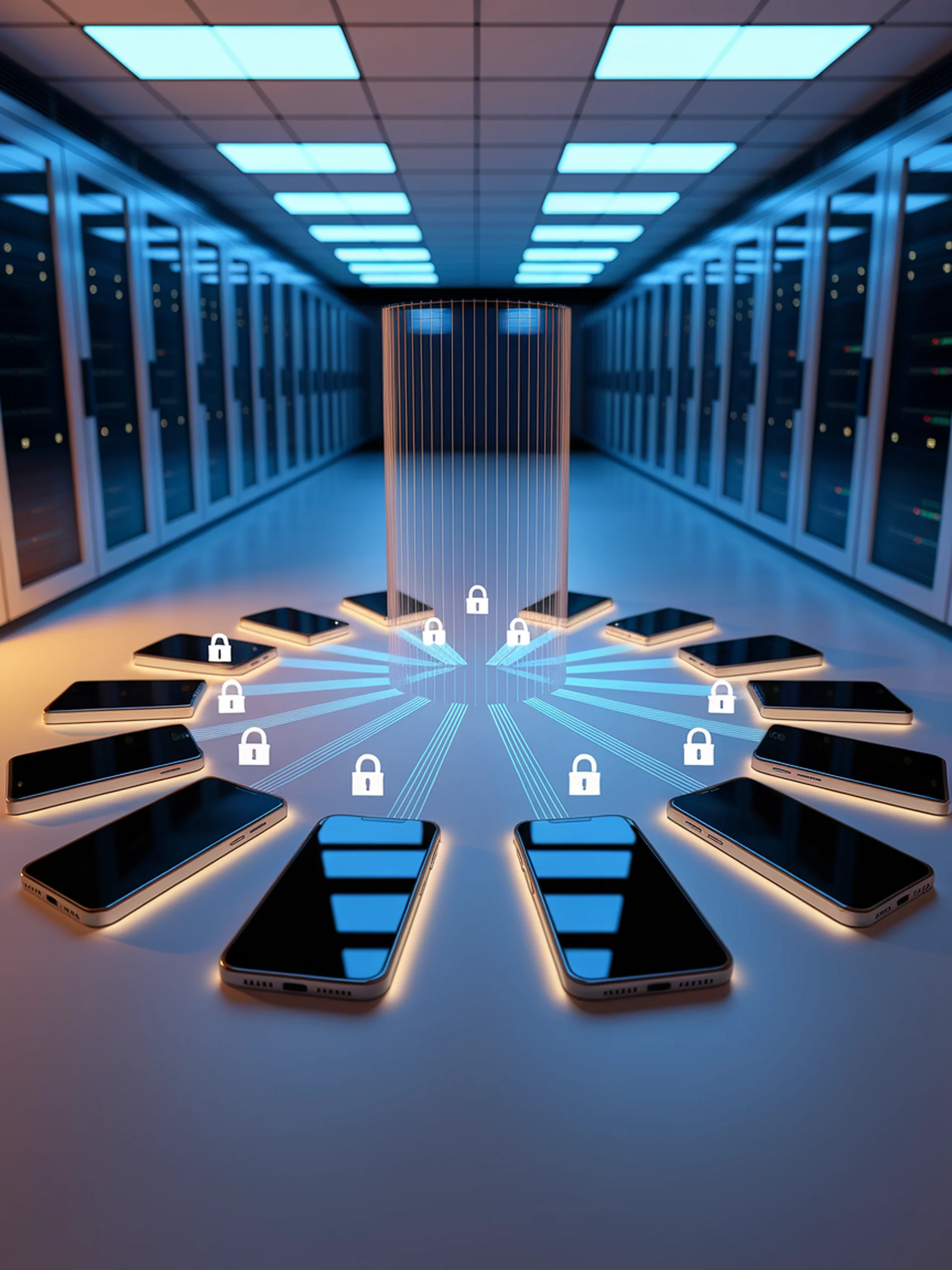
Privacy-Preserving AI Alignment
Federated Learning for RLHF Without Sharing Personal Data
This research introduces federated RLHF architectures that enable large language models to learn from human preferences while protecting user privacy.
- Developed new methods (FedBis and FedBiscuit) that keep sensitive preference data on users' devices
- Demonstrated effective AI alignment without centralized data collection
- Achieved comparable performance to traditional RLHF while enhancing privacy protection
- Enables organizations to improve AI systems ethically while respecting data sovereignty
This breakthrough matters for Security by solving the privacy-utility tradeoff in AI alignment, allowing organizations to fine-tune LLMs with real user preferences without exposing sensitive information.
Towards Federated RLHF with Aggregated Client Preference for LLMs