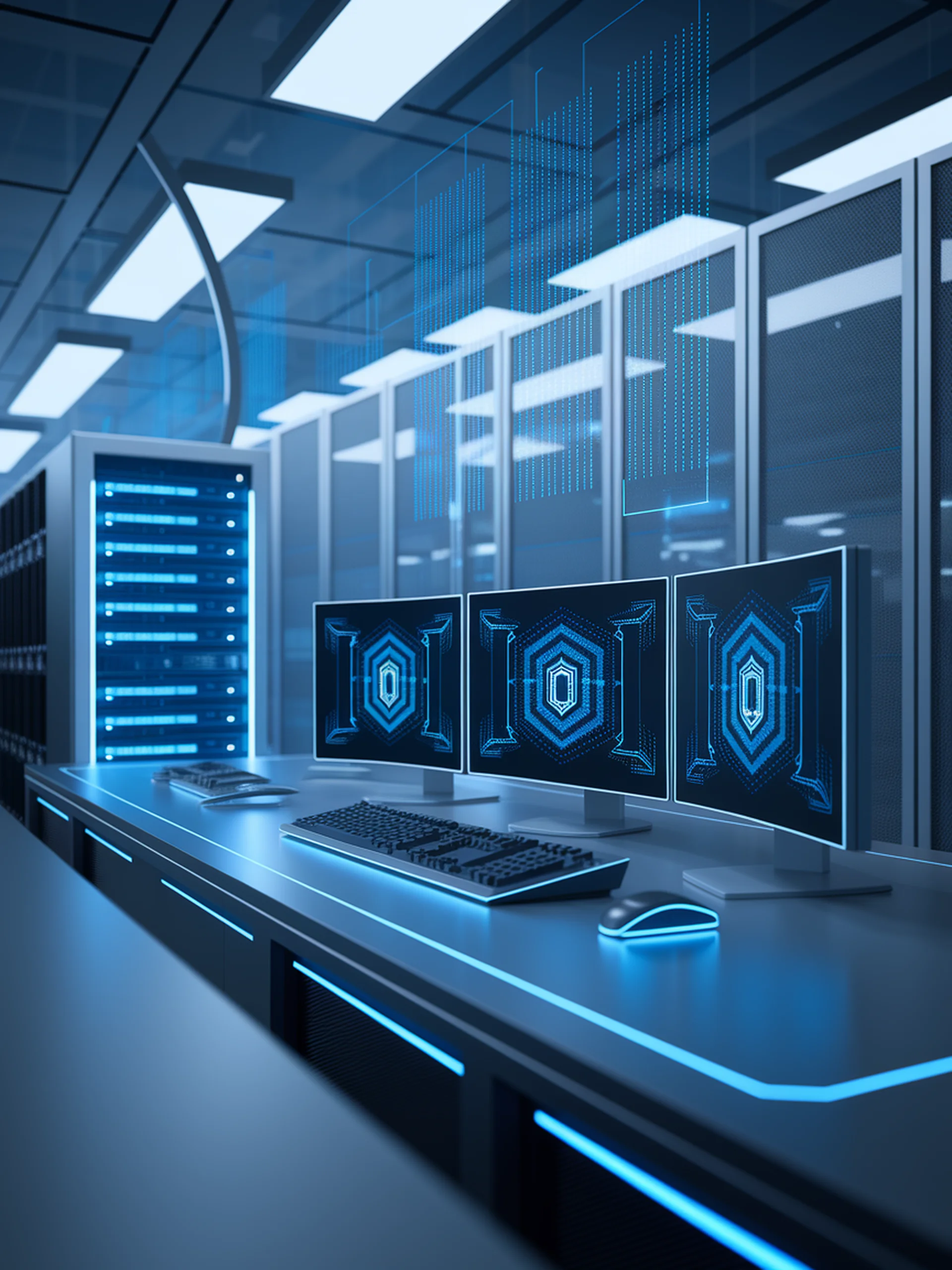
Privacy-Preserving LLM Fine-Tuning
A Zeroth-Order Approach for Balancing Privacy, Utility, and Performance
This research introduces DP-ZOSO, a novel differentially private method for fine-tuning large language models that protects sensitive training data while maintaining model performance.
- Addresses the critical privacy-utility-scalability tradeoff in LLM fine-tuning
- Implements zeroth-order optimization techniques that reduce computational overhead
- Provides mathematical guarantees for differential privacy protection
- Demonstrates scalable performance on large language models
As LLMs become increasingly integrated into critical business applications, this research offers security teams a practical approach to protect sensitive data during fine-tuning while preserving model utility—essential for regulatory compliance and protecting proprietary information.
Differentially Private Zeroth-Order Methods for Scalable Large Language Model Finetuning