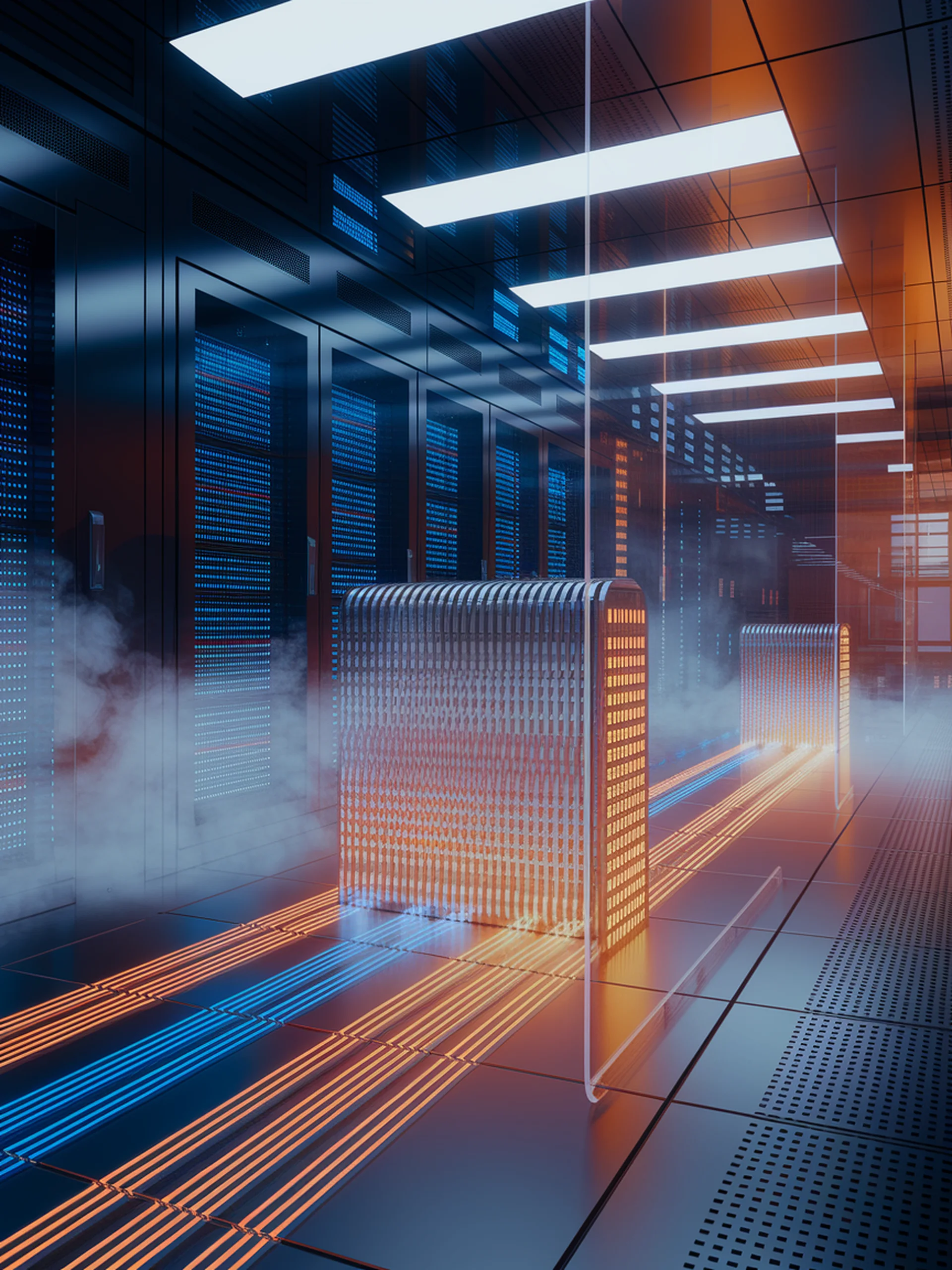
Private LLM Fine-tuning Breakthrough
Securing AI Training with Zeroth-order Optimization
DP-ZO introduces a novel privacy-preserving framework for fine-tuning large language models using zeroth-order optimization, achieving better privacy-utility tradeoffs than traditional methods.
- Leverages randomized gradient directions with only scalar loss values influencing the training process
- Successfully balances privacy guarantees with maintained model utility
- Provides a scalable alternative to DP-SGD for foundation model fine-tuning
- Demonstrates practical implementation with formal differential privacy guarantees
This research addresses critical security concerns for organizations deploying custom LLMs, enabling compliance with privacy regulations while maintaining model performance. The approach represents a significant advancement in protecting sensitive training data from extraction and inference attacks.
Private Fine-tuning of Large Language Models with Zeroth-order Optimization