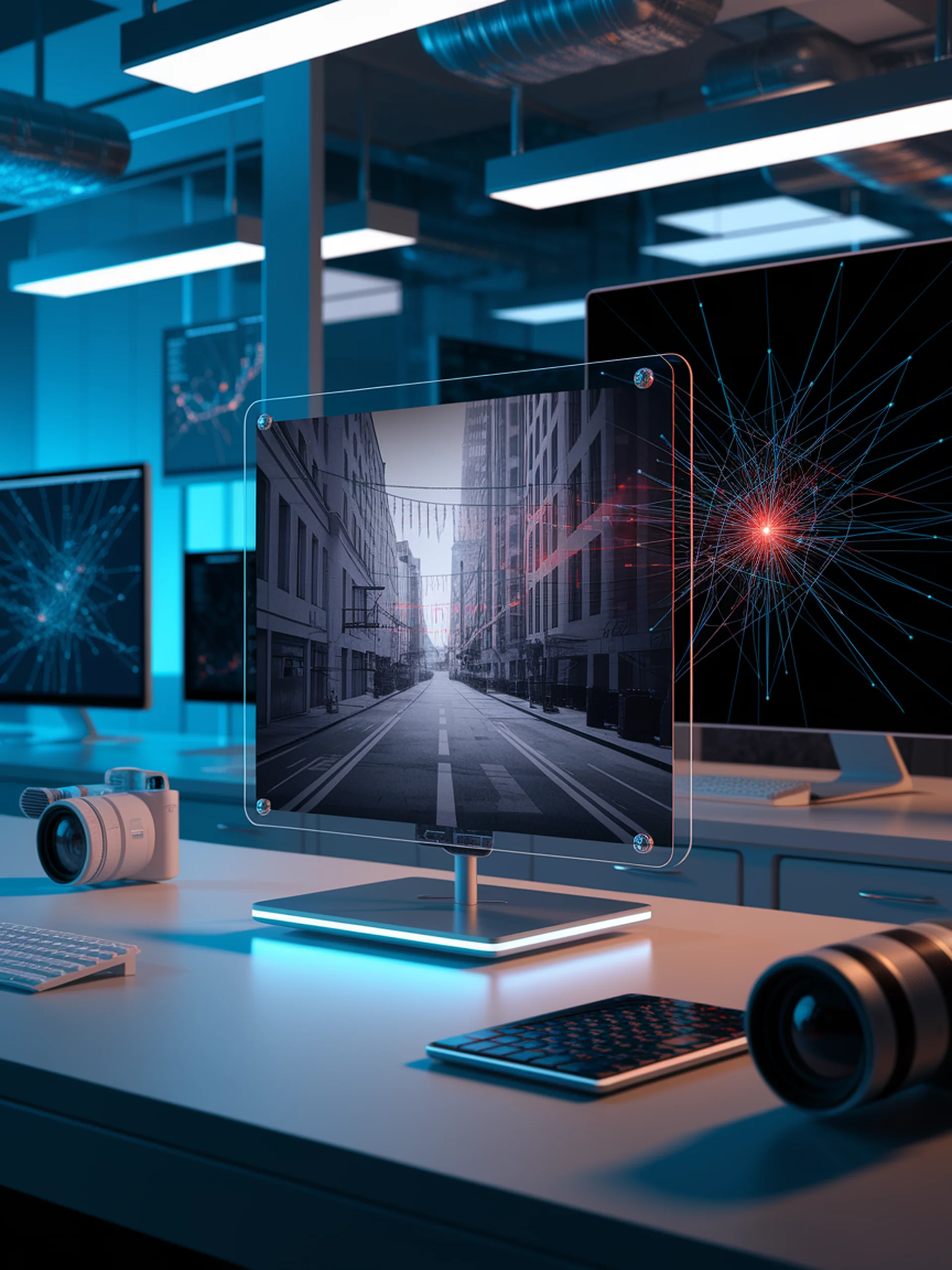
Breaking Vision-Language Models
Query-Agnostic Attacks That Fool LVLMs Regardless of Questions
This research introduces QAVA, a novel attack method that can make large vision-language models (LVLMs) consistently generate incorrect responses to any question about a manipulated image.
- Creates universal adversarial images that trigger incorrect answers regardless of what questions are asked
- Demonstrates vulnerabilities in major LVLMs including GPT-4V, Claude, and Gemini
- Achieves up to 95% attack success rate while maintaining visual imperceptibility
- Proposes defense mechanisms through adversarial training
This research highlights critical security flaws in multimodal AI systems, revealing how seemingly innocuous images can systematically compromise vision-language models across diverse applications—essential knowledge for developing robust AI safety measures.
QAVA: Query-Agnostic Visual Attack to Large Vision-Language Models