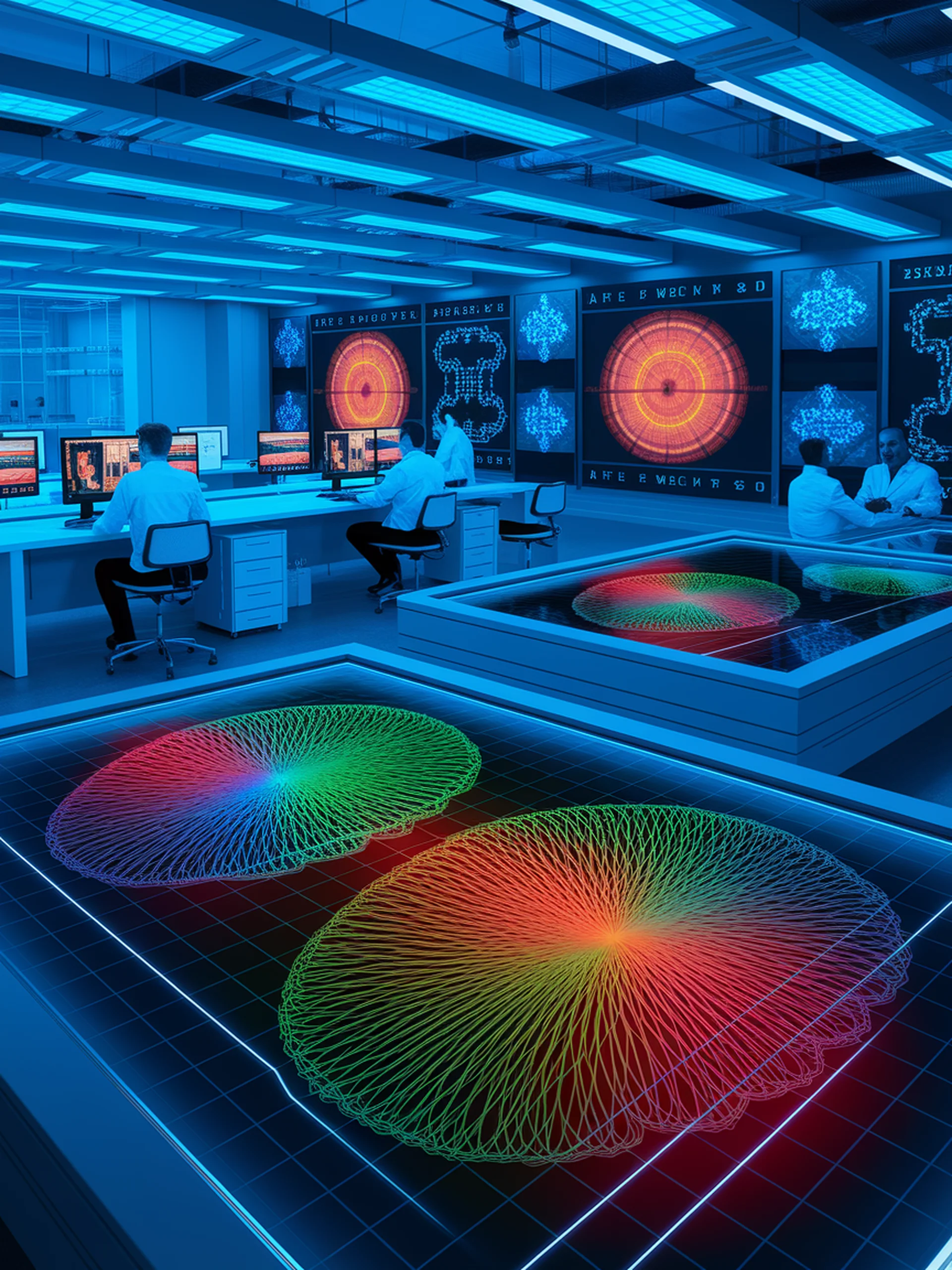
Making Vision-Language Models Safer
Novel approach to identify and neutralize unsafe model weights
This research introduces a new method to enhance safety in vision-language models without compromising performance on safe inputs.
- Developed SafeGround, a comprehensive metric suite to evaluate model safety at different levels
- Introduced Unsafe Weights Manipulation (UWM) to identify and modify parameters processing unsafe content
- Demonstrated better performance preservation on safe inputs compared to existing safety tuning methods
- Achieved improved safety-utility trade-offs across multiple model architectures
This work addresses critical security concerns in AI systems by providing a targeted approach to remove unsafe behaviors while maintaining model performance where it matters most.