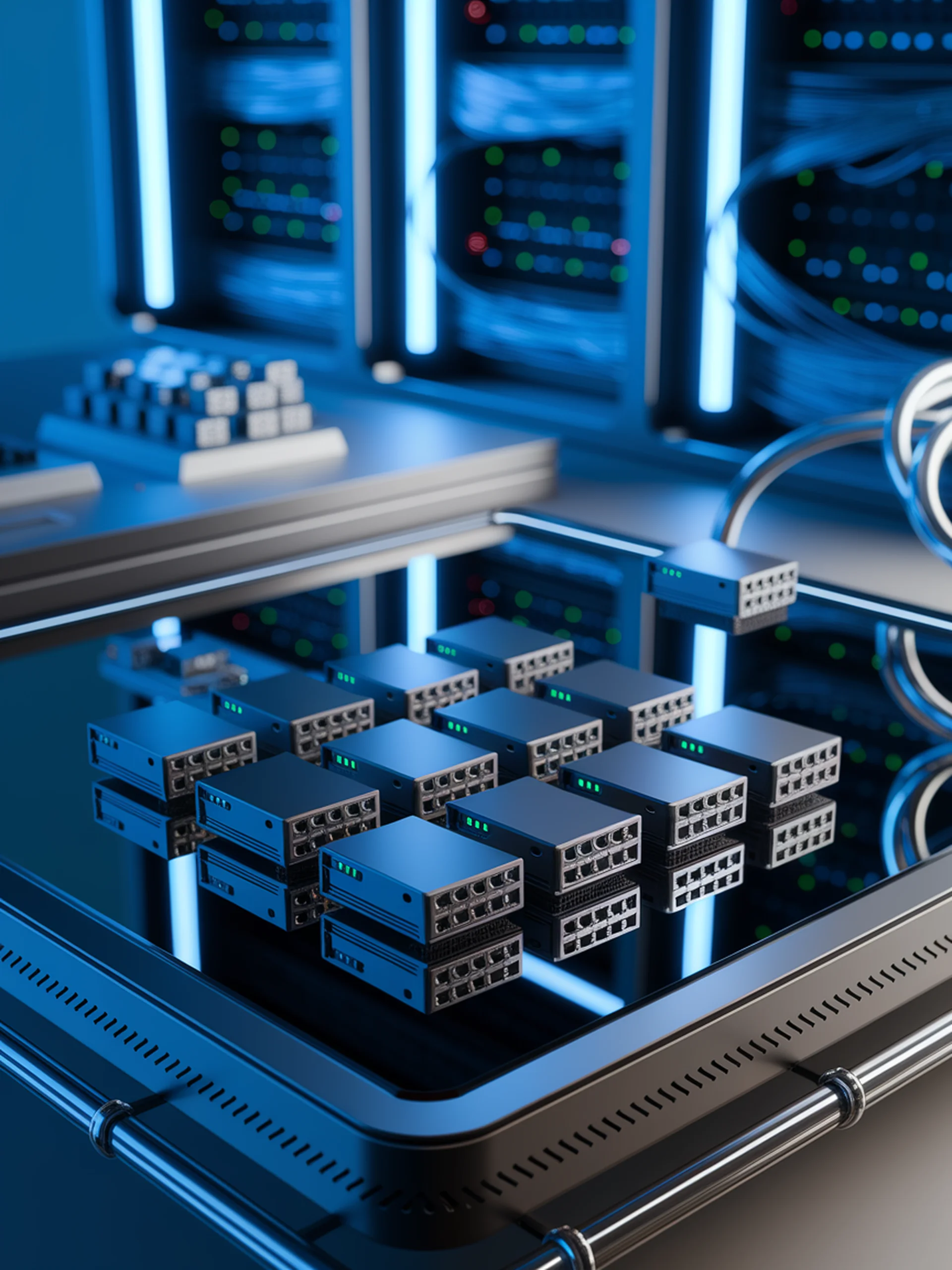
Controlled Access & Data Removal for LLMs
AdapterSwap: A framework for managing evolving data requirements in LLMs
AdapterSwap introduces a novel approach to continuously train language models with strong guarantees for data access control and removal.
- Selective Knowledge Access: Enables user-based access controls to specific knowledge subsets
- Guaranteed Forgetting: Ensures complete removal of information when required
- Modular Architecture: Uses lightweight adapters instead of full model retraining
- Practical Implementation: Achieves these goals with minimal performance impact
For security teams, AdapterSwap offers a critical solution to data governance challenges in LLM deployments, addressing privacy regulations and access management requirements while maintaining model utility.
AdapterSwap: Continuous Training of LLMs with Data Removal and Access-Control Guarantees