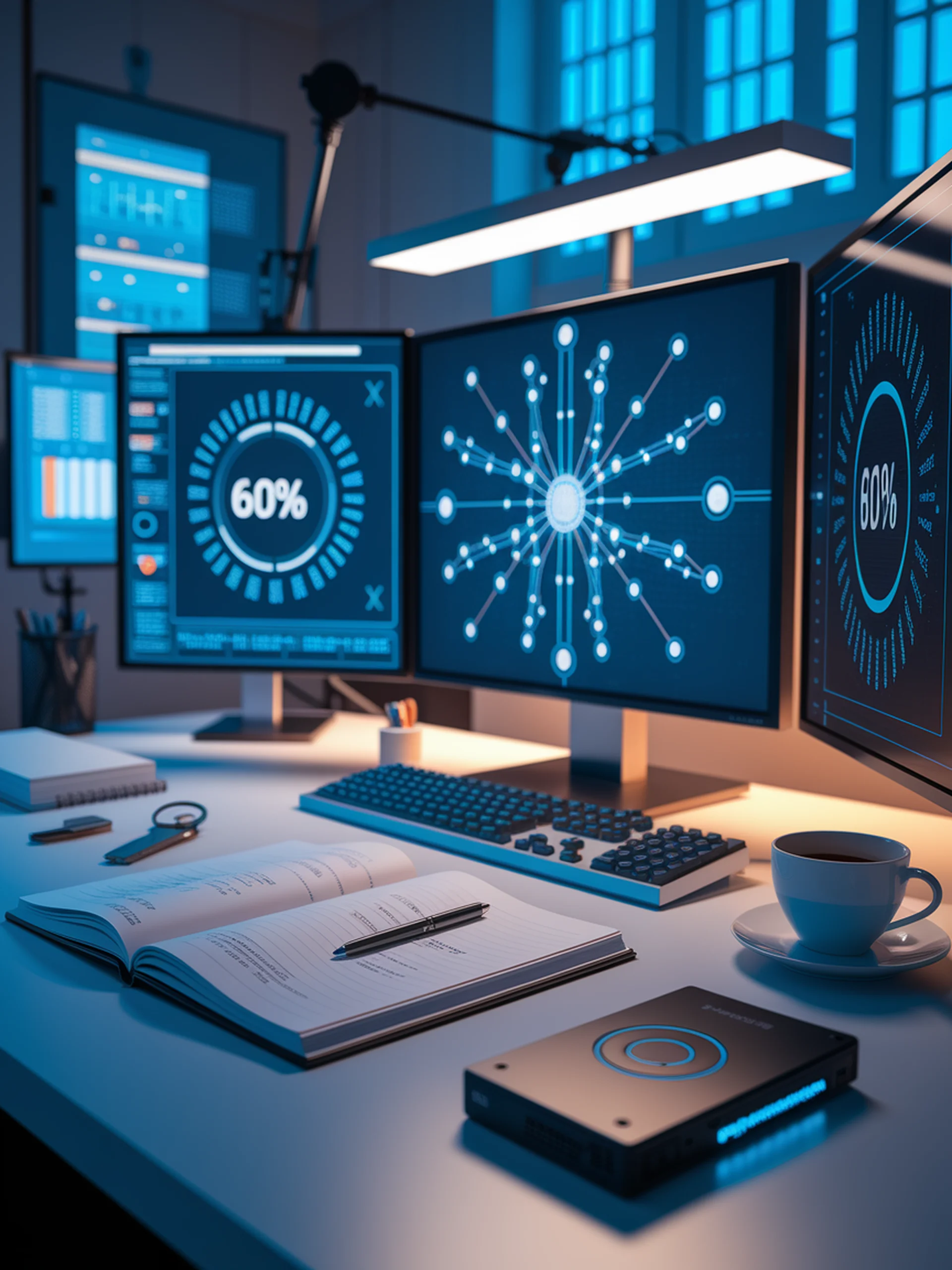
Aligning Multimodal LLMs with Human Preferences
Advancing security and capability through MM-RLHF
This research introduces MM-RLHF, a novel approach for systematically aligning multimodal large language models with human preferences, addressing a critical gap in current MLLM development.
- Achieves a 60% improvement in safety while enhancing overall model capabilities
- Develops a comprehensive multimodal preference dataset for robust alignment
- Demonstrates that human preference alignment can systematically enhance MLLM capabilities
- Establishes new benchmarks for security and safety in multimodal AI systems
Security Impact: By significantly reducing harmful outputs and improving alignment with human values, MM-RLHF represents a crucial advancement for deploying safer AI systems in real-world applications where security concerns are paramount.