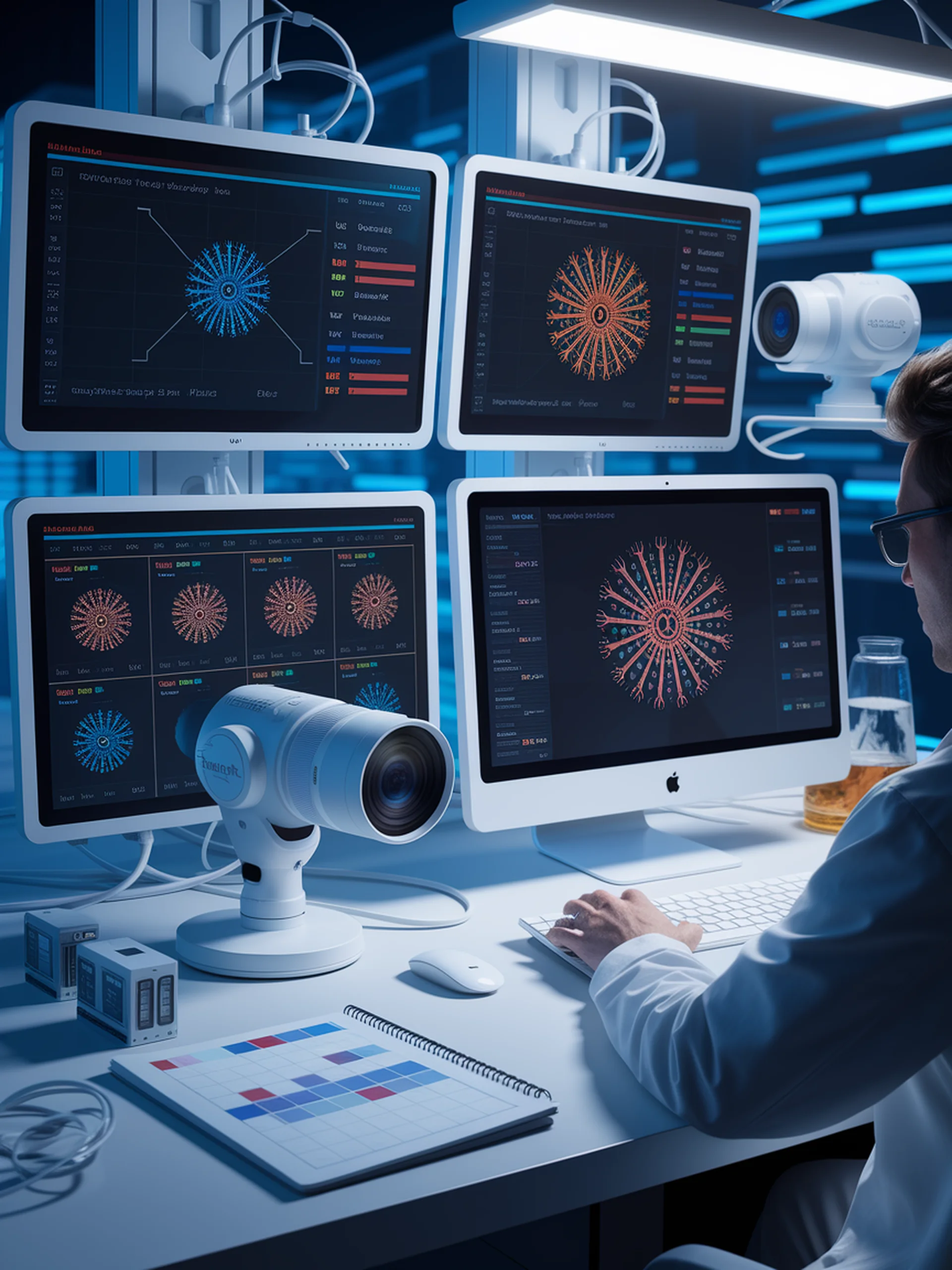
Improving ML Security through Better Error Detection
Novel approach for identifying misclassifications in vision-language models
This research tackles the critical challenge of overconfidence in neural networks by developing efficient methods to detect when vision-language models make incorrect predictions.
- Addresses the problem of neural networks being overconfident even when wrong
- Proposes a few-shot approach that doesn't require extensive retraining
- Enables more reliable deployment in high-security and dynamic environments
- Provides a practical solution for identifying potential vulnerabilities in AI systems
For security teams, this research offers valuable tools to strengthen AI deployments by identifying when models are likely to be wrong, reducing the risk of security incidents caused by undetected misclassifications.