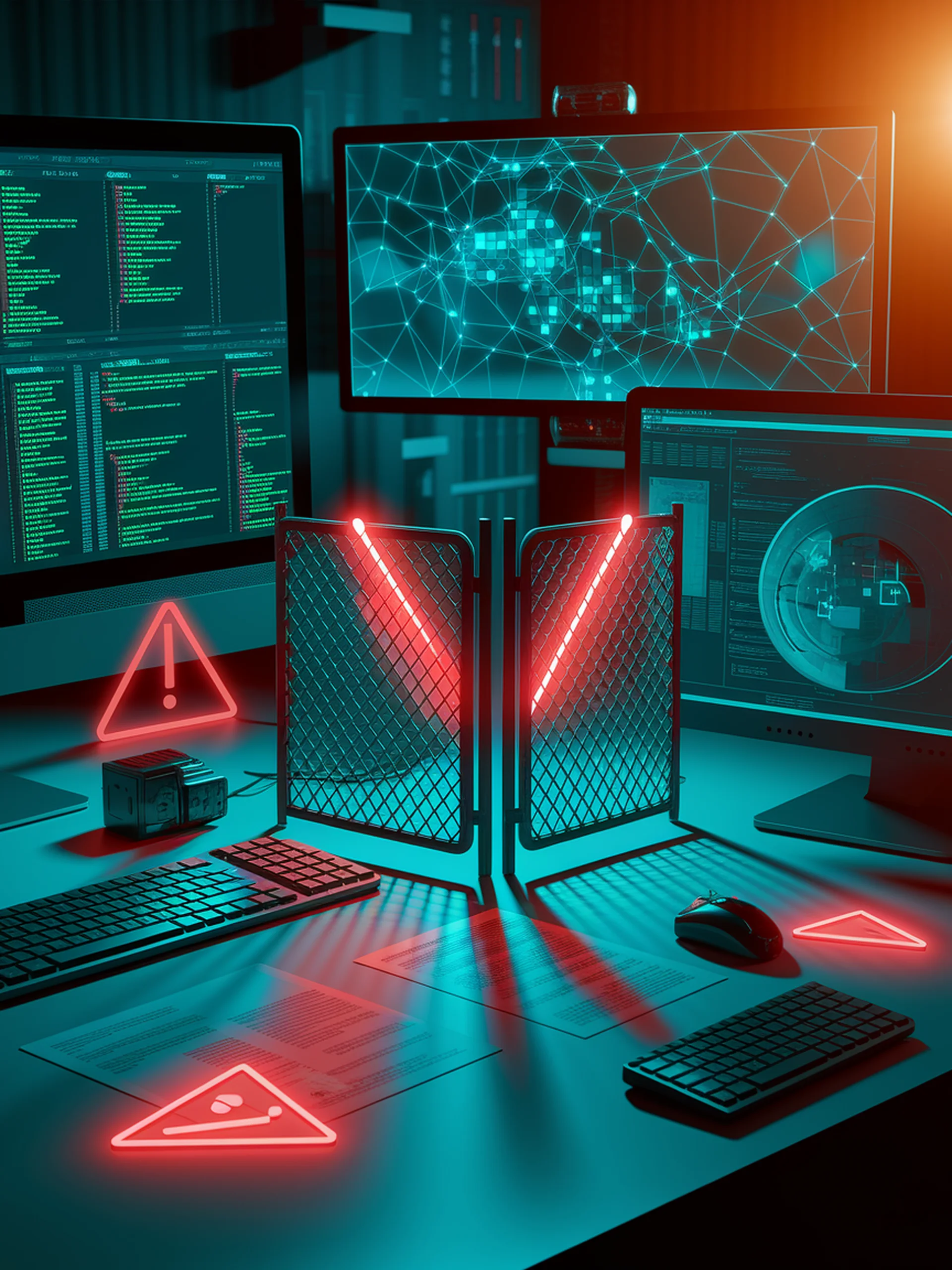
Breaching VLLM Security Guardrails
How sophisticated attacks can bypass multi-layered safety defenses in Vision Large Language Models
This research demonstrates critical security vulnerabilities in Vision Large Language Models by developing a novel MultiFaceted Attack framework that bypasses safety measures in commercial VLLMs.
- Reveals gaps in current multi-layered safety defenses including alignment training and content moderation
- Exposes how sophisticated adversarial techniques can manipulate VLLMs into generating unsafe content
- Highlights urgent security concerns as VLLMs gain wider adoption in real-world applications
- Underscores the need for more robust security measures against evolving attack methods
Security Implications: As VLLMs become more integrated into applications processing visual data, these vulnerabilities could lead to misuse, misinformation, and other harmful outputs if left unaddressed. Organizations deploying VLLMs should evaluate their security posture against these newly identified attack vectors.
Effective Black-Box Multi-Faceted Attacks Breach Vision Large Language Model Guardrails